Credit Card Fraud Detection Based on Improved Variational Autoencoder Generative Adversarial Network
IEEE Access(2023)
摘要
The rapid spread of mobile banking and e-commerce has coincided with a dramatic increase in fraudulent online payments in recent years. Although machine learning and deep learning are widely used in credit card fraud detection, the typical credit card transaction data set is unbalanced, and the fraud data is much less than the normal transaction data, limiting the effectiveness of traditional binary classification algorithms. To overcome this issue, researchers oversample minority class data and utilize ensemble learning classification algorithms. However, oversampling still has disadvantages. Hence, we improve the generator part of the Variational Autoencoder Generative Adversarial Network (VAEGAN) and propose a new oversampling method that generates convincing and diverse minority class data. The training set is enhanced by generating minority class fraud data to train the ensemble learning classification model. The method is tested on an open credit card dataset, with the experimental results demonstrating that the oversampling method utilizing the improved VAEGAN is superior to the oversampling method of Generative Adversarial Network (GAN), Variational Autoencoder (VAE), and Synthetic Minority Oversampling Technique (SMOTE) in terms of Precision, F1_score, and other indicators. The oversampling method based on the improved VAEGAN effectively deals with the classification problem of imbalanced data.
更多查看译文
关键词
Credit card fraud,ensemble learning,variational autoencoder generative adversarial network,oversampling
AI 理解论文
溯源树
样例
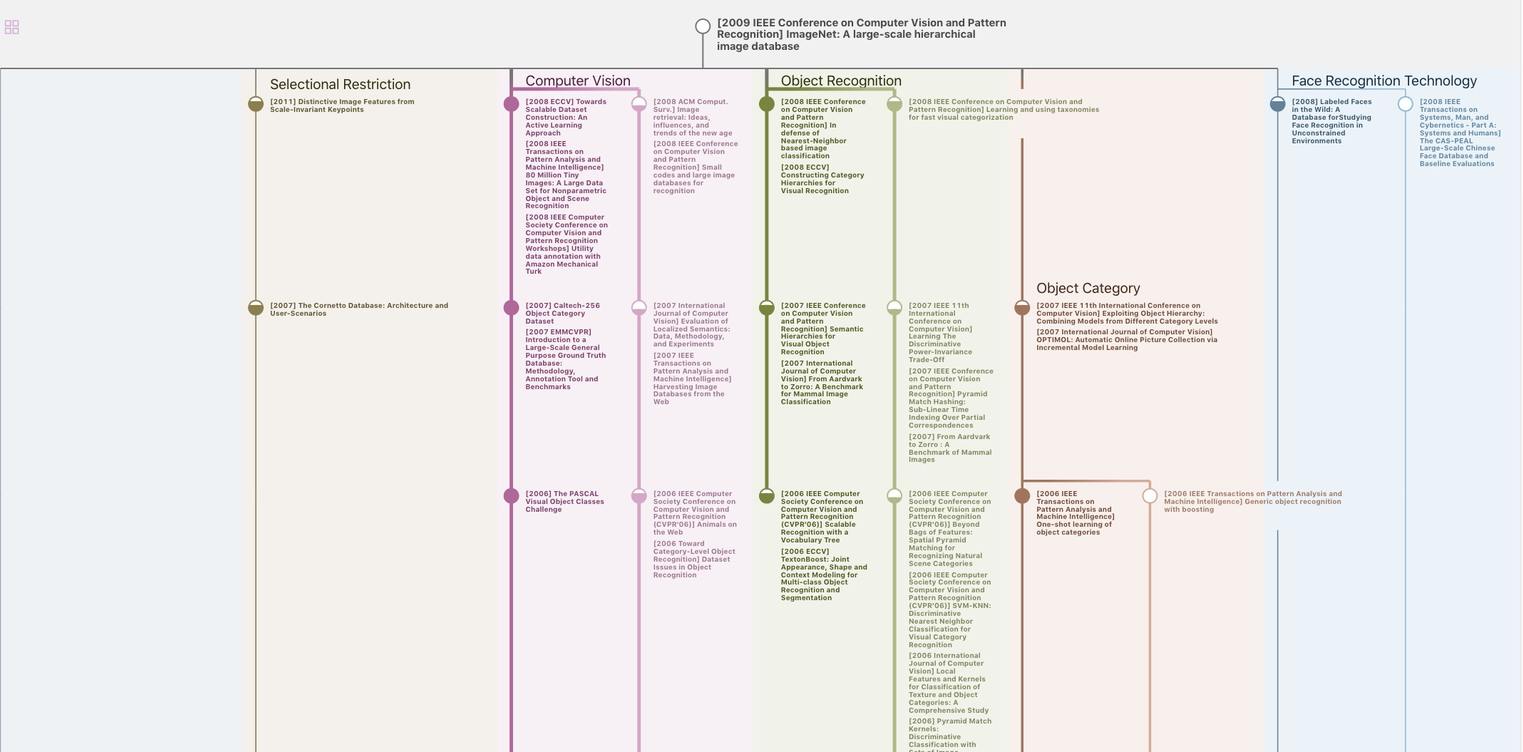
生成溯源树,研究论文发展脉络
Chat Paper
正在生成论文摘要