Deep learning theory of distribution regression with CNNs
Adv. Comput. Math.(2023)
摘要
We establish a deep learning theory for distribution regression with deep convolutional neural networks (DCNNs). Deep learning based on structured deep neural networks has been powerful in practical applications. Generalization analysis for regression with DCNNs has been carried out very recently. However, for the distribution regression problem in which the input variables are probability measures, there is no mathematical model or theoretical analysis of DCNN-based learning theory. One of the difficulties is that the classical neural network structure requires the input variable to be a Euclidean vector. When the input samples are probability distributions, the traditional neural network structure cannot be directly used. A well-defined DCNN framework for distribution regression is desirable. In this paper, we overcome the difficulty and establish a novel DCNN-based learning theory for a two-stage distribution regression model. Firstly, we realize an approximation theory for functionals defined on the set of Borel probability measures with the proposed DCNN framework. Then, we show that the hypothesis space is well-defined by rigorously proving its compactness. Furthermore, in the hypothesis space induced by the general DCNN framework with distribution inputs, by using a two-stage error decomposition technique, we derive a novel DCNN-based two-stage oracle inequality and optimal learning rates (up to a logarithmic factor) for the proposed algorithm for distribution regression.
更多查看译文
关键词
Learning theory, Deep learning, Distribution regression, Deep CNN, Oracle inequality, ReLU, .
AI 理解论文
溯源树
样例
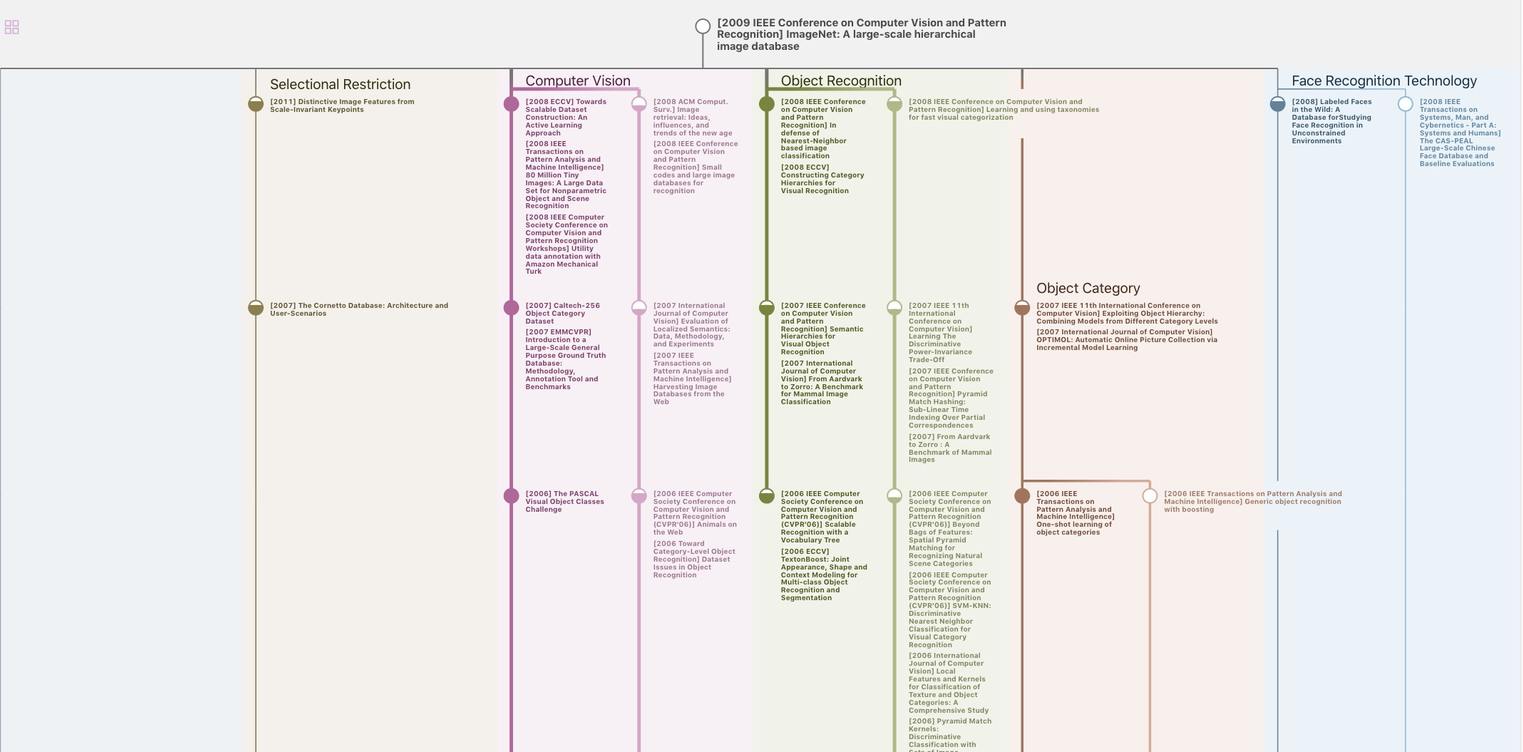
生成溯源树,研究论文发展脉络
Chat Paper
正在生成论文摘要