RNL: A Robust and Highly-Efficient Model for Time-Aware Web Service QoS Prediction.
ICIC (4)(2023)
摘要
Accurately and efficiently predicting unknown time-varying quality-of-service (QoS) data based on observed ones is essential in time-aware Web service selection. A nonnegative latent factorization of tensors (NLFT) model is one of the most attractive approaches to tackle this issue owing to its excellent scalability. Current NLFT models generally adopt L2-norm loss to measure the discrepancy between the predicted time-varying QoS values and observed ones. However, L2-norm is sensitive to outlier data that often exist in observed QoS data, which may significantly impair prediction accuracy. Moreover, a gradient descent-based optimization method is frequently used to build a NLFT-based QoS predictor, yet it may suffer from low computational efficiency. To address these issues, this work proposes a Robust Nonnegative Latent factorization of tensors (RNL) model. It adopts two-fold ideas: a) adopting Cauchy loss to build objective function for achieving strong robustness and high prediction accuracy, b) applying an alternating direction method of multi-pliers (ADMM) principle to design parameters learning scheme for obtaining high computational efficiency. Experimental results on two time-varying QoS datasets demonstrate that the proposed RNL is superior to state-of-the-art models in both prediction accuracy and computational efficiency when they are used to predict the unknown QoS.
更多查看译文
关键词
qos,prediction,service,rnl,highly-efficient,time-aware
AI 理解论文
溯源树
样例
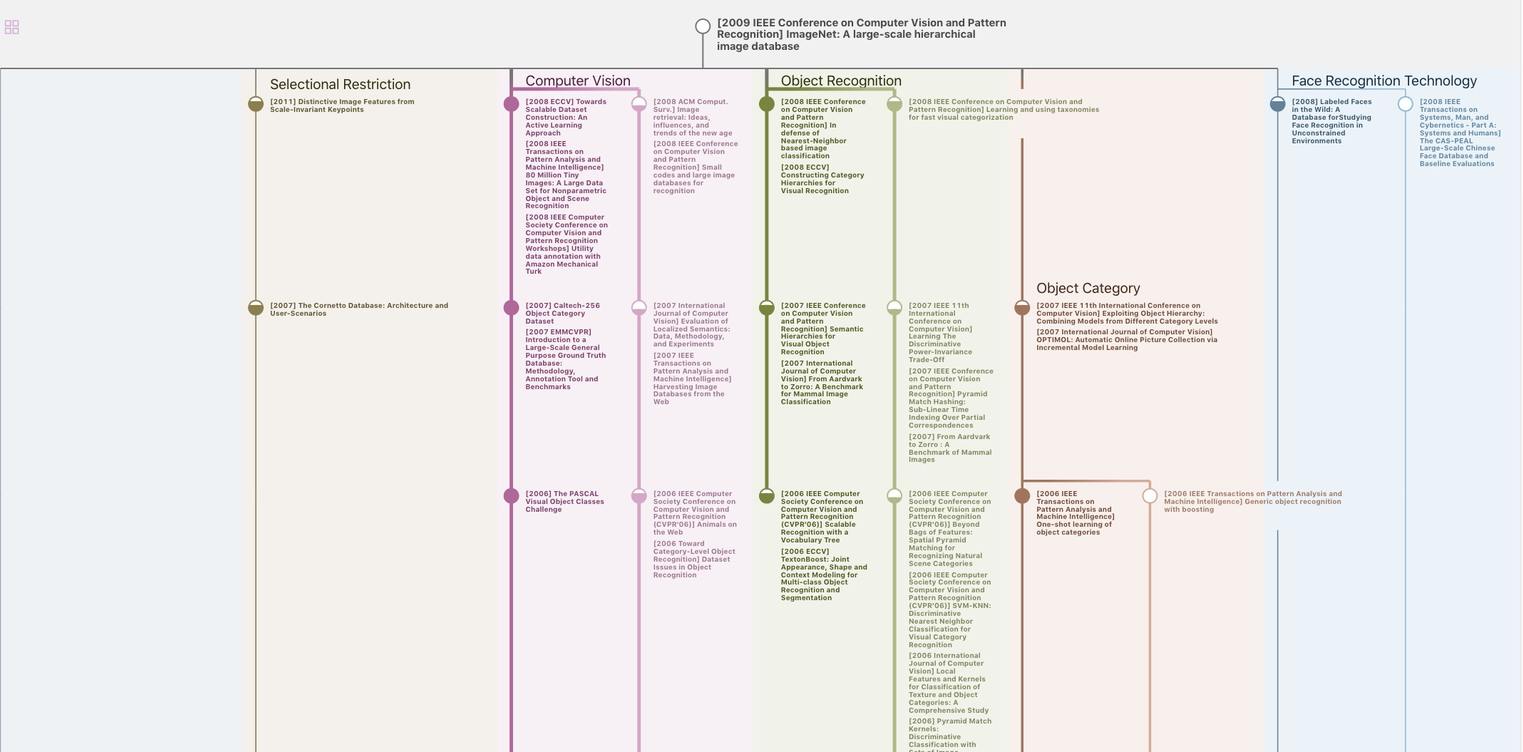
生成溯源树,研究论文发展脉络
Chat Paper
正在生成论文摘要