Feature Clustering-Based Network for Industrial Process Diagnosis With Incremental Fault Types.
IEEE Trans. Instrum. Meas.(2023)
摘要
When new faults are identified in complex industrial processes, the model parameters in neural networks can be incrementally updated to adapt to new diagnosis tasks. However, the catastrophic forgetting problem inevitably decreases the diagnosis performance. Network expansion is a feasible solution for current diagnosis models. In this article, a new two-stage diagnosis model based on feature clustering and network expansion is designed to adapt to new diagnosis tasks. In the first stage, the samples of different faults are transformed into different feature clusters. In the second stage, the sample is identified by its feature similarities with different feature clusters. The model is expanded only when the existing features fail to distinguish the new faults. It expands slower than the existing models. The proposed model is verified on the Tennessee-Eastman (TE) process and the three-phase flow (TPF) facility. The results show that the proposed method outperforms 1%-5% better than other incremental fault diagnosis methods.
更多查看译文
关键词
Catastrophic forgetting,fault diagnosis,feature clustering,incremental learning,network expansion
AI 理解论文
溯源树
样例
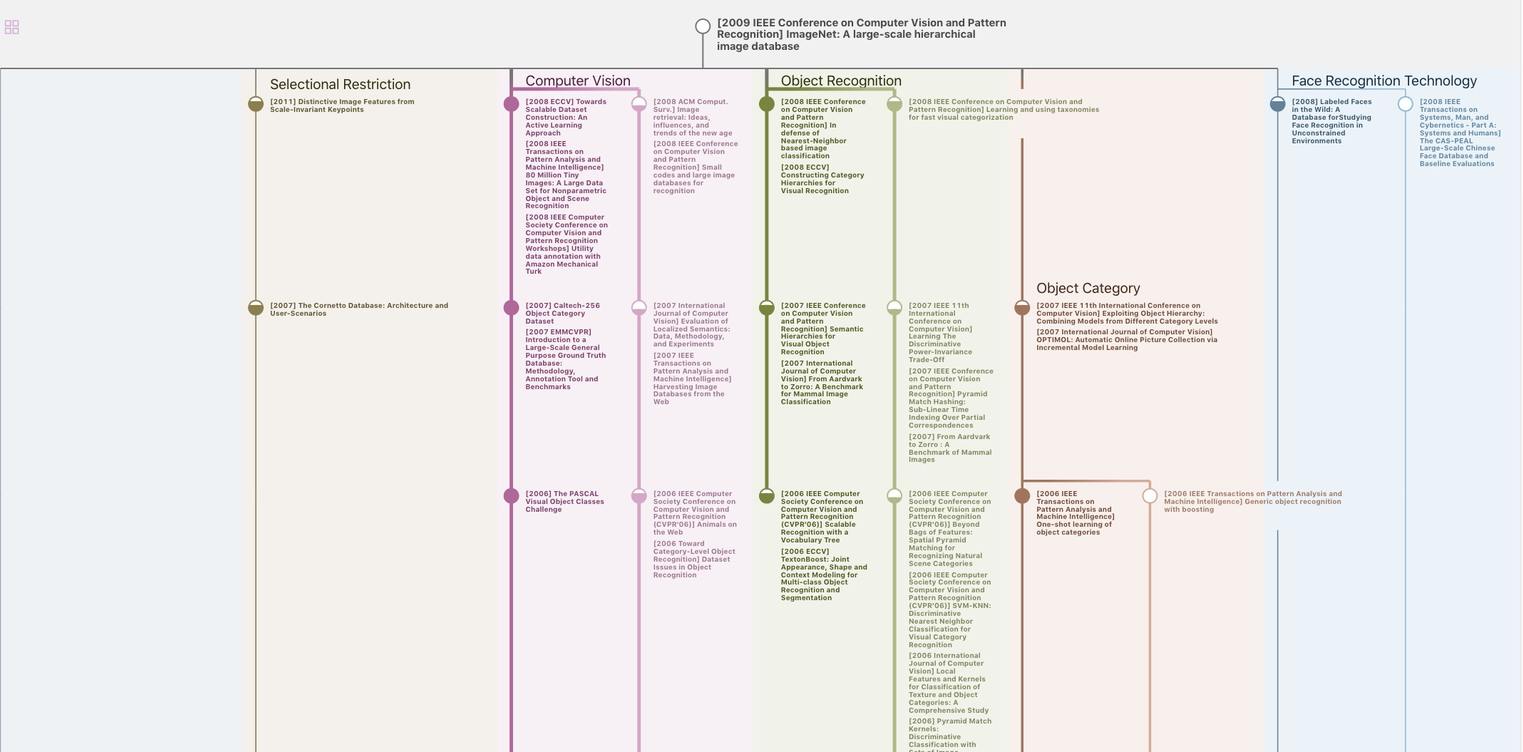
生成溯源树,研究论文发展脉络
Chat Paper
正在生成论文摘要