I-FLASH: Interpretable Fake News Detector Using LIME and SHAP
Wirel. Pers. Commun.(2023)
摘要
The rise of social media enables people to disseminate information. However, when false but appealing information is presented as news, it becomes a cause for serious concern as it might lead to a harmful influence on communities of innocent believers. To address this issue, we propose I-FLASH, an interpretable fake news detector that not only detects fake news but also explains why it considers some content fake or genuine. Moreover, recent research evaluated their models for fake news detection on domain-specific datasets. Therefore, in this paper, two new tiny datasets, FactCheck and FactCheck2, were culled from the official Twitter accounts/websites of various well-known media outlets, covering a variety of other societal domains such as education, crime, and technology. We also compared the performance of the machine learning model (logistic regression with TF-IDF), deep learning model (bidirectional LSTM with GloVe word embeddings), and the pre-trained Bidirectional Encoder Representations from Transformers (BERT) model on curated datasets along with two other popular datasets, namely, LIAR and COVID-19. The stratified 10-fold cross-validation accuracy of 94.41 ± 0.38% on the COVID-19 dataset, 61.18 ± 0.55% on the LIAR dataset, 87.25 ± 2.45% on FactCheck, and 92.91 ± 2.07% on FactCheck2, attained at 95% confidence interval, establishes the efficacy of models. On cross-dataset validation, we observe that the model trained on a generalized dataset like FactCheck2 can perform well on domain-specific datasets like COVID-19 and LIAR with a validation accuracy of 64.25% and 54.22%, respectively. Finally, using XAI methods—LIME and SHAP revealed important terms while predicting the news class (fake/real).
更多查看译文
关键词
Explainable AI,Fake news detection,BERT,LIME,SHAP
AI 理解论文
溯源树
样例
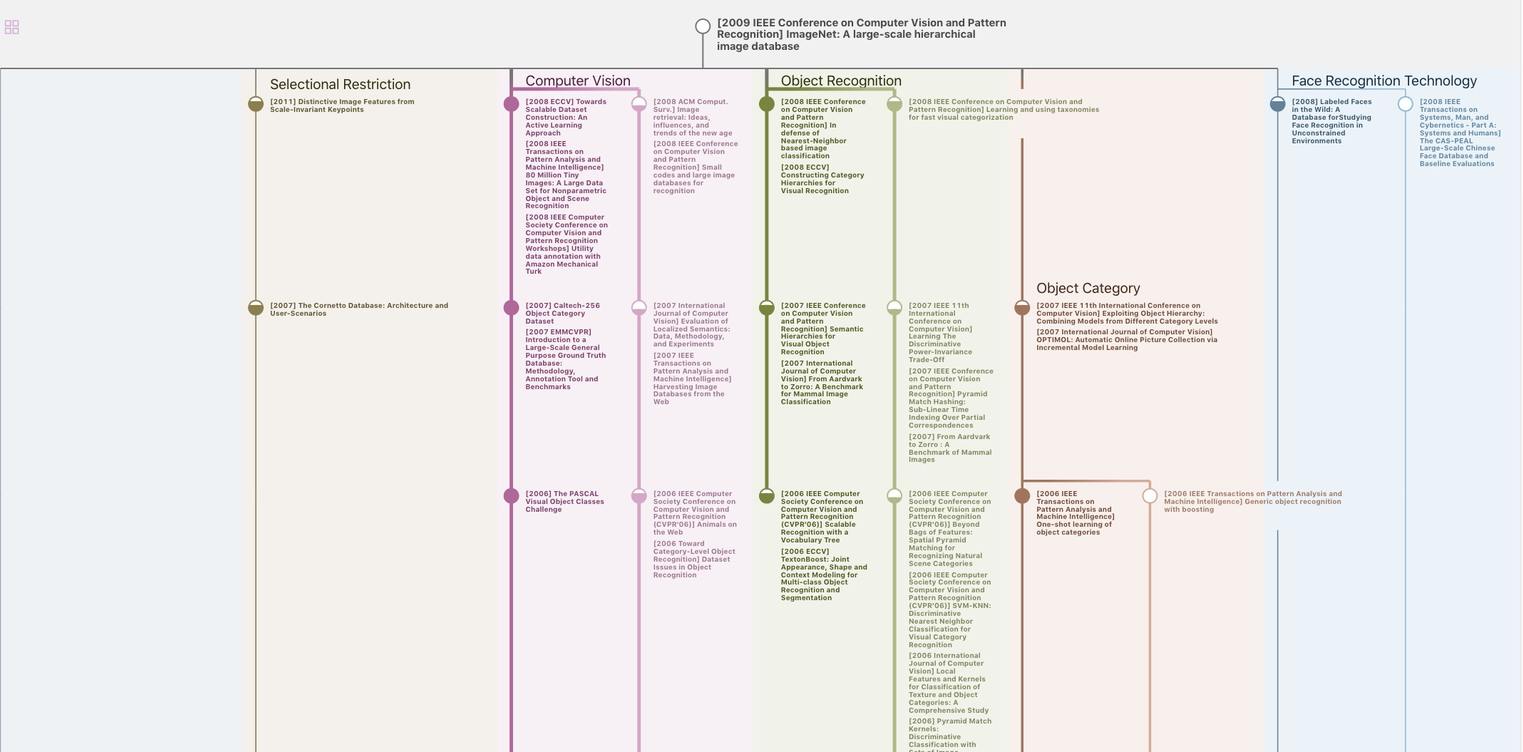
生成溯源树,研究论文发展脉络
Chat Paper
正在生成论文摘要