CTGAN-MOS: Conditional Generative Adversarial Network Based Minority-Class-Augmented Oversampling Scheme for Imbalanced Problems
IEEE Access(2023)
摘要
This paper proposes a novel data augmentation scheme called the conditional generative adversarial network minority-class-augmented oversampling scheme (CTGAN-MOS) for solving class imbalance problems. Our methodology encompassed six key steps: data engineering using sophisticated pre-processing techniques, identifying the type of vulnerabilities present in the data, curating good quality synthetic data using the CTGAN model, the intelligent fusion of real and synthetic data, noise removal from the augmented data using coin-throwing algorithm, and building classifiers with the high-quality augmented data. Our scheme maintains higher structural similarity (data truthfulness) between the original and the resampled data by intelligently adding high-quality samples only to the minority class, whereas some augmentation techniques add records to the majority class, leading to poor-quality resampled data. Our scheme removes noisy samples from the data, which has remained unexplored in the CTGAN-based data augmentation. Furthermore, it augments data by adding fewer records compared to existing schemes, while offering comparable performance. Experiments are conducted on benchmark datasets to prove the feasibility of the proposed CTGAN-MOS in realistic scenarios. Results prove the improvement by CTGAN-MOS over existing state-of-the-art (SOTA) techniques in terms of accuracy, recall, precision, F1 score, and G-mean score. Specifically, the CTGAN-MOS has yielded accuracy values of 100% and 99.83% on two datasets which are higher than recent SOTA techniques. On average, it has yielded the 22.58% and 29.47% improvements w.r.t. G-mean score on two different datasets. On average, it adds 8.26% and 26.01% fewer records than the existing SOTA methods in the two datasets. Lastly, our scheme yields highly balanced confusion matrices compared to recent SOTA data augmentation techniques.
更多查看译文
关键词
~Imbalanced problem,data augmentation,machine learning,classifiers,noise,majority class,minority class,model training,samples,intelligent fusion,data truthfulness,data engineering
AI 理解论文
溯源树
样例
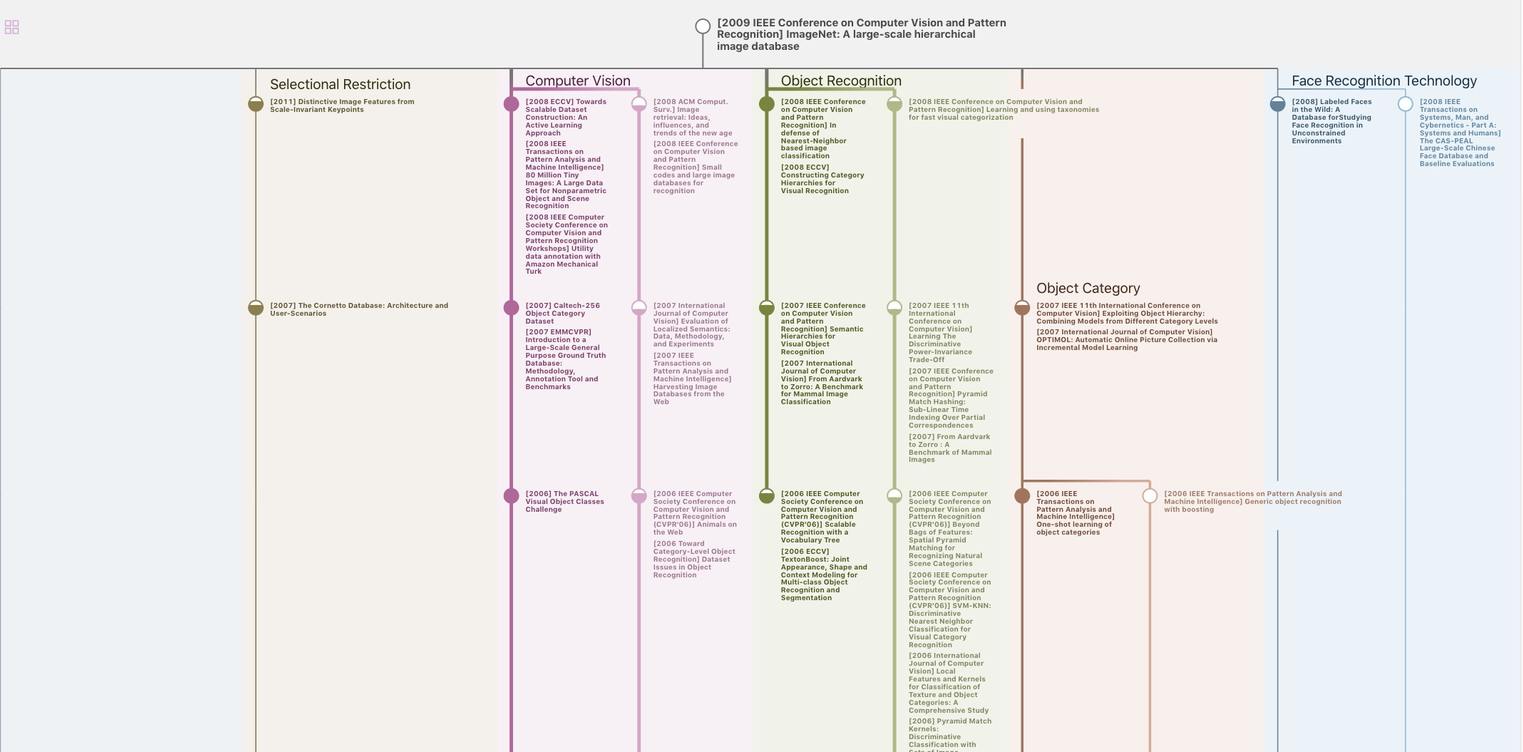
生成溯源树,研究论文发展脉络
Chat Paper
正在生成论文摘要