Granularity self-information based uncertainty measure for feature selection and robust classification.
Fuzzy Sets Syst.(2023)
摘要
Information entropy theory has been widely studied and successfully applied to machine learning and data mining. The fuzzy entropy and neighborhood entropy theories have been rapidly developed and widely used in uncertainty measure. In this paper, a granularity self-information theory is first proposed to measure uncertainty robustly. The theory improves the shortcomings of neighborhood self-information in measuring sample uncertainty by combining with data distributions. Then, granularity entropy theory is put forward and fully explained. With the proposed theories, a novel feature selection algorithm and a robust classification algorithm are designed and validated with some experiments. The experimental results show the designed algorithms have good performance. This indicates the efficacy of granularity self-information and granularity entropy for evaluating samples and features. & COPY; 2023 Elsevier B.V. All rights reserved.
更多查看译文
关键词
Uncertainty measure,Granularity self-information,Neighborhood entropy,Feature selection,Robust classification
AI 理解论文
溯源树
样例
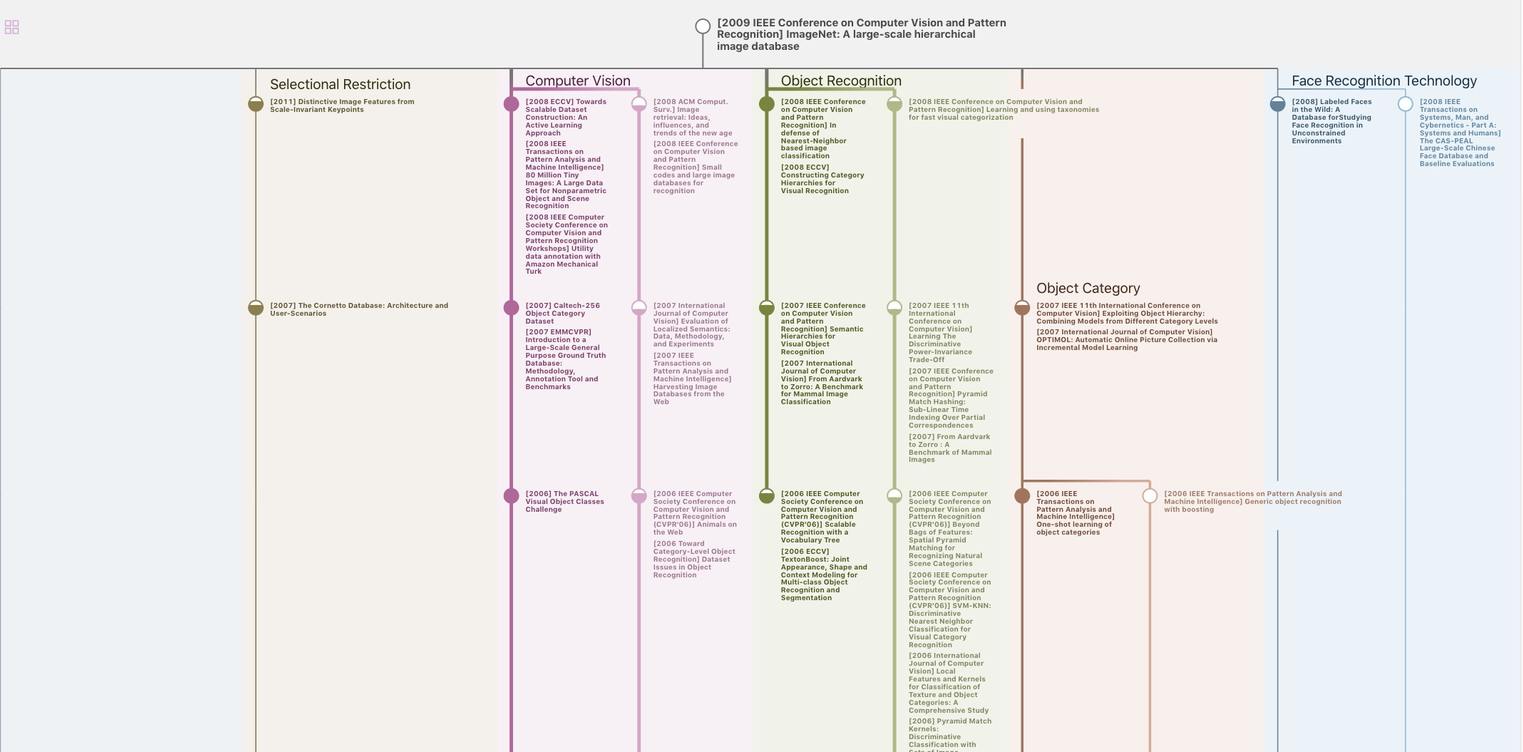
生成溯源树,研究论文发展脉络
Chat Paper
正在生成论文摘要