A multi-graph neural group recommendation model with meta-learning and multi-teacher distillation
KNOWLEDGE-BASED SYSTEMS(2023)
摘要
Group recommendation has garnered significant attention recently, aiming to suggest items of interest to groups. Most deep learning-based approaches for group recommendation focus on learning group representation through group-user interactions or by aggregating user preferences. However, these approaches face challenges due to the complexity of group representation learning and the limited availability of group-item interactions. To address these difficulties, we propose a multi-graph neural group recommendation model with meta-learning and multi-teacher distillation. Our model consists of three stages: multiple graphs representation learning (MGRL), meta-learning-based knowledge transfer (MLKT), and multi-teacher distillation (MTD). In the MGRL stage, we construct two bipartite graphs and an undirected weighted graph based on group-user interactions, group-item interactions, and similarity between groups, respectively. We then utilize a linear variational graph auto-encoder network to learn the semantic features of groups, users, and items. In the MLKT stage, we introduce a multilayer perceptron (MLP) network for the recommendation task and design a meta-learning-based knowledge transfer algorithm to train an initialization parameter that incorporates user preference knowledge. In the MTD stage, we use the trained parameters as initialization parameters for all teacher networks. We evenly divide the group-item interactions among the teachers and sequentially train the teacher networks. Finally, we employ a multi-teacher distillation approach to train student networks with superior performance. Furthermore, we conduct extensive experiments on six real-world datasets to evaluate the effectiveness of our proposed model. The experimental findings demonstrate that our model, in comparison to state-of-the-art approaches, achieves superior performance in terms of group recommendation.(c) 2023 Elsevier B.V. All rights reserved.
更多查看译文
关键词
Group recommendation,Graph auto-encoder,Meta-learning,Knowledge distilling,Deep learning
AI 理解论文
溯源树
样例
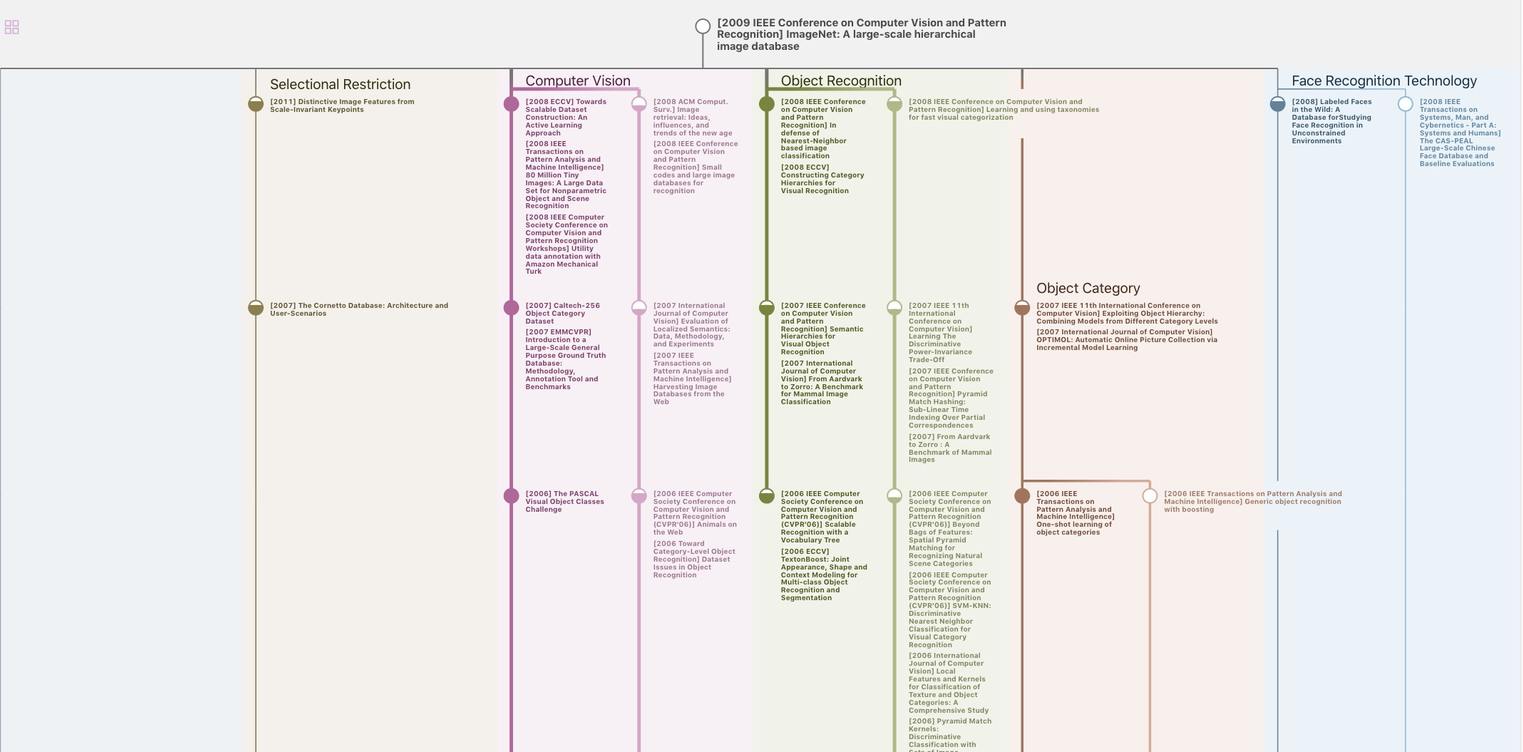
生成溯源树,研究论文发展脉络
Chat Paper
正在生成论文摘要