Product Ranking in the Presence of Social Learning.
Oper. Res.(2023)
摘要
This paper studies product ranking mechanisms of a monopolistic online platform in the presence of social learning. The products' quality is initially unknown, but consumers can sequentially learn it as online reviews accumulate. A salient aspect of our problem is that consumers, who want to purchase a product from a list of items displayed by the platform, incur a search cost while scrolling down the list. In this setting, the social learning dynamics, and hence the demand, is affected by the interplay of two unique features: substitution and ranking effects. The platform can influence the social learning dynamics by adjusting the ranking of the products to ultimately maximize the revenue collected from commission fees for sold items. To formulate the problem in a tractable form, we use a large-market (fluid) approximation and show that consumers eventually learn the products' quality and characterize the speed of learning. Armed with this backing, we formulate the platform's ranking problem in the fluid setting, where we assume the perspective of an uninformed platform that does not know the true quality vector but rather learns it through consumers' review process. We compare different ranking policies based on the worst-case regret with respect to a fully informed platform benchmark. Our analysis yields three main insights. First, a greedy policy that maximizes immediate revenue by displaying products based on current ratings may incur highly suboptimal worst-case regret, as it may relegate the most profitable products to the lowest positions in the ranking if their current rating is not high enough. Second, a simple variant of the greedy policy can suffi- ciently alleviate the regret by balancing the trade-off between exploration and exploitation. Third, we characterize the critical level of search cost for which the regret does not grow exponentially with the number of products.
更多查看译文
关键词
Revenue Management and Market Analytics,social learning,information aggregation,online reviews,online platforms
AI 理解论文
溯源树
样例
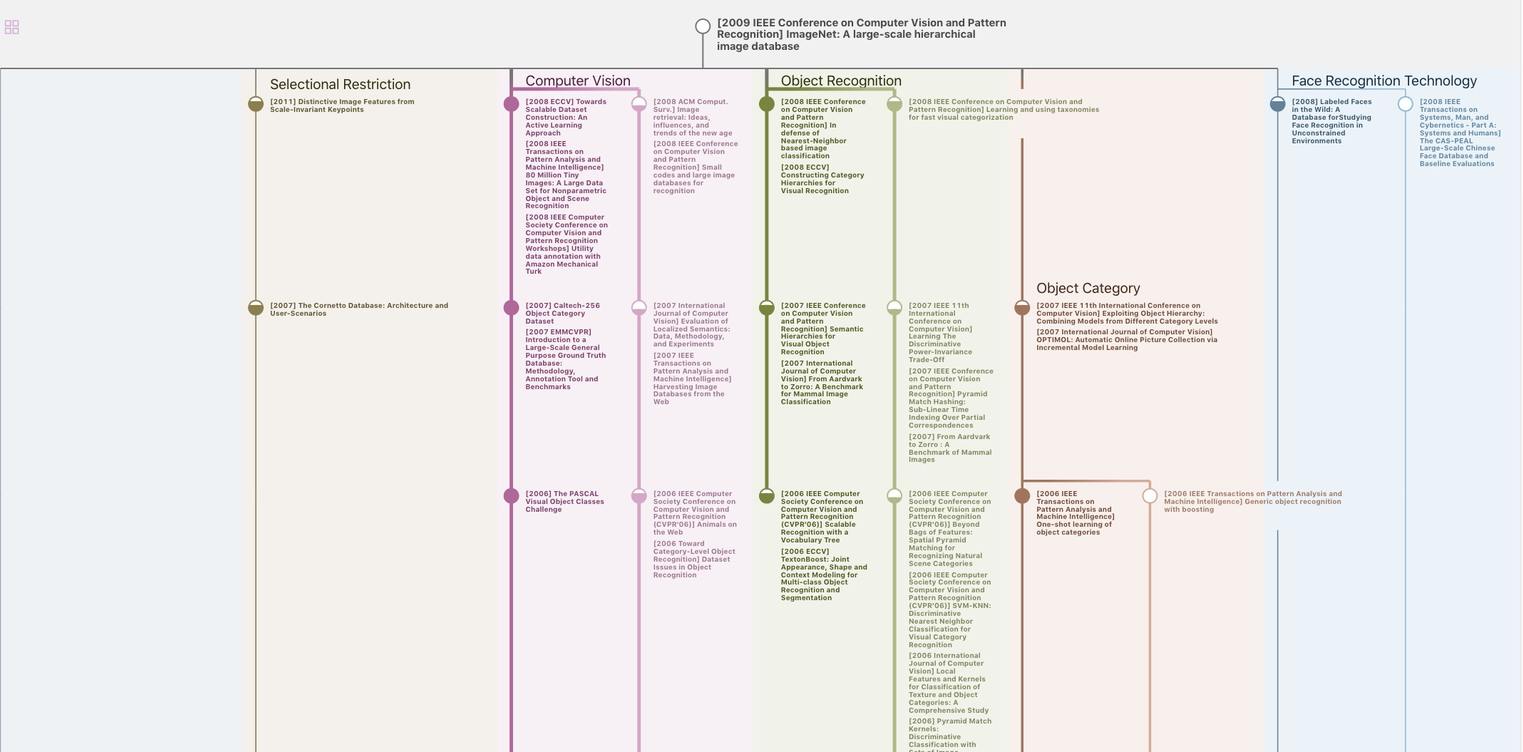
生成溯源树,研究论文发展脉络
Chat Paper
正在生成论文摘要