Enhancing the Reliability of Perception Systems using N-version Programming and Rejuvenation
DSN-W(2023)
摘要
Machine Learning (ML) has become indispensable for real-world complex systems, such as perception systems of autonomous systems and vehicles. However, ML-based systems are sensitive to input data, faults, and malicious threats that can degrade output quality and compromise the complete system's correctness. Ensuring a reliable output of ML-based components is crucial, especially for safety-critical systems. In this paper, we investigate architectures of perception systems using N-version programming for ML to mitigate the dependence on a singular ML component and combine it with a time-based rejuvenation mechanism to maintain a healthy system over extended periods. We propose models and functions to evaluate the reliability of N-version perception systems subject to faults, malicious threats, and rejuvenation. Our numerical experiments show that a rejuvenation mechanism could benefit a multiple-version system, with a reliability improvement superior to 13%. Also, the results indicate that rejuvenation could improve output reliability when ML modules' accuracy is high.
更多查看译文
关键词
Byzantine fault,N-version system,Machine learning,Perception,Rejuvenation,Reliability
AI 理解论文
溯源树
样例
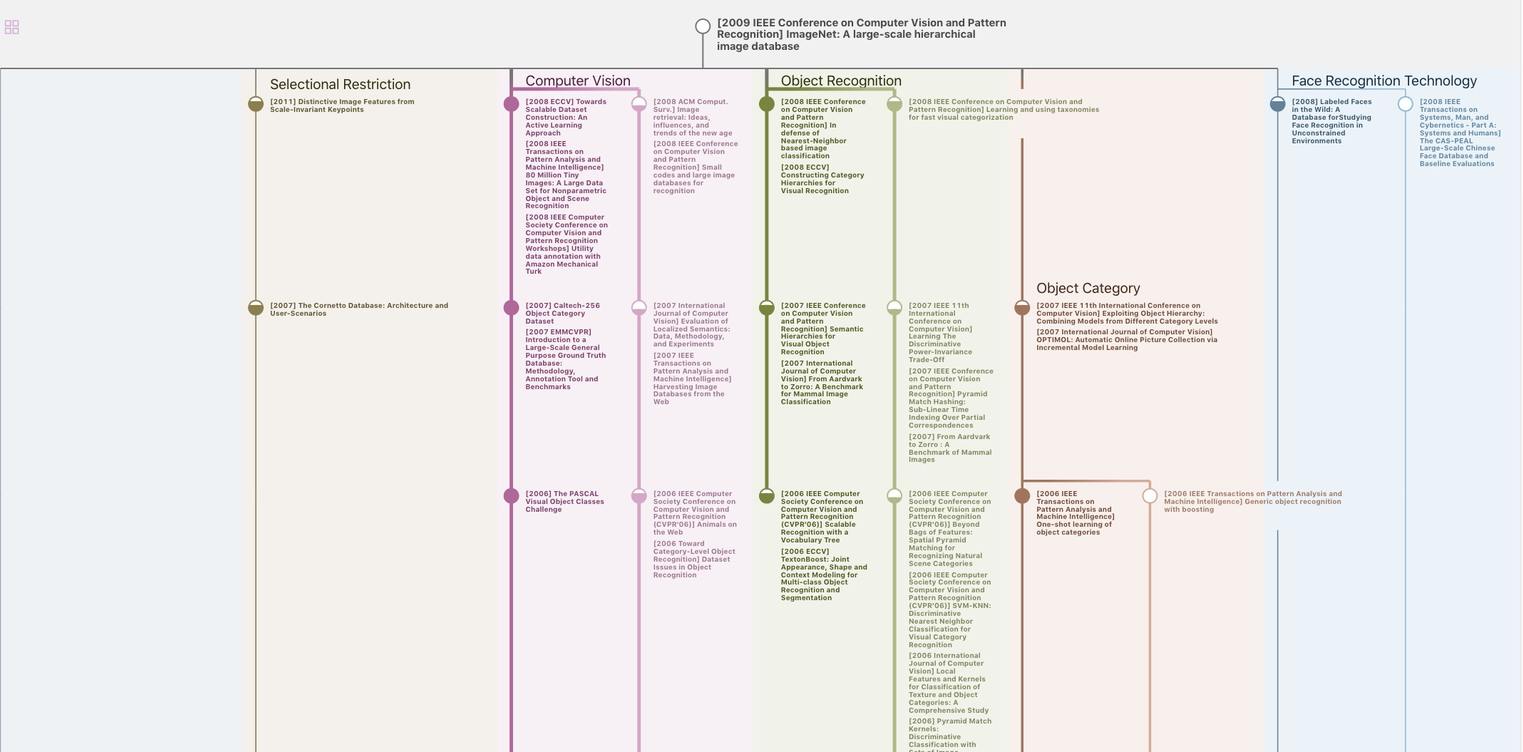
生成溯源树,研究论文发展脉络
Chat Paper
正在生成论文摘要