Improving Causality Explanation of Judge-View Generation Based on Counterfactual.
ICIC (4)(2023)
Abstract
Legal justice predication has attracted wide attentions in both AI research communities and legal research area. With the general pre-trained model employed in many NLP task and get the SOTA performance, many methods of judge view generation in LJP were developed. This sort of methods improved the accuracy in prediction in general. But there are issues remained, one of the fatal problems of them which may hinder the application in real scenarios is the causality explanation of facts to judges. Researches showed big models with good prediction performance can easily make decisions based on spurious correlations from wrong text content in facts description to correct result. Apparently, this would weaken the model’s interpretability, accountability and trustworthy. Furthermore it might hinder the prevalence of AI LJP applications in real legal scenarios. Inspired by the ideas of counterfactuals in causality inference, we investigated its usage in legal AI application. We introduced a method of counterfactuals generation by intervening the raw data to address the data imbalance problem in vertical LJP domain. Combined with the generalization performance of large language model, the related embedding of facts in legal case are more expressive, thus reduced the probability of resulting in potential spurious correlations such as result view inferred from unrelated fact description text. We conduct a comparison experiment to illustrate the performance of our method. The result showed that with counterfactuals intervened in raw data, the performance of legal judge generation can be promoted.
MoreTranslated text
Key words
causality explanation,judge-view
AI Read Science
Must-Reading Tree
Example
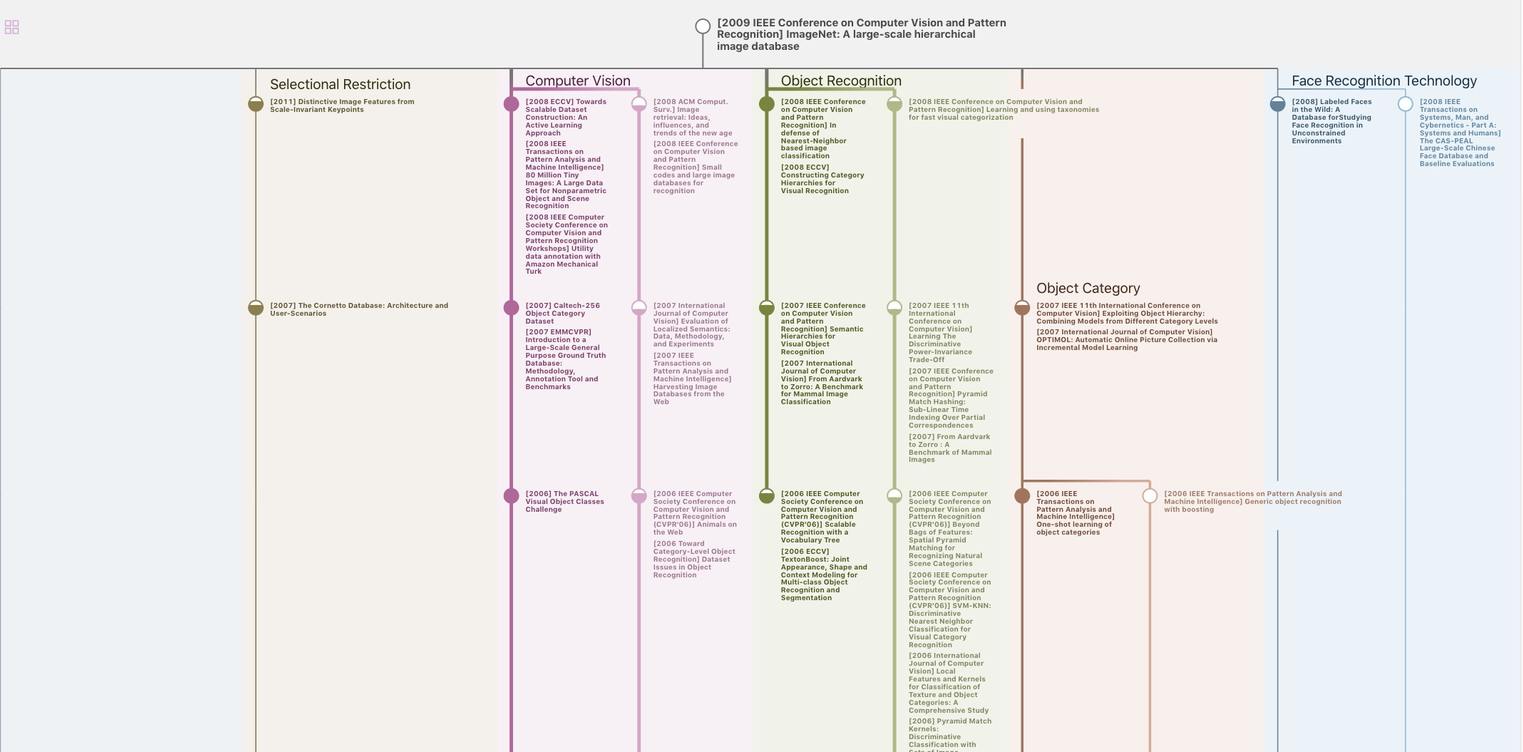
Generate MRT to find the research sequence of this paper
Chat Paper
Summary is being generated by the instructions you defined