A high-level feature channel attention UNet network for cholangiocarcinoma segmentation from microscopy hyperspectral images
Mach. Vis. Appl.(2023)
摘要
Pathological diagnosis is the gold standard for the diagnosis of cholangiocarcinoma. The manual segmentation of pathology sections is time-consuming. Automatic segmentation has become a clinical requirement. Recently, the UNet network has been widely used in automatic segmentation; however, due to the complex structure and diverse shapes of pathological slices of cholangiocarcinoma, the segmentation ability of UNet is insufficient. In addition, traditional RGB images cannot reflect the spectral characteristics of cancerous tissue. Therefore, in this paper, a high-level feature channel attention UNet (HLCA-UNet) network is proposed to segment cholangiocarcinoma using microscopy hyperspectral images. Compared with the original UNet, HLCA-UNet has the following improvements: (1) a new path consisting of hierarchical feature extraction and channel attention mechanism designed to deliver encoder features to decoder, in which the hierarchical feature extraction module is able to extract high-resolution high-level features and reduce semantic gaps while channel attention mechanism establishes a deep correlation between the low-level and high-level features, (2) we introduce bilinear interpolation technology to replace the transpose convolution so as to achieve a smooth segmentation boundary. We have evaluated the performance of the proposed HLCA-UNet model and compared it with other excellent models using cholangiocarcinoma microscopy hyperspectral images. Experiment results show that HLCA-UNet gains a superior performance, with accuracy, precision, and recall of 82.84%, 69.60%, and 77.99%, respectively. In general, our study provides new ideas for future pathological diagnosis of cholangiocarcinoma and makes recommendations for future researchers.
更多查看译文
关键词
cholangiocarcinoma segmentation,attention,high-level
AI 理解论文
溯源树
样例
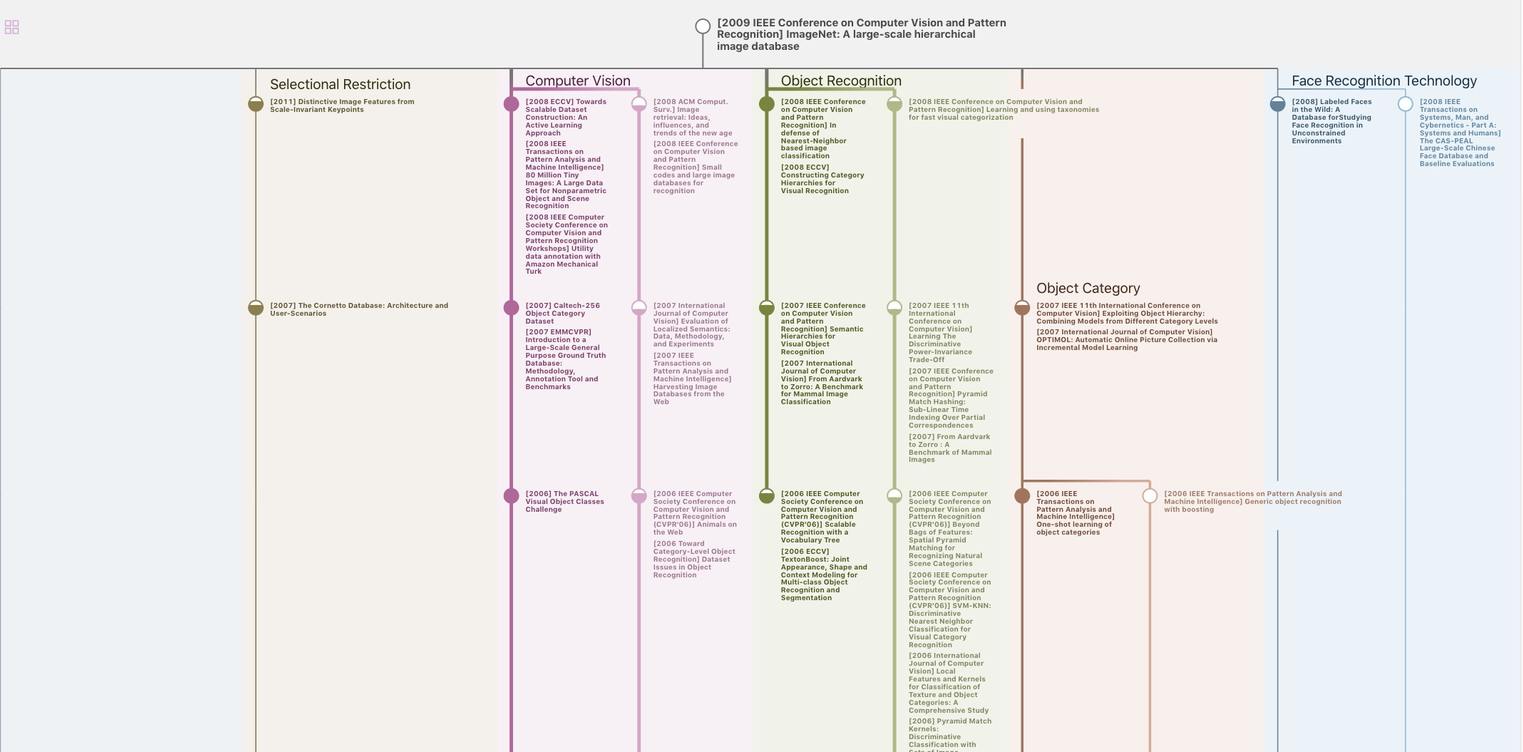
生成溯源树,研究论文发展脉络
Chat Paper
正在生成论文摘要