Development of machine learning algorithms for predicting internal corrosion of crude oil and natural gas pipelines
Comput. Chem. Eng.(2023)
摘要
Energy industry is losing billions of dollars each year due to corrosion. However, publicly accessible datasets on pipeline corrosion are limited, making it difficult to accurately predict and prevent corrosion. In this research, thousands of corrosion scenarios of crude oil pipelines and natural gas pipelines were simulated using OLI Studio Corrosion Analyzer, a software based on the rigorous first principles. Thousands of datasets were generated with simulation. Then two ML algorithms, Random Forest (RF) and CatBoost were developed and used to predict the internal corrosion rate (CR) based on pipeline operating conditions. The models for crude oil pipeline CR predictions reached high prediction accuracy using five important features: temperature (T), molar concentrations (CO2, CCl - 1), velocity (v), and pH value (pH). Similarly, the models for natural gas pipeline CR predictions achieved high prediction accuracy using six important features: stream compositions (nO2, nH2S, nCO2), pressure (P), velocity (v), and temperature (T). Both the RF- and CatBoost-Corrosion Rate models demonstrated good performance in predicting the internal corrosion rates of crude oil and natural gas pipeline.
更多查看译文
关键词
Internal corrosion rate,Machine learning models,Crude oil pipeline,Natural gas pipeline
AI 理解论文
溯源树
样例
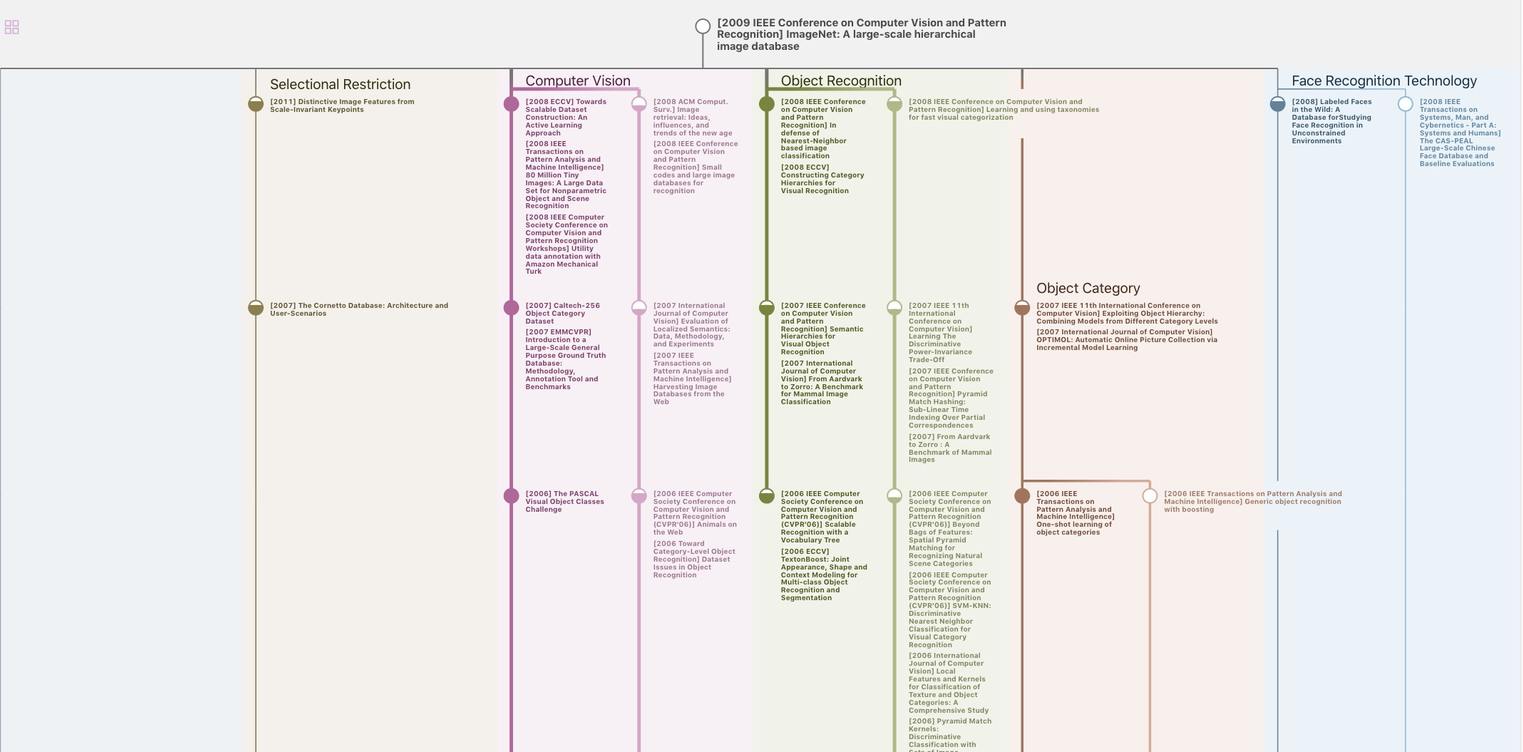
生成溯源树,研究论文发展脉络
Chat Paper
正在生成论文摘要