An Unsupervised Deep Learning Method for Direct Seismic Deblending in Shot Domain.
IEEE Trans. Geosci. Remote. Sens.(2023)
摘要
By increasing the source density, the blended data can significantly improve the seismic data quality. However, the blended data cannot be directly used in the subsequent seismic processing process, and it is necessary to separate the useful signals from the crosstalk noise. For a high-density acquisition with the denser shot coverage survey (DSCS), we propose an unsupervised deep learning (UDL) method to directly deblend the blended data in the common shot gather (CSG). Both the useful signal and the crosstalk noise in the pseudo-deblended data (PDD) are continuously coherent in CSG. By minimizing the final total loss function, the similar coherent signals from the PDD of the main and second sources are extracted using the proposed UDL method. The UDL consists of two U-nets, which extract similar features from the PDD of the main and second sources in the training stage, and directly obtain the deblended results in the test stage. The original unblended data, which may be needed for supervised learning, are not available from the actual collected blended data. However, the proposed UDL method does not require the original unblended data as training data, which solves the lack of training data. The proposed UDL method can be applied to the CSG without providing the delay time and the blending operator, so it has more flexibility. Synthetic data and field data examples show that the proposed UDL method can effectively and directly deblend the blended data in CSG under DSCS.
更多查看译文
关键词
direct seismic deblending,unsupervised deep learning method,deep learning,domain
AI 理解论文
溯源树
样例
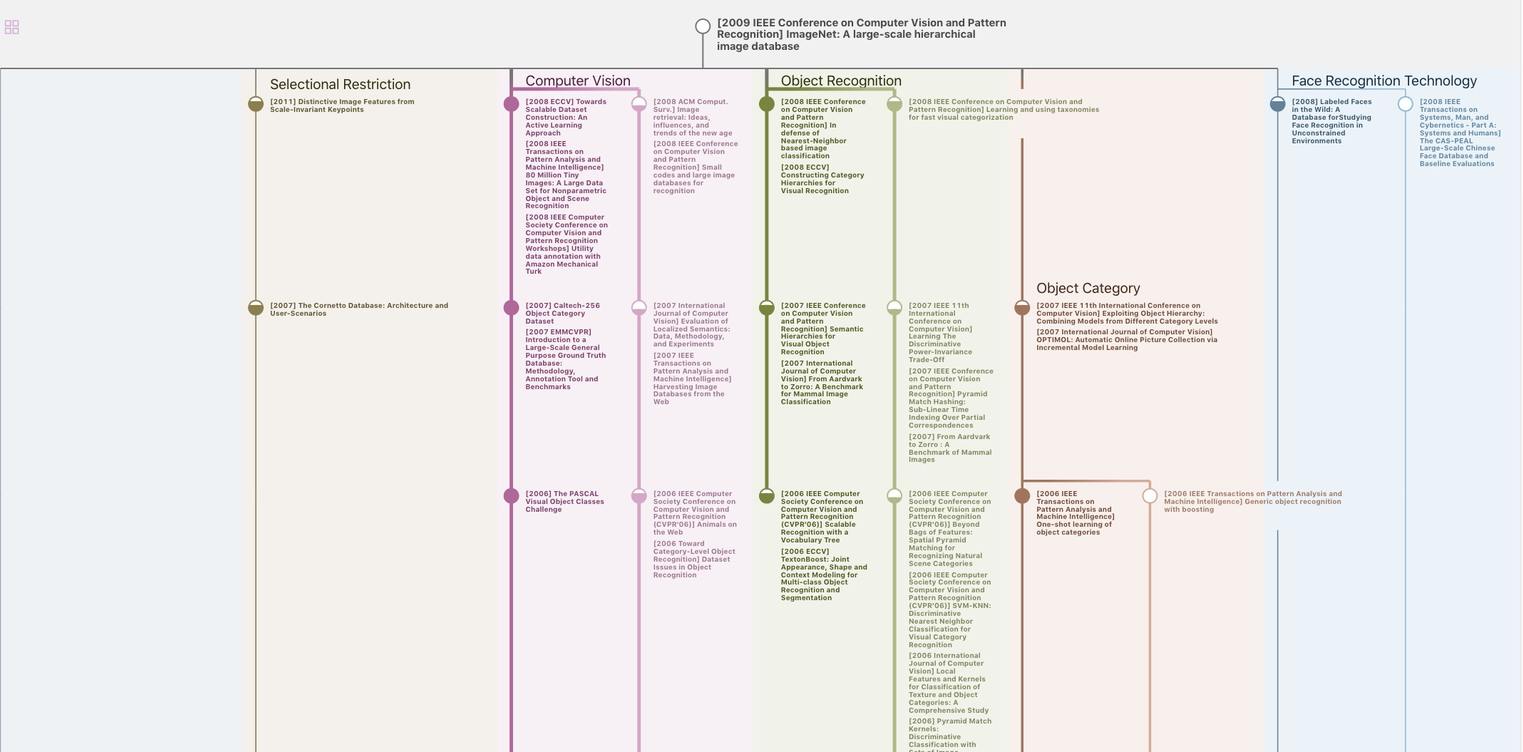
生成溯源树,研究论文发展脉络
Chat Paper
正在生成论文摘要