RA-KD: Random Attention Map Projection for Knowledge Distillation.
ICIC (4)(2023)
摘要
Pretrained language models such as BERT, ELMO, and GPT have proven to be effective for natural language processing tasks. However, deploying them on computationally constrained devices poses a challenge. Moreover, the practical application of these models is affected by the training and deployment of large-scale pretrained language models. While knowledge distillation (KD) in the intermediate layer can improve standard KD techniques, especially for large-scale pretrained language models, distillation in the intermediate layers brings excessive computational burdens and engineering difficulties in mapping the middle layer of a student model with a variable number of layers. The attention map is one of the essential blocks in the intermediate layer. To address these problems, we propose an approach called random attention map projection, in which the intermediate layers are randomly selected from the teacher model. Then, the attention map knowledge is extracted to the student model's attention block. This approach enables the student model to capture deeper semantic information while reducing the computational cost of the intermediate layer distillation method. We conducted experiments on the GLUE dataset to verify the effectiveness of our approach. Our proposed RA-KD approach performs considerably better than other KD approaches in both performance and training time.
更多查看译文
关键词
random attention map projection,knowledge distillation
AI 理解论文
溯源树
样例
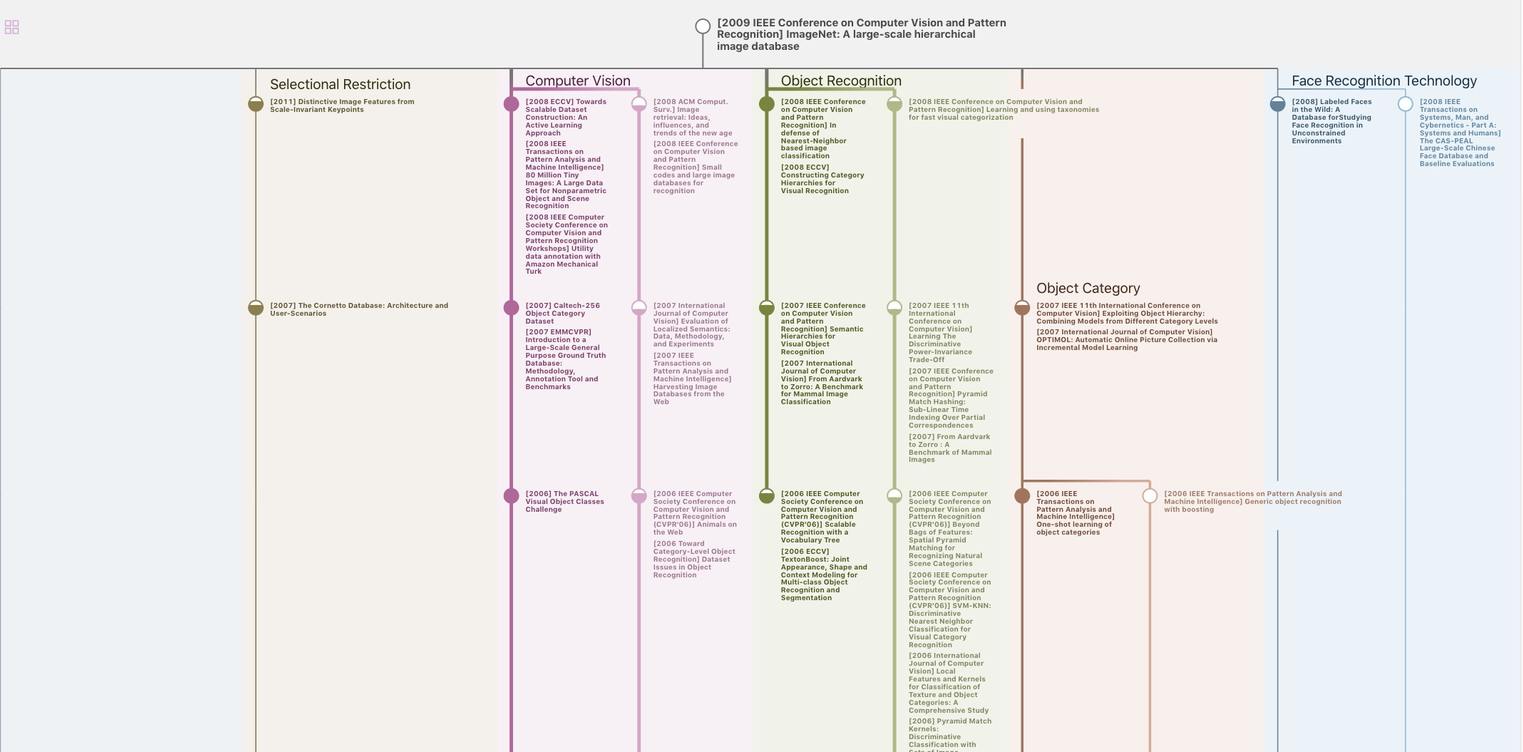
生成溯源树,研究论文发展脉络
Chat Paper
正在生成论文摘要