ATT-YOLOv5-Ghost: water surface object detection in complex scenes
J. Real Time Image Process.(2023)
摘要
In recent years, Unmanned Surface Vehicles (USVs) have been widely used in water surface monitoring and management. The main problems of the USVs-based water surface object detection method are that the features will be lost when downsampling complex water surface environment images, resulting in low detection accuracy. Moreover, the number of parameters and calculation amount of these models are too much, which will seriously affect the speed of training and detection. Therefore, this paper proposed the ATT-YOLOv5-Ghost algorithm. First, we added an Efficient Channel Attention (ECA) module to each CSP1 unit of the backbone CSPDarknet, which solves the problem of accuracy drop caused by multi-scale feature loss during downsampling. Second, we proposed a method combining ECA and Ghost modules. In the process of feature fusion, the problems such as the increase of parameters, slow detection speed and repeated gradient calculation caused by too complex algorithm were solved. The ATT-YOLOv5-Ghost algorithm improves the detection accuracy by 4.6% compared with the baseline. The FPS can reach 64.9, and the computational amount is reduced by 8.9%. The algorithm complexity was significantly reduced.
更多查看译文
关键词
water surface object detection,complex scenes,att-yolov
AI 理解论文
溯源树
样例
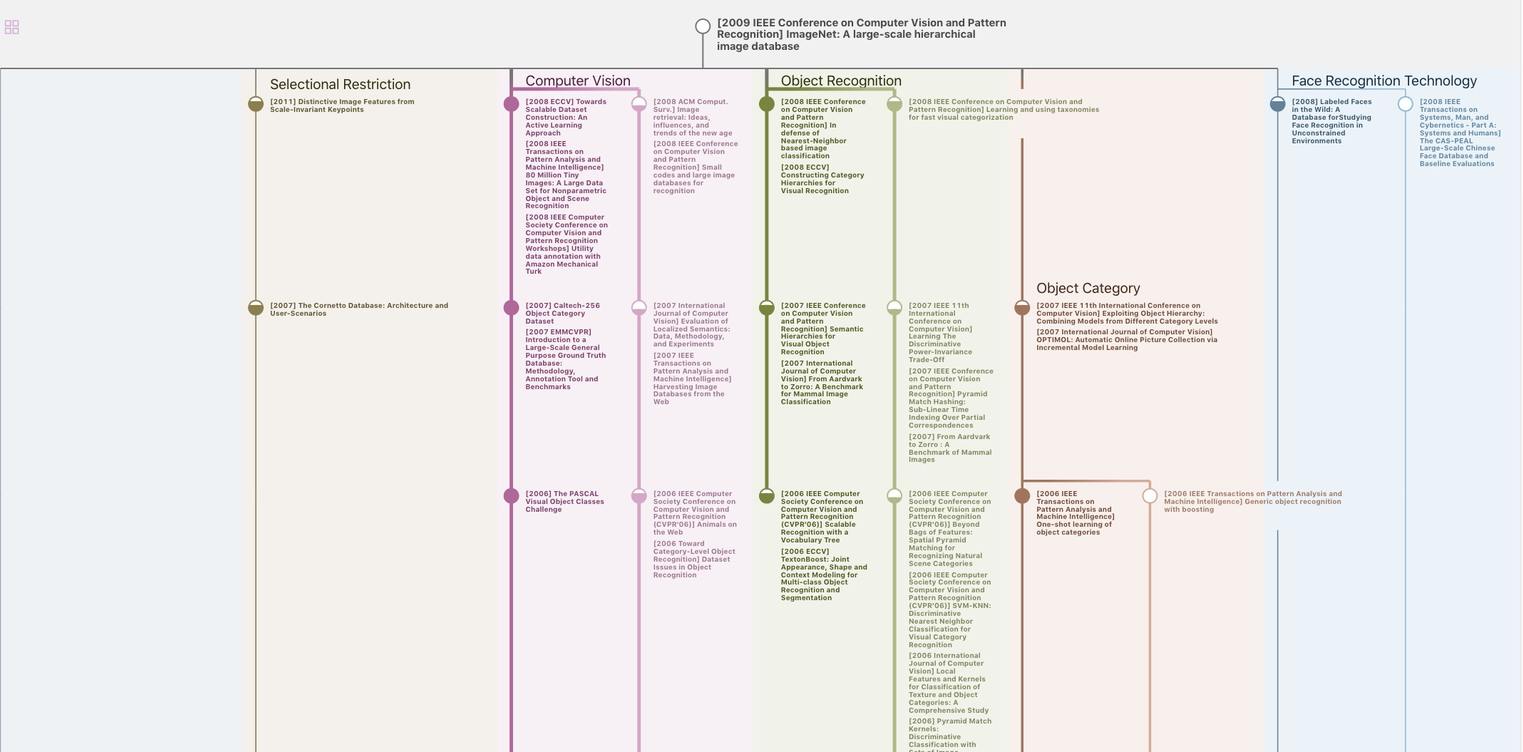
生成溯源树,研究论文发展脉络
Chat Paper
正在生成论文摘要