ASHFormer: Axial and Sliding Window-Based Attention With High-Resolution Transformer for Automatic Stratigraphic Correlation.
IEEE Trans. Geosci. Remote. Sens.(2023)
Abstract
The stratigraphic correlation of well logs is crucial for characterizing subsurface reservoirs. However, due to the complexity of well logs and the huge amount of well data, manual correlation is time- and resource-intensive. Hence, various computerized stratigraphic correlation methods have been developed, especially regarding convolutional neural networks (CNNs). Recently, transformer, a self-attention system that evolved from the natural language processing (NLP), has attained state-of-the-art (SOTA) performance over CNNs in a variety of domains because of its ability to perceive global features. We propose the axial and sliding window-based attention with high-resolution transformer (ASHFormer), combining the high-resolution network (HRNet) with an axial and sliding window self-attention block (ASBlock) intended for stratigraphic correlation of well logs. ASBlock includes three different forms of multihead self-attentions (MHSAs), including sliding-window attention, horizontal-axis attention, and vertical-axis attention, therefore, it is possible to retrieve well logs' long-range and local information. The experiments show that ASHFormer predicts more accurate stratigraphic correlation results than HRNet and CNNs meet transformer (CMT) (a transformer combining CNN and self-attention). The usefulness of the transformer for well log feature extraction and automatic stratigraphic correlation is demonstrated by ASHFormer's 9.74% improvement in correlation accuracy over HRNet with the same architecture.
MoreTranslated text
Key words
axial,attention,high-resolution high-resolution,ashformer,window-based
AI Read Science
Must-Reading Tree
Example
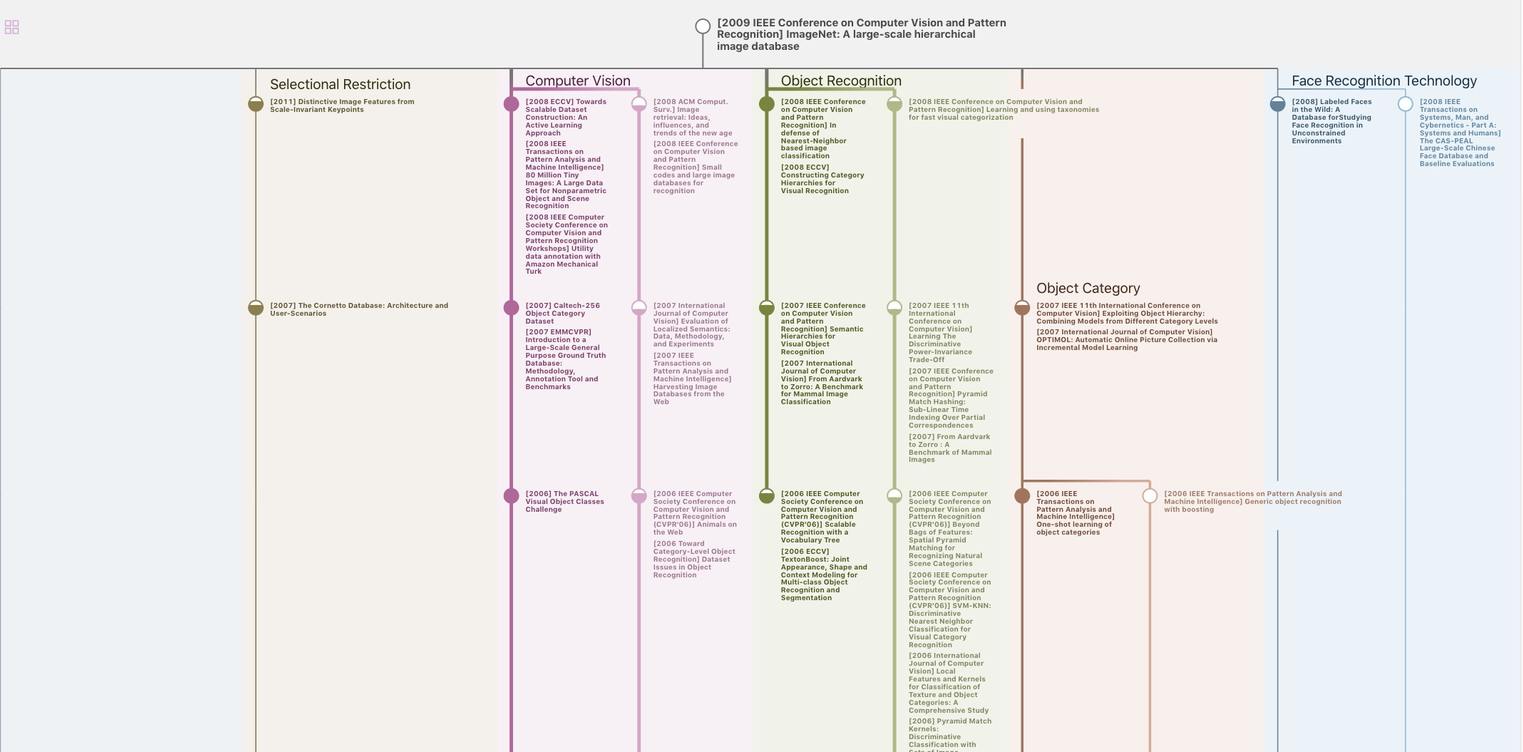
Generate MRT to find the research sequence of this paper
Chat Paper
Summary is being generated by the instructions you defined