MarsFormer: Martian Rock Semantic Segmentation With Transformer
IEEE Trans. Geosci. Remote. Sens.(2023)
摘要
Semantic segmentation of Mars scenes has a crucial role in Mars rovers science missions. Current convolutional neural network (CNN)-based composition of U-Net has powerful information extraction capabilities; however, convolutional localization suffers from the limited global context modeling capability. Although transformer global modeling has performed well, it still encounters obstacles in the extraction and retention of low-level features. This issue is particularly relevant for Martian rocks with their varying shapes, textures, and sizes in Mars scenes. In this article, we propose a novel transformer semantic segmentation framework for Martian rock images, called MarsFormer, that consists of an encoder-decoder structure connected through a feature enhancement module (FEM) and a window transformer block (WTB). Specifically, multiscale hierarchical features are generated by the mix transformer (MiT) encoders, upgraded-FFN decoder (UFD) fuse and filter features at different scales, preserving the rich local and global contextual information. FEM enhances the inter-multiscale feature correlation from both spatial and channel perspectives. WTB captures the long-range contexts and preserves the local features. We built two datasets of synthetic and real Martian rocks. The synthetic dataset is SynMars, referencing data from the ZhuRong rover taken from its virtual terrain engine. The other dataset is MarsData-V2, from real Mars scenes, and published recently in our previous study. Extensive experiments conducted on both datasets showed that MarsFormer achieves superiority in Martian rock segmentation, obtaining state-of-the-art performance with favorable computational simplicity. The data are available at: https://github.com/CVIR-Lab/SynMars.
更多查看译文
关键词
Mars exploration,semantic segmentation,synthetic Mars,transformer model
AI 理解论文
溯源树
样例
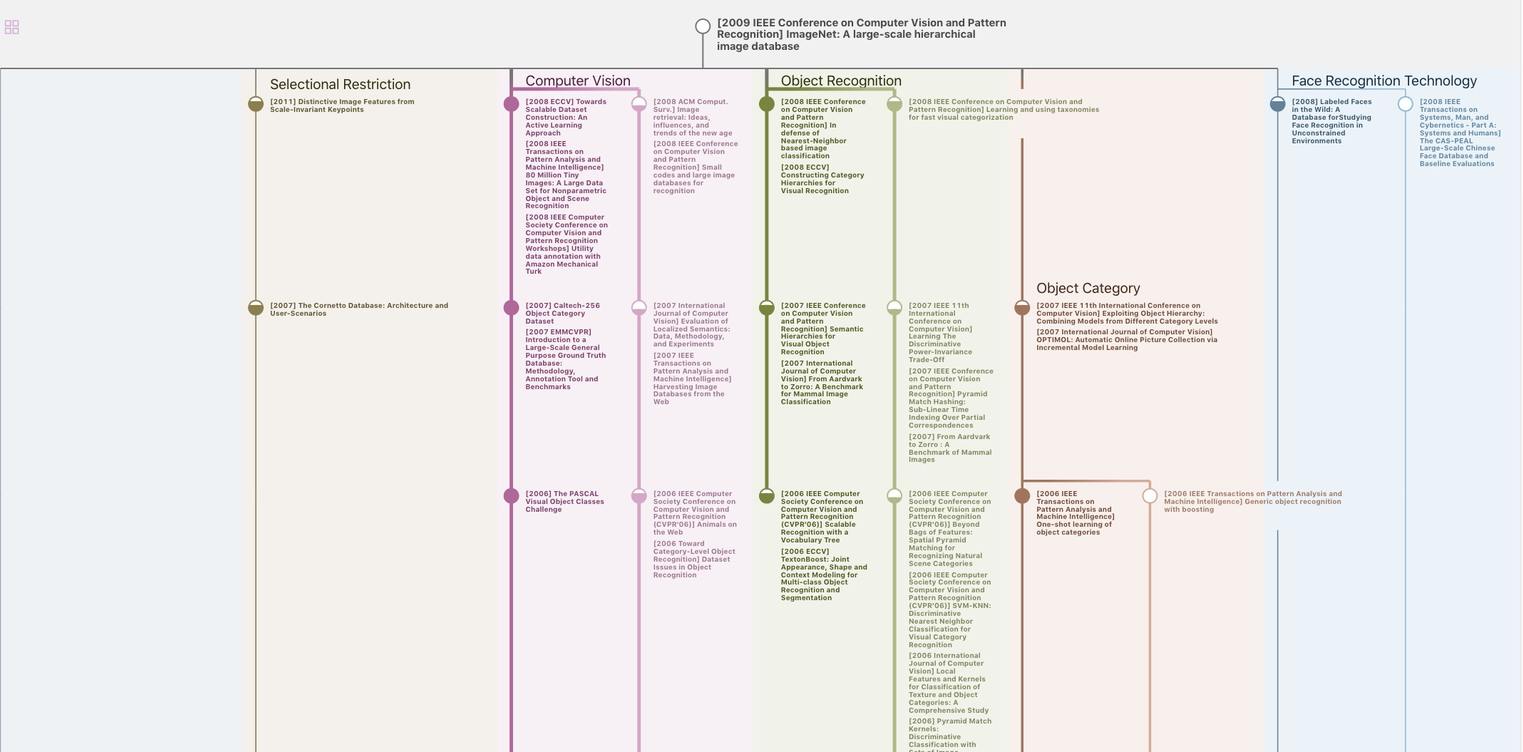
生成溯源树,研究论文发展脉络
Chat Paper
正在生成论文摘要