Multi-Grained Temporal Segmentation Attention Modeling for Skeleton-Based Action Recognition.
IEEE Signal Process. Lett.(2023)
Abstract
The Transformer network has been widely studied for skeleton-based action recognition, and existing methods have made significant progress. However, these methods still suffer from severe overfitting issues and are less effective at capturing local relationships compared to methods based on graph convolutional neural networks (GCN). Building on previous research on attention, we propose MTS-Former, a novel multi-granularity temporal segmentation attention-based modeling method, to address these challenges. MTS-Former dynamically learns the topology of the skeleton by position-sensitive axis-attention, eliminating the constraints of manually crafted adjacency matrices. Furthermore, it effectively reduces overfitting by combining attention-guided regularization. It models the global-local relationship of skeleton sequences through segmental sampling and a multi-granularity aggregation strategy, thus enabling more robust local motion feature extraction. MTS-Former outperforms state-of-the-art Transformer networks on four benchmark datasets, DHG, SHREC, NUT RGB+D 60, and NTU RGB+D 120.
MoreTranslated text
Key words
Index Terms-Attention, computational modeling, skeleton, topology, transformer
AI Read Science
Must-Reading Tree
Example
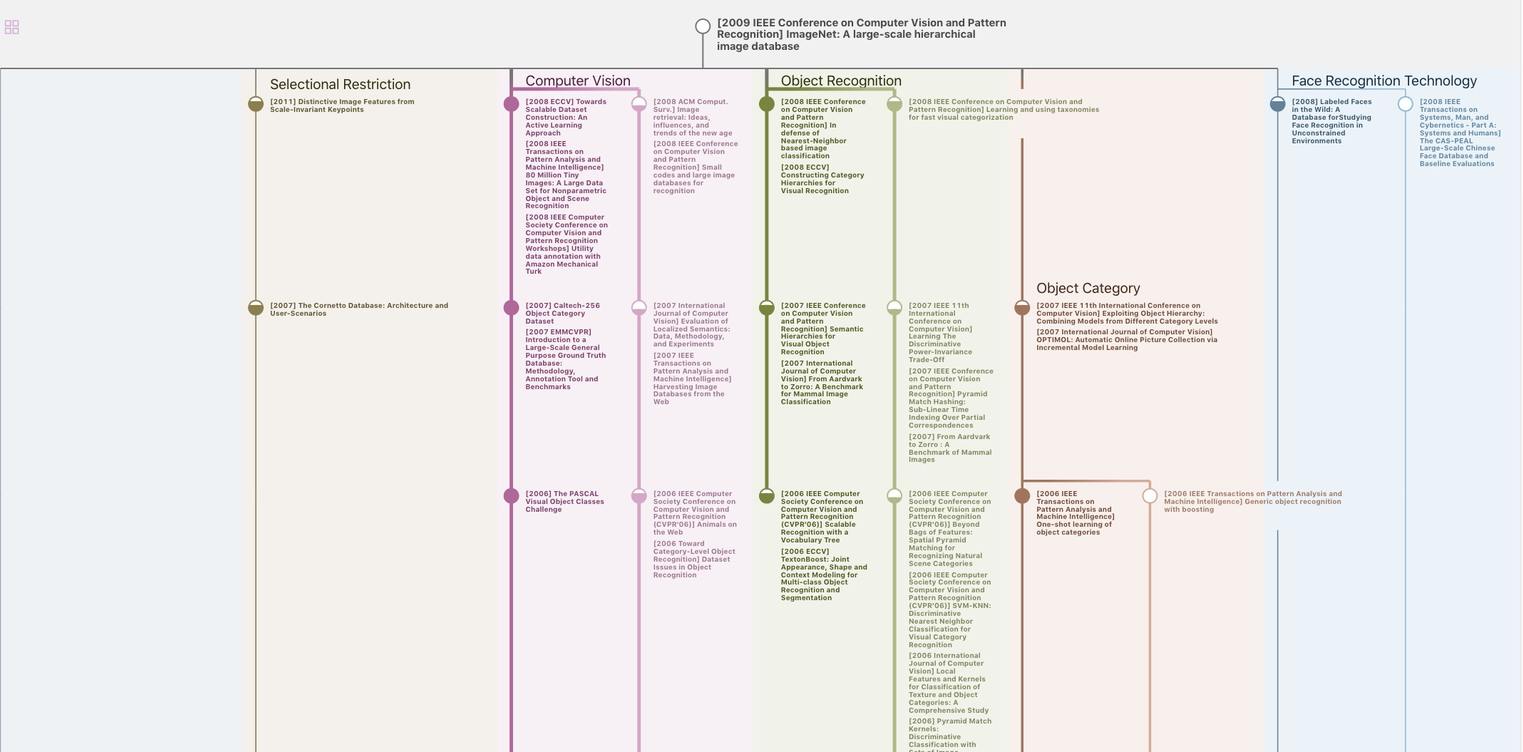
Generate MRT to find the research sequence of this paper
Chat Paper
Summary is being generated by the instructions you defined