Contrastive Learning Based on Category Matching for Domain Adaptation in Hyperspectral Image Classification.
IEEE Trans. Geosci. Remote. Sens.(2023)
摘要
Cross-scene hyperspectral image classification (HSIC) is a challenging topic in remote sensing, especially when there are no labels in the target domain. Domain adaptation (DA) techniques for cross-scene HSIC aim to label a target domain by associating it with a labeled source domain. Most existing DA methods learn domain-invariant features by reducing feature distance across domains. Recently, contrastive learning has shown excellent performance in computer vision (CV) tasks, but there is little or no research on the performance of cross-scene HSIC. Considering that its idea is similar to reducing feature distance, this article attempts to explore whether contrastive learning can achieve cross-scene HSIC. In this work, an instance-to-instance contrastive learning framework based on category matching (CLCM) is designed. The main idea is to take the category information as the premise in the feature space, regard the source sample as an anchor, and find its positive and negative matching samples across domains. The instance-level discriminative feature embeddings are learned through positive matching pairs attracting each other and negative matching pairs repelling each other. Among them, the target label is a pseudo-label. To further improve the quality of contrastive learning, it is considered to focus on extracting the spectral-spatial features of hyperspectral images (HSIs) to more accurately represent semantic information. Simultaneously, high-confidence target samples are screened to update the network. Three DA tasks confirm the effectiveness and feature discriminativeness of CLCM, while also providing new ideas for cross-scene image classification.
更多查看译文
关键词
hyperspectral image classification,domain adaptation,category matching,learning
AI 理解论文
溯源树
样例
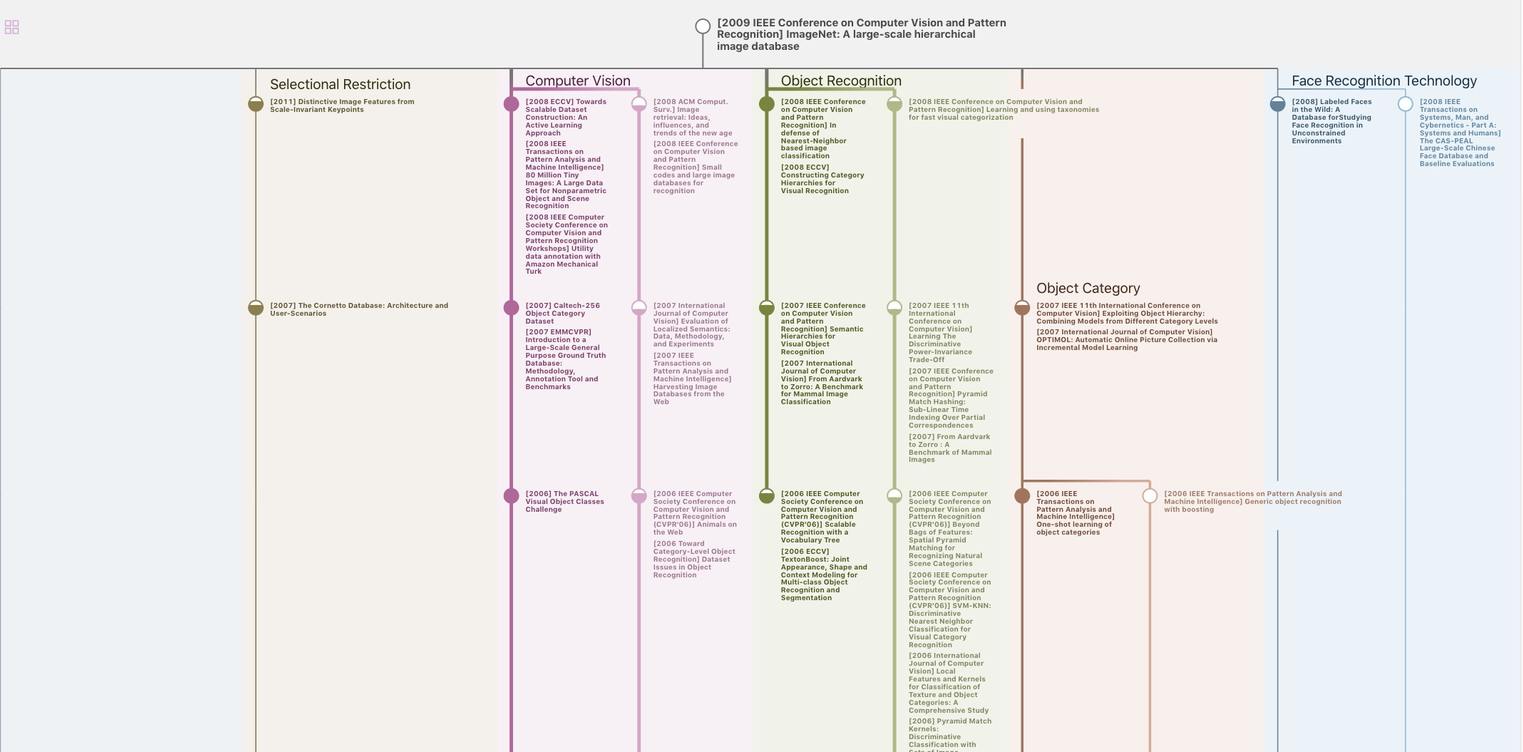
生成溯源树,研究论文发展脉络
Chat Paper
正在生成论文摘要