Multivariate Time Series Anomaly Detection Method Based on mTranAD.
ICIC (4)(2023)
摘要
Multivariate time series anomaly detection is a crucial area of research in several domains, including finance, logistics, and manufacturing. Successfully identifying abnormal behaviors or events can help prevent disruptions, but the high false positive rate in this field is a significant challenge that affects detection accuracy. In this paper, we propose a novel method, mTranAD, which improves upon the TranAD algorithm by leveraging the benefits of Transformer and variational autoencoder (VAE) in multivariate unsupervised anomaly detection. Specifically, mTranAD replaces TranAD’s autoencoder structure with a VAE and trains it using the VAE’s loss function. The incorporation of latent variables in the VAE model enables accurate reconstruction of data and mapping of data to a lower dimensional latent space, allowing for a more efficient description of input data complexity with fewer parameters. By utilizing these latent variables, the model can effectively handle high-dimensional, complex data and exhibit greater flexibility when generating new data. We conduct experiments on four public datasets (NAB, MBA, SMAP and WADI) and compare mTranAD’s performance with 11 other state-of-the-art methods, including TranAD, MERLIN, LSTM-NDT, OmniAnomaly, USAD, and DAGMM. The experimental results demonstrate that mTranAD outperforms these methods in terms of performance, accuracy, and reliability. The primary purpose of this paper is to improve the TranAD algorithm and enhance the accuracy of multivariate time series anomaly detection by reducing the false positive rate.
更多查看译文
关键词
anomaly detection,time series
AI 理解论文
溯源树
样例
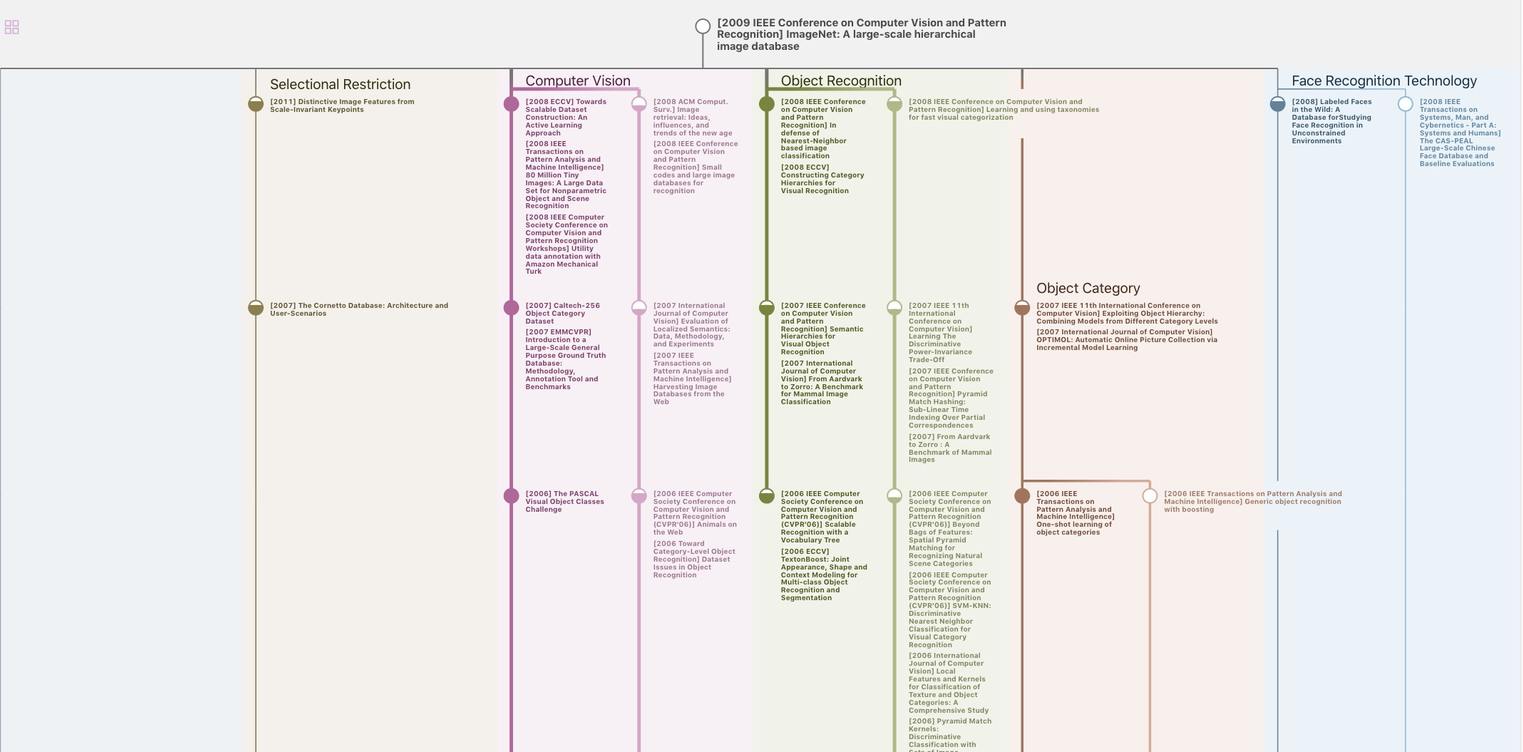
生成溯源树,研究论文发展脉络
Chat Paper
正在生成论文摘要