A hybrid estimation procedure for modeling shallow foundation's settlement: RBF-optimized neural network.
J. Intell. Fuzzy Syst.(2023)
摘要
The complexity of the cohesive soil structure necessitates settlement modeling beneath shallow foundations. The goal of this research is to use recently discovered machine learning techniques called the hybridized radial basis function neural network (RBFNN) with sine cosine algorithm (SCA) and firefly algorithm (FFA) to detect settlement (Sm) of shallow foundations. The purpose of using optimization methods was to find the optimal value for the primary attributes of the model under investigation. With R-2 values of at least 0.9422 for the learning series and 0.9271 for the assessment series, both the produced SCA - RBFNN and FFA - RBFNN correctly replicated the Sm, which indicates a considerable degree of efficacy and even a reasonable match between reported and modeled Sm. In comparison to FFA - RBFNN and ANFIS - PSO, the SCA - RBFNN is believed to be the more correct method, with the values of R-2, RMSE and MAE was 0.9422, 7.2255mm and 5.1257 mm, which is superior than ANFIS - PSO and FFA - RBFNN. The SCA - RBFNN could surpass FFA one by 25% for the learning component and 14.2% for the test data, according to the values of PI index. Ultimately, it is apparent that the RBFNN combined with SCA could score higher than the FFA and even the ANFIS - PSO, which is the proposed system in the Sm forecasting model, after assessing the reliability and considering the assumptions.
更多查看译文
关键词
shallow foundations,hybrid estimation procedure,neural network,settlement,rbf-optimized
AI 理解论文
溯源树
样例
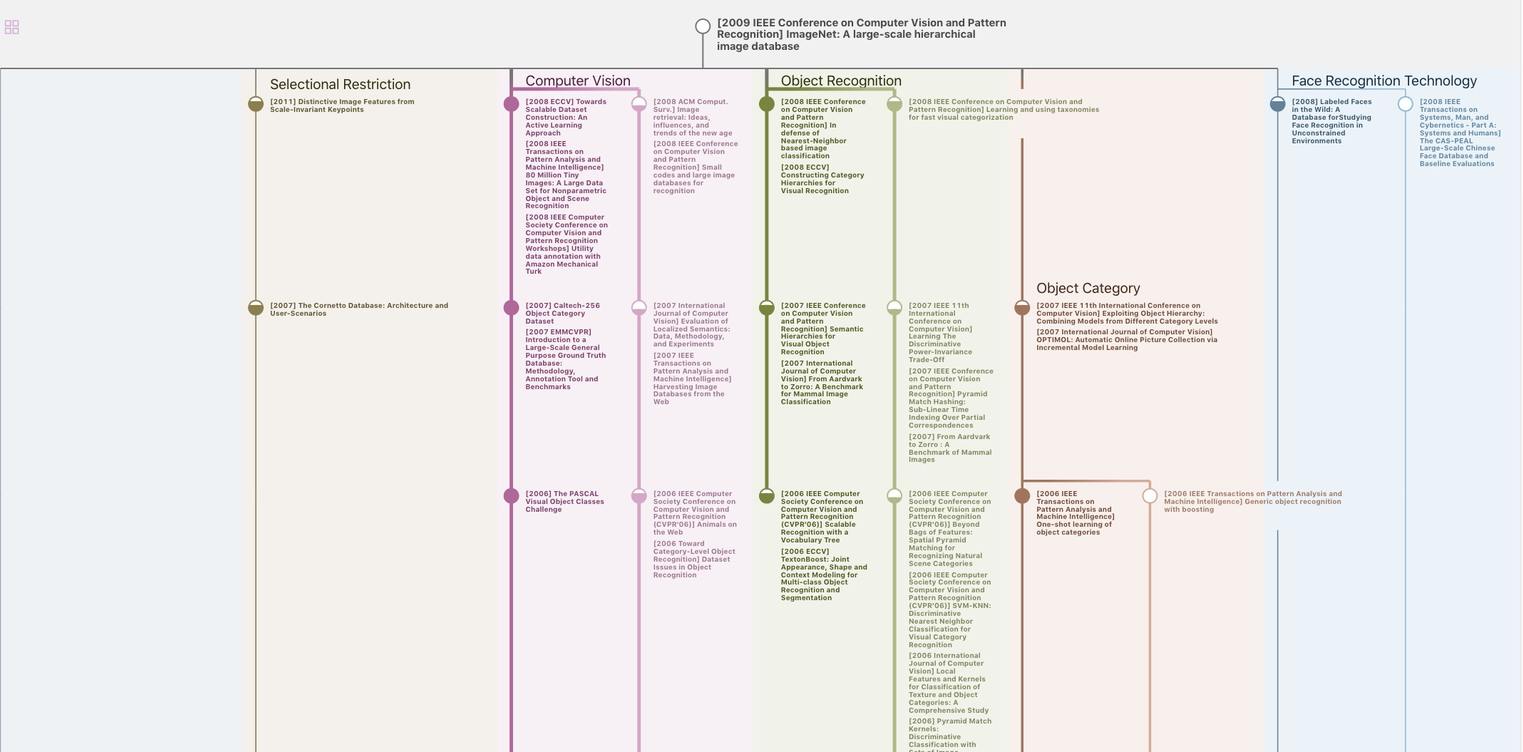
生成溯源树,研究论文发展脉络
Chat Paper
正在生成论文摘要