TrafficSCINet: An Adaptive Spatial-Temporal Graph Convolutional Network for Traffic Flow Forecasting.
ICIC (1)(2023)
摘要
For complex nonlinear temporal and spatial correlation in traffic flow data, the accurate and effective traffic flow forecasting model is indispensable for understanding the traffic dynamics and predicting the future status of an evolving traffic system. In terms of spatial information extraction, existing approaches are mostly devoted to capture spatial dependency on a predefined graph, which assumes the relation between traffic nodes can be completely offered by an invariant graph structure. However, the fixed graph does not reflect real spatial dependency in traffic data. In this paper, a novel Adaptive Spatial-Temporal Graph Convolutional Network, named as TrafficSCINet, is proposed for traffic flow forecasting. Our model consists of two components: 1) AGCN module uses an adaptive adjacency matrix to dynamically learn the spatial dependencies between traffic nodes under different forecast horizon; 2) SCINet module extracts potential temporal information from traffic flow data through its superb temporal modeling capabilities. Two convolution modules in SCI-Block that have no effect on the results are removed to significantly improve the training speed of the model. Experimental results on four real-world traffic datasets demonstrate that TrafficSCINet achieves state-of-the-art performance consistently than other baselines.
更多查看译文
关键词
flow,network,spatial-temporal
AI 理解论文
溯源树
样例
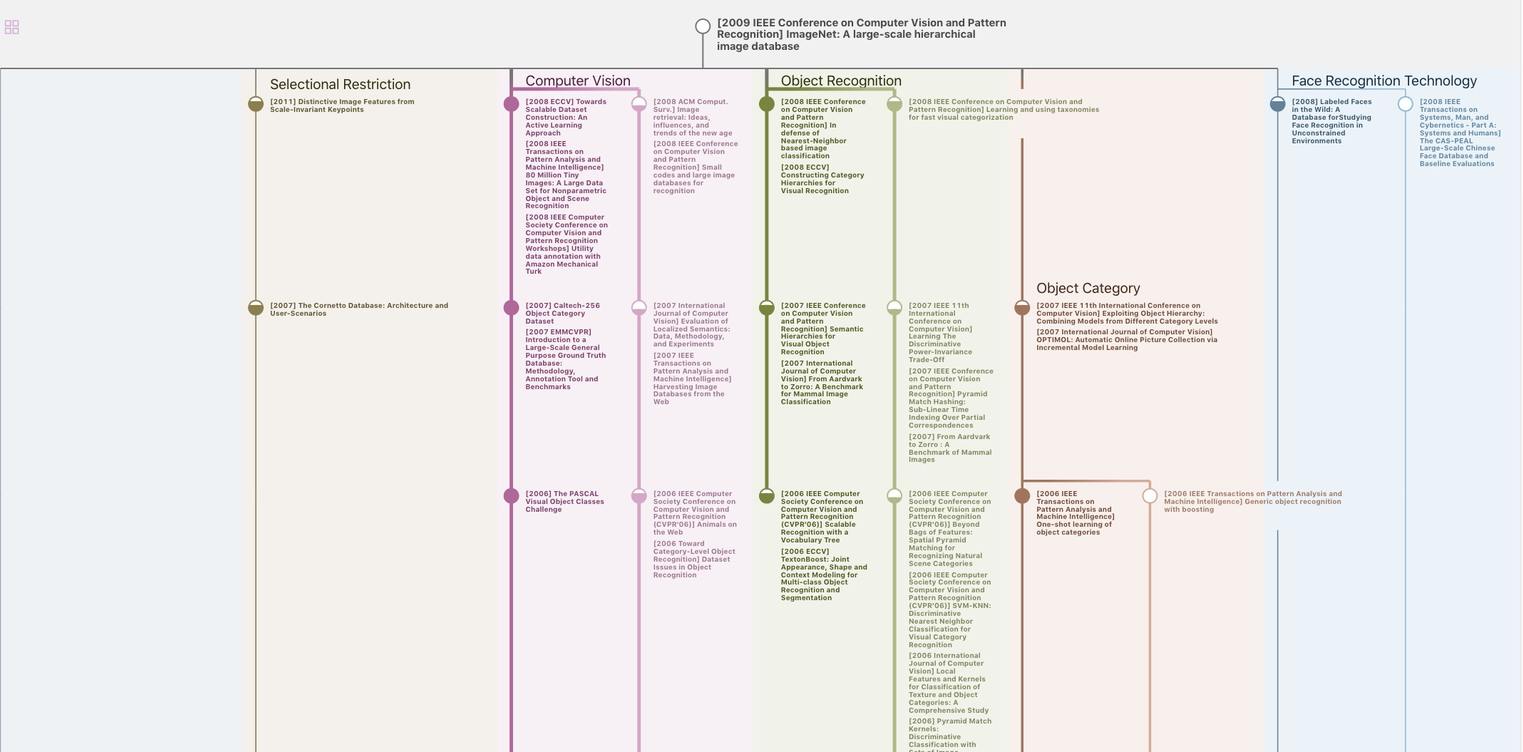
生成溯源树,研究论文发展脉络
Chat Paper
正在生成论文摘要