Accurate Identification of Submitochondrial Protein Location Based on Deep Representation Learning Feature Fusion.
ICIC (3)(2023)
摘要
Mitochondria, comprising two layers of membranes, are indispensable organelles present in most cells. They perform a vital function in generating cellular energy and facilitating aerobic respiration. Experimentally determining the sub-mitochondrial location of proteins is both time-consuming and costly. Therefore, the development of a reliable method to predict the sub-mitochondrial position of mitochondrial proteins is imperative. In this study, we propose a gradient boosting tree (GBDT) based approach to enhance the accuracy of sub-mitochondrial protein localization. To achieve this, we re-divided the benchmark dataset called M317 and utilized deep representation learning to extract features from mitochondrial protein sequences. Additionally, we used Generative Adversarial Network (GAN) to balance the dataset. The extracted features were selected using light gradient boosting machine (LightGBM). In the end, we selected the optimal feature set from the submitochondrial protein features extracted by the TAPE model and combined it with the submitochondrial protein features extracted by the SeqVec model. Subsequently, we inputted the fused features into six traditional machine learning models. We performed tenfold cross-validation experiments on the M317 dataset and achieved high accuracies. The accuracy for inner membrane, matrix, and outer membrane on the M317 dataset were 98.34%, 97.16%, and 98.23%, respectively.
更多查看译文
关键词
submitochondrial protein location,representation
AI 理解论文
溯源树
样例
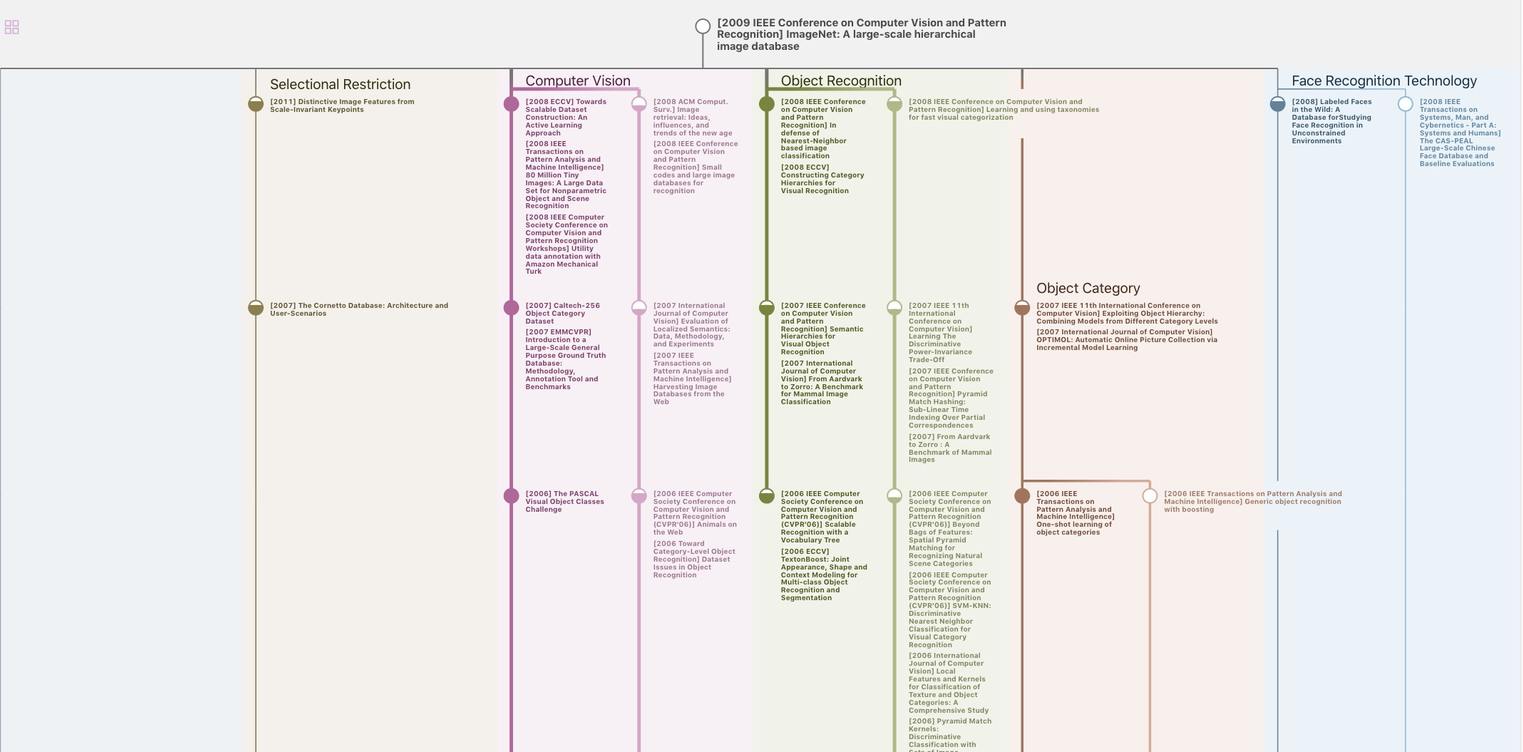
生成溯源树,研究论文发展脉络
Chat Paper
正在生成论文摘要