Aerial Insights: Deep Learning-Based Human Action Recognition in Drone Imagery
IEEE Access(2023)
摘要
Human action recognition is critical because it allows machines to comprehend and interpret human behavior, which has several real-world applications such as video surveillance, robot-human collaboration, sports analysis, and entertainment. The enormous variety in human motion and appearance is one of the most challenging problems in human action recognition. Additionally, when drones are employed for video capture, the complexity of recognition gets enhanced manyfold. The challenges including the dynamic background, motion blur, occlusions, video capture angle, and exposure issues gets introduced that need to be taken care of. In this article, we proposed a system that deal with the mentioned challenges in drone recorded red-green-blue (RGB) videos. The system first splits the video into its constituent frames and then performs a focused smoothing operation on the frames utilizing a bilateral filter. As a result, the foreground objects in the image gets enhanced while the background gets blur. After that, a segmentation operation is performed using a quick shift segmentation algorithm that separates out human silhouette from the original video frame. The human skeleton was extracted from the silhouette, and key-points on the skeleton were identified. Thirteen skeleton key-points were extracted, including the head, left wrist, right wrist, left elbow, right elbow, torso, abdomen, right thigh, left thigh, right knee, left knee, right ankle, and left ankle. Using these key-points, we extracted normalized positions, their angular and distance relationship with each other, and 3D point clouds. By implementing an expectation maximization algorithm based on the Gaussian mixture model, we drew elliptical clusters over the pixels using the key-points as the central positions to represent the human silhouette. Landmarks were located on the boundaries of these ellipses and were tracked from the beginning until the end of activity. After optimizing the feature matrix using a naive Bayes feature optimizer, the classification is performed using a deep convolutional neural network. For our experimentation and the validation of our system, three benchmark datasets were utilized i.e., the UAVGesture, the DroneAction, and the UAVHuman dataset. Our model achieved a respective action recognition accuracy of 0.95, 0.90, and 0.44 on the mentioned datasets.
更多查看译文
关键词
Convolutional neural network,expectation maximization,quadratic discriminant analysis,quick-shift segmentation,video processing
AI 理解论文
溯源树
样例
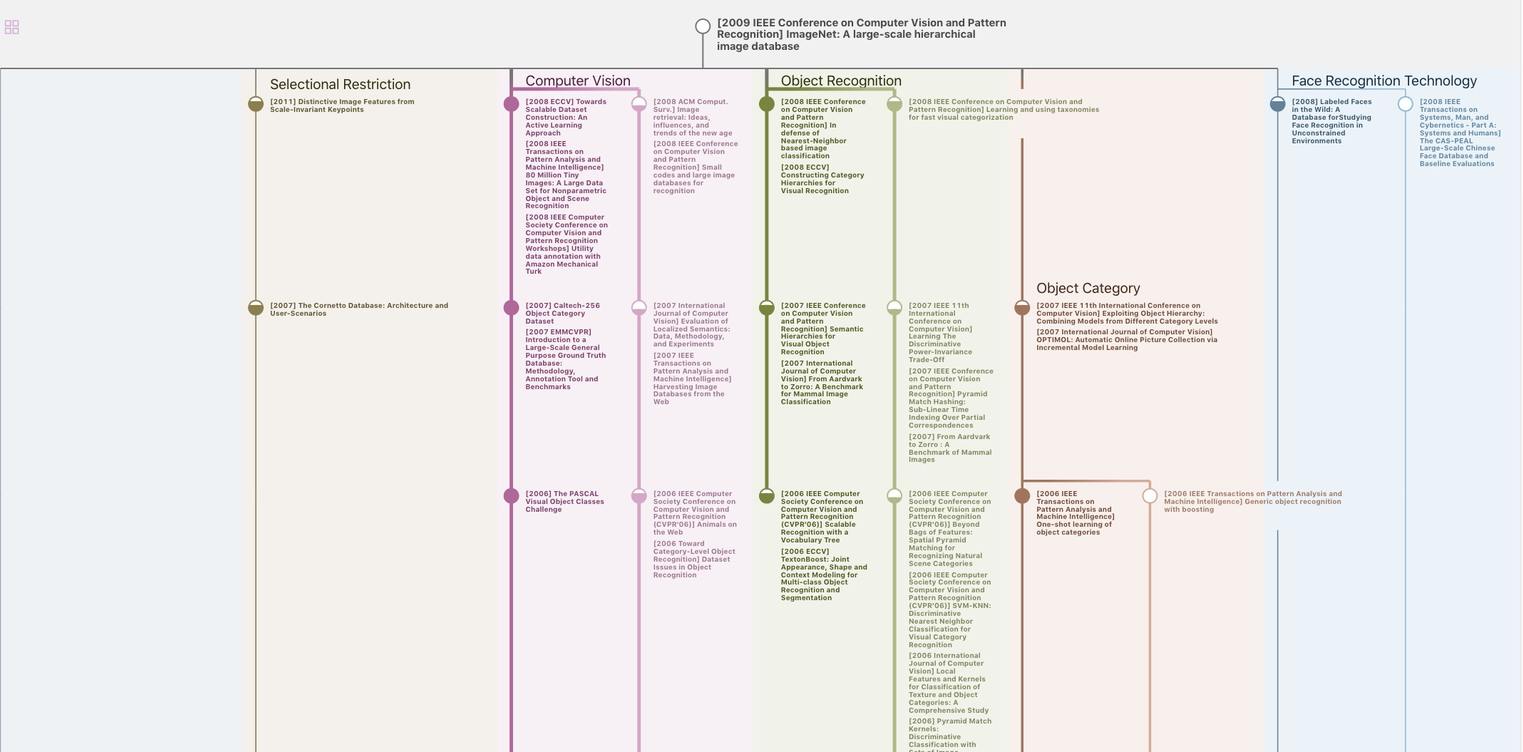
生成溯源树,研究论文发展脉络
Chat Paper
正在生成论文摘要