DeepMAT: Predicting Metabolic Pathways of Compounds Using a Message Passing and Attention-Based Neural Networks.
ICIC (3)(2023)
摘要
The study of compounds and their metabolic pathways is crucial for predicting the presence of compounds in metabolic pathways based on the molecular properties, which can be used for drug design and metabolic pathway reconstruction. To accurately reconstruct metabolic pathways, predicting which compounds belong to specific pathways is necessary. While several computational methods have been proposed for this task, they can only map compounds to metabolic pathway classes and not actual metabolic pathways. Furthermore, similarity and feature-engineering-based methods are proposed to predict actual metabolic pathways. However, problems arise when the similarity score is below 50%, and similarity score calculations can be computationally intensive and time-consuming, especially when dealing with large datasets. To address these limitations, this paper proposes a message-based neural network (DeepMAT) by integrating a Message passing neural network (MPNN) and a multi-head attention Transformer encoder to predict the actual metabolic pathways involved by compounds. The purpose of multi-head attention transformer encoder integration is to calculate the overall information of the entire graph by calculating the influence of each node’s neighbors. Experimental results show that integrating a Message-passing network into a Transformer-style architecture is more expressive and outperforms other methods.
更多查看译文
关键词
metabolic pathways,neural networks,attention-based
AI 理解论文
溯源树
样例
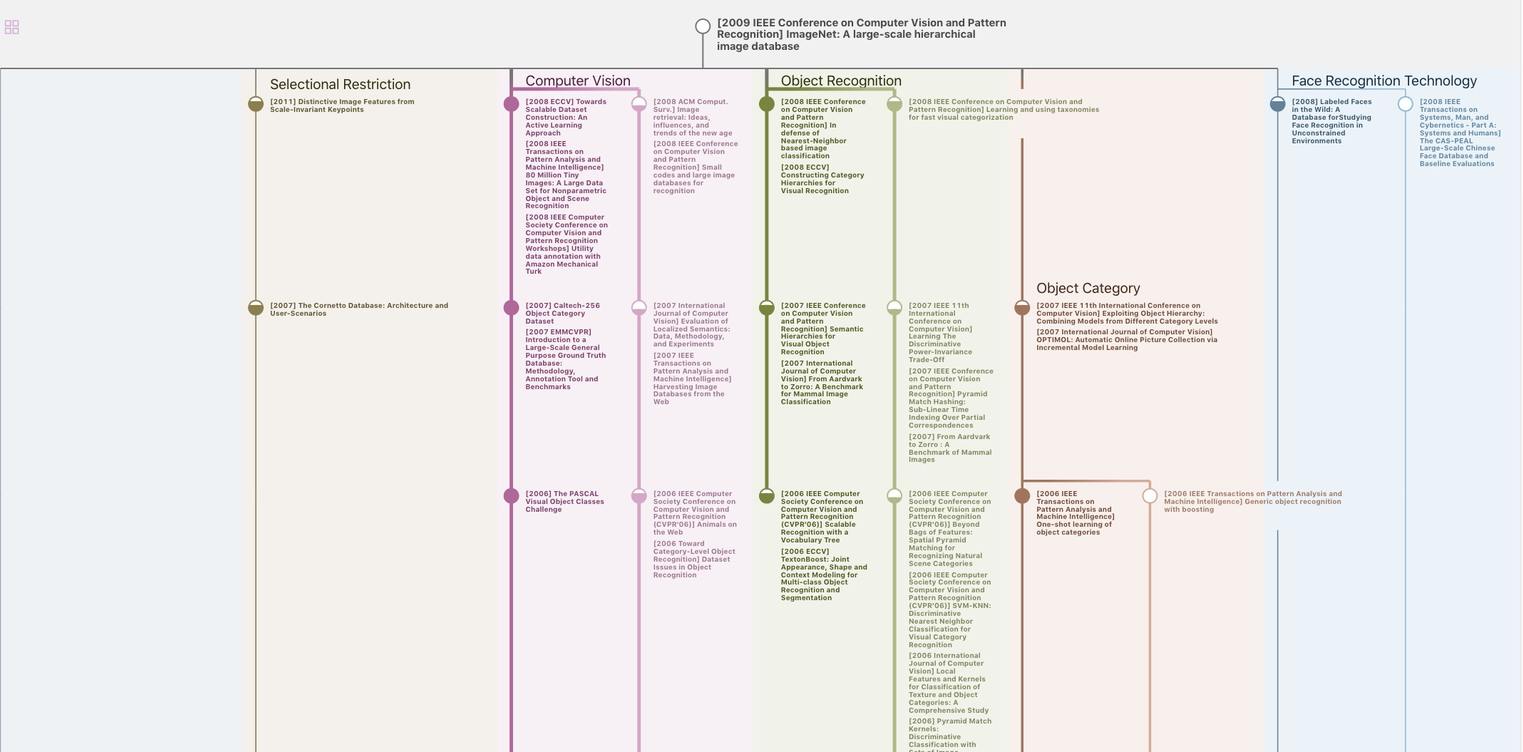
生成溯源树,研究论文发展脉络
Chat Paper
正在生成论文摘要