Semi-Supervised Multiscale Permutation Entropy-Enhanced Contrastive Learning for Fault Diagnosis of Rotating Machinery
IEEE Trans. Instrum. Meas.(2023)
摘要
In recent years, deep learning-based methods have attracted much attention and achieved remarkable results for intelligent fault diagnosis of rotating machinery. However, in many actual industrial scenes, there are few labeled and large unlabeled samples that can be collected for learning due to the cost of experiments, which affect seriously the performance of supervised learning methods. A novel semi-supervised fault diagnosis method based on multiscale permutation entropy (MPE) enhanced contrastive learning (CL) is proposed. First, each channel signal of labeled and unlabeled samples collected in fault diagnosis experiments is expanded into 2-D matrices by minimizing MPE and encoded into gray Euclidean distance plots (EPs). Second, these EPs of different channels of each sample are aggregated and spliced into a color EP. CL model is employed as a pretraining model to learn deep features of color EPs of all labeled and unlabeled samples. Finally, the few labeled samples are inputted into ResNet-18 to obtain good classification performance with the learned feature weights by the CL pretraining model. The applications of gearbox and milling tool fault diagnosis experiments show the classification accuracy of the proposed MPE-CL method outperforms significantly other five benchmark methods, which are above 95.4% and 96.0% when the sample size in the labeled training dataset is 50/class, respectively.
更多查看译文
关键词
Contrastive learning (CL),fault diagnosis,multiscale permutation entropy (MPE),rotating machinery,semi-supervised learning
AI 理解论文
溯源树
样例
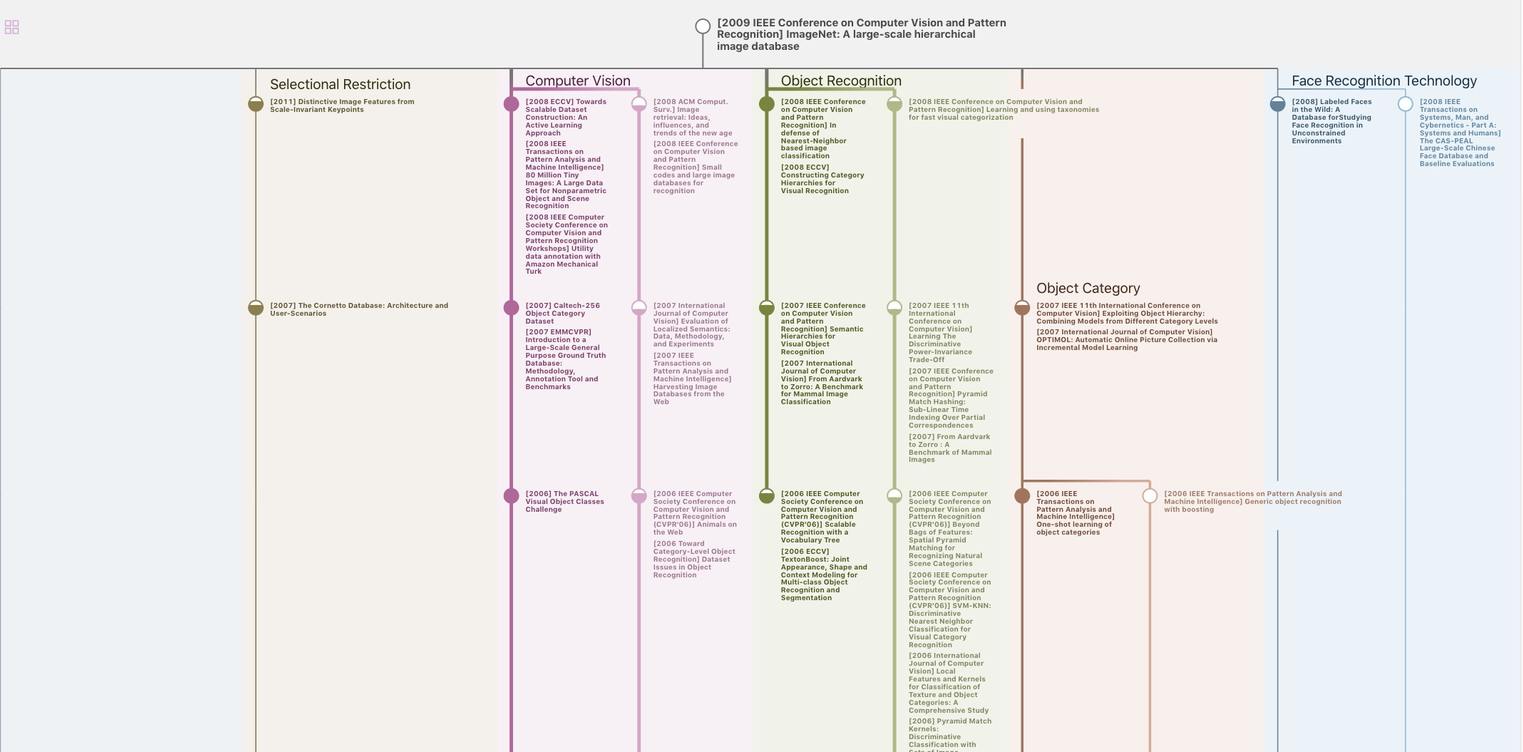
生成溯源树,研究论文发展脉络
Chat Paper
正在生成论文摘要