A Potential Solution to Insufficient Target-Domain Noise Data: Transfer Learning and Noise Modeling.
IEEE Trans. Geosci. Remote. Sens.(2023)
摘要
Recently, a number of deep learning (DL) methods are developed to attenuate the noise in seismic data. Most of them show good performance under a common precondition: the training and testing data are drawn from the same distribution. However, it is challenging to acquire sufficient noise training data whose distribution is the same as the noise presented in the testing seismic data; we call such noise data with the same distribution as target-domain noise. To address this issue, we propose a promising DL paradigm for seismic data denoising based on transfer learning and seismic noise modeling. We first utilize a Green-function-based modeling method for seismic noise to generate a massive amount of synthetic noise, which is similar to real seismic noise. Second, the high-authenticity synthetic noise is used as the pretraining data in the source domain. Finally, we utilize limited real target-domain noise data to fine-tune the partial trainable parameters of pretrained model, thus transferring it into the target domain. This proposed DL paradigm gets rid of the need for enough target-domain noise data, so as to extend the application scope of DL-based method in seismic data denoising. Moreover, we design a novel network architecture based on multicascade structure and attention mechanism. This DL paradigm shows extremely similar denoising performance to that of using a large amount of target-domain noise data in both synthetic and real examples, demonstrating its potential in mitigating the dependence of DL-based seismic denoising methods on target-domain noise data.
更多查看译文
关键词
transfer learning,noise,target-domain
AI 理解论文
溯源树
样例
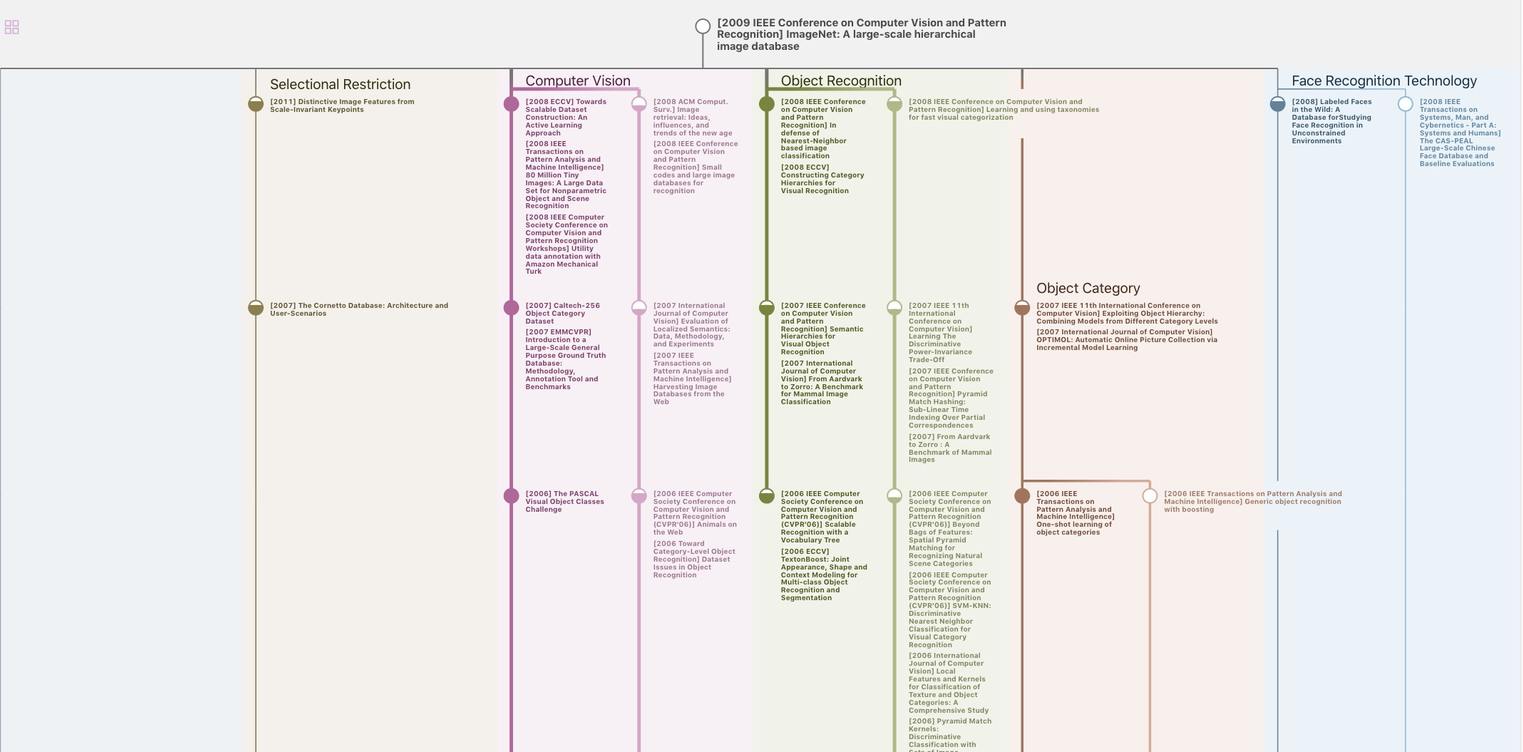
生成溯源树,研究论文发展脉络
Chat Paper
正在生成论文摘要