IPIML: A Deep-Scan Earthquake Detection and Location Workflow Integrating Pair-Input Deep Learning Model and Migration Location Method.
IEEE Trans. Geosci. Remote. Sens.(2023)
Abstract
Optimized deep learning (DL)-based workflows can improve the efficiency and accuracy of earthquake detection and location processes. This article introduces a six-step automated event detection, phase association, and earthquake location workflow, which integrates the state-of-the-art pair-input DL (PIDL) model and waveform migration location methods [integrated PIDL and MIL (IPIML)]. Applying IPIML on an 18-month dataset of Ghana Digital Seismic Network (GHSDN) recorded from 2012 to 2014, a catalog with 461 events is automatically obtained. Compared to other DL catalogs obtained using EQTransformer (EQT) and Siamese EQT (S-EQT), the seismic event clusters in the IPIML catalog focus more on tectonically active regions or known seismogenic source areas and show a consistent depth distribution. The compiled catalog is 6.3x larger than the reported catalog obtained by applying EQT with the default settings, indicating the importance of optimization and hyperparameter tuning when applying DL models. As a result, a previously unknown seismogenic fault with a clear spatial trend has been identified using the new IPIML catalog, which provides more insights into the fault activities and seismic hazards in the region. The IPIML codes and datasets are available at the GitHub repository https://github.com/SigProSeismology/IPIML.git, contributing to the geoscience community.
MoreTranslated text
Key words
Earthquake detection and location, pair-input deep learning (PIDL), waveform migration location (MIL) method
AI Read Science
Must-Reading Tree
Example
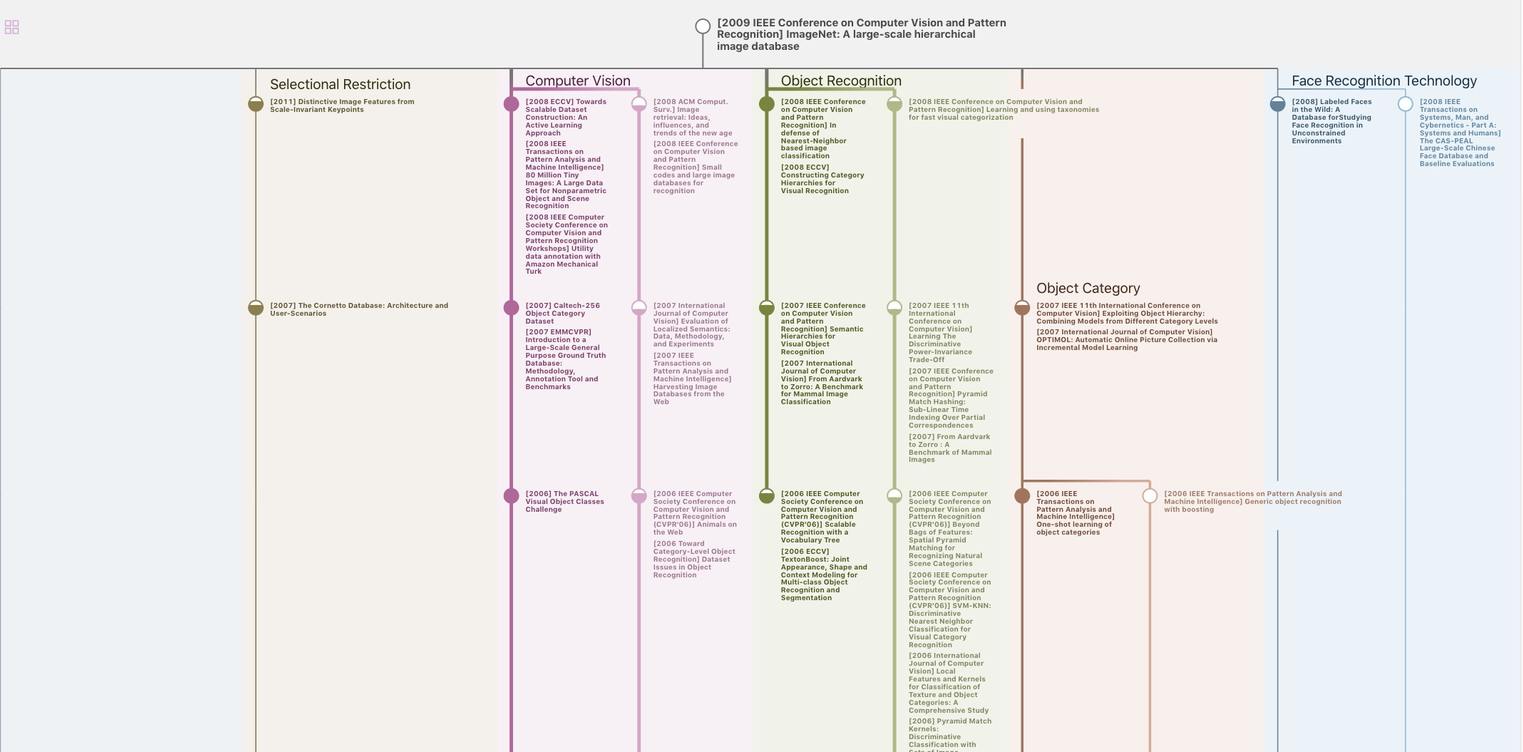
Generate MRT to find the research sequence of this paper
Chat Paper
Summary is being generated by the instructions you defined