Reward poisoning attacks in deep reinforcement learning based on exploration strategies
NEUROCOMPUTING(2023)
Abstract
In recent years, deep reinforcement learning (DRL) has been widely applied in various fields, and its vulnerability to adversarial samples, which is inherited from deep learning, has also received considerable attention for training robust DRL agents. However, most of existing attack approaches aim to perturb the observations of DRL agents, and poisoning attacks against DRL through perturbing the reward from the environment have been studied less. In this paper, we propose a novel reward poisoning adversarial attack method that employs the exploration strategy of reinforcement learning to help effectively attack a target. The adversary first builds a deep neural network to attain the exploration value of each state-action pair, i.e., the E-value, which is utilized to determine the appropriate time to attack the target and the sign of adversarial perturbation to generate adversary samples to the reward. The experimental results demonstrate that the reward poisoning attack algorithm based on E-values proposed in this paper enables an adversary to improve the efficiency of attacks with fewer adversarial samples.
MoreTranslated text
Key words
Deep reinforcement learning,Poisoning attacks,Exploration strategies,Reward-based attacks
AI Read Science
Must-Reading Tree
Example
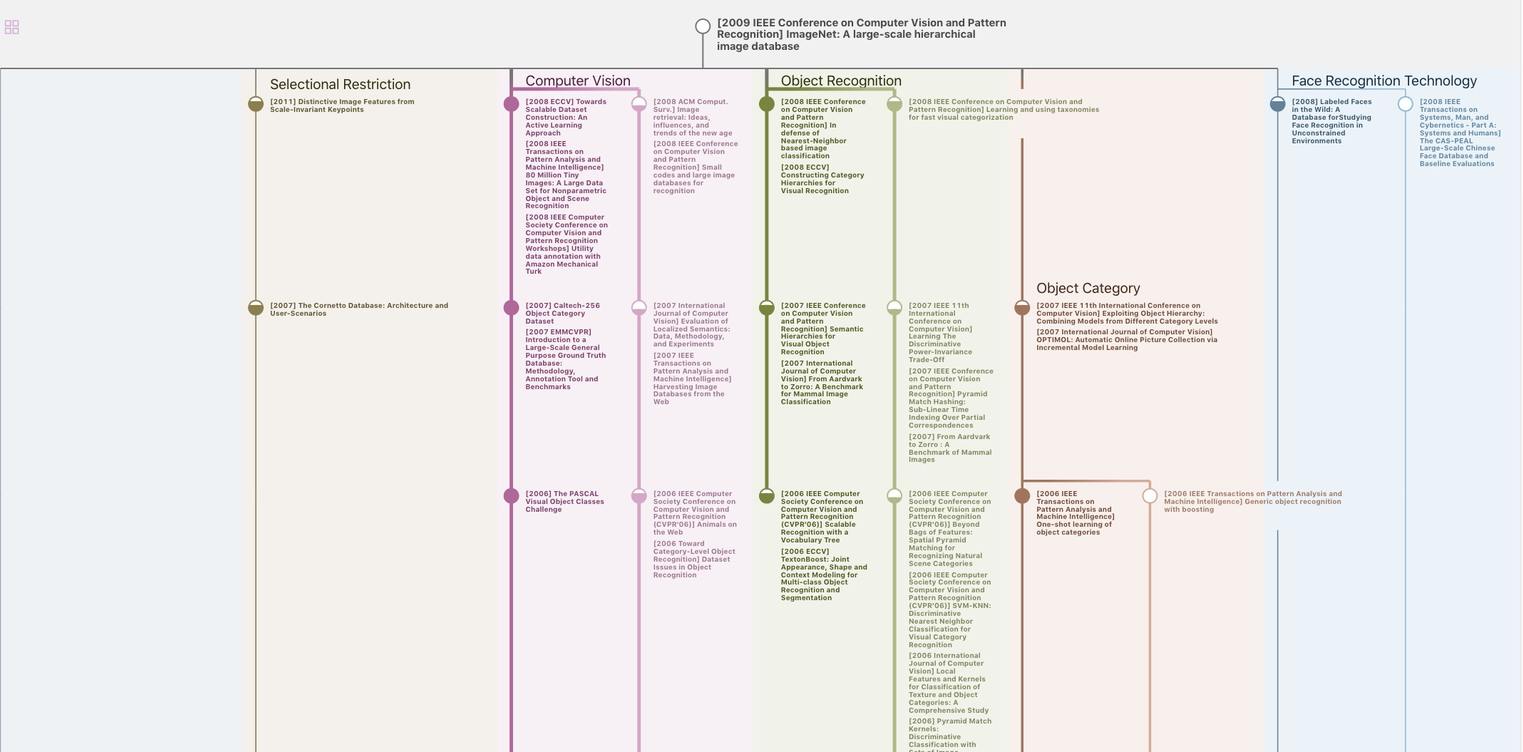
Generate MRT to find the research sequence of this paper
Chat Paper
Summary is being generated by the instructions you defined