Performance Analysis and Deep Learning Evaluation of URLLC Full-Duplex Energy Harvesting IoT Networks over Nakagami-m Fading Channels.
SSP(2023)
摘要
This paper studies full-duplex (FD) energy-harvesting Internet-of-Things (IoT) networks, where multiple FD IoT devices are deployed to assist short-packet communications between a source and a robot used in automation factories. Taking into account two residual interference models for FD relays, we propose a full relay selection (FRS) scheme that maximizes the end-to-end signal-to-noise ratio of packet transmissions aiming at improving the block error rate (BLER) and system throughput. Towards real-time settings, we design a deep learning framework based on the FRS scheme to accurately predict the average BLER and throughput via a short inference process. Simulation results show the significant effects of RSI models on the performance of FD IoT networks. Importantly, the DL framework can estimate similar BLER and throughput values as the FRS scheme, but with significantly reduced complexity and execution time, showing the potential of DL design in dealing with complex scenarios of heterogeneous IoT networks.
更多查看译文
关键词
Deep neural network,residual self-interference,short-packet communication,and wireless power transfer
AI 理解论文
溯源树
样例
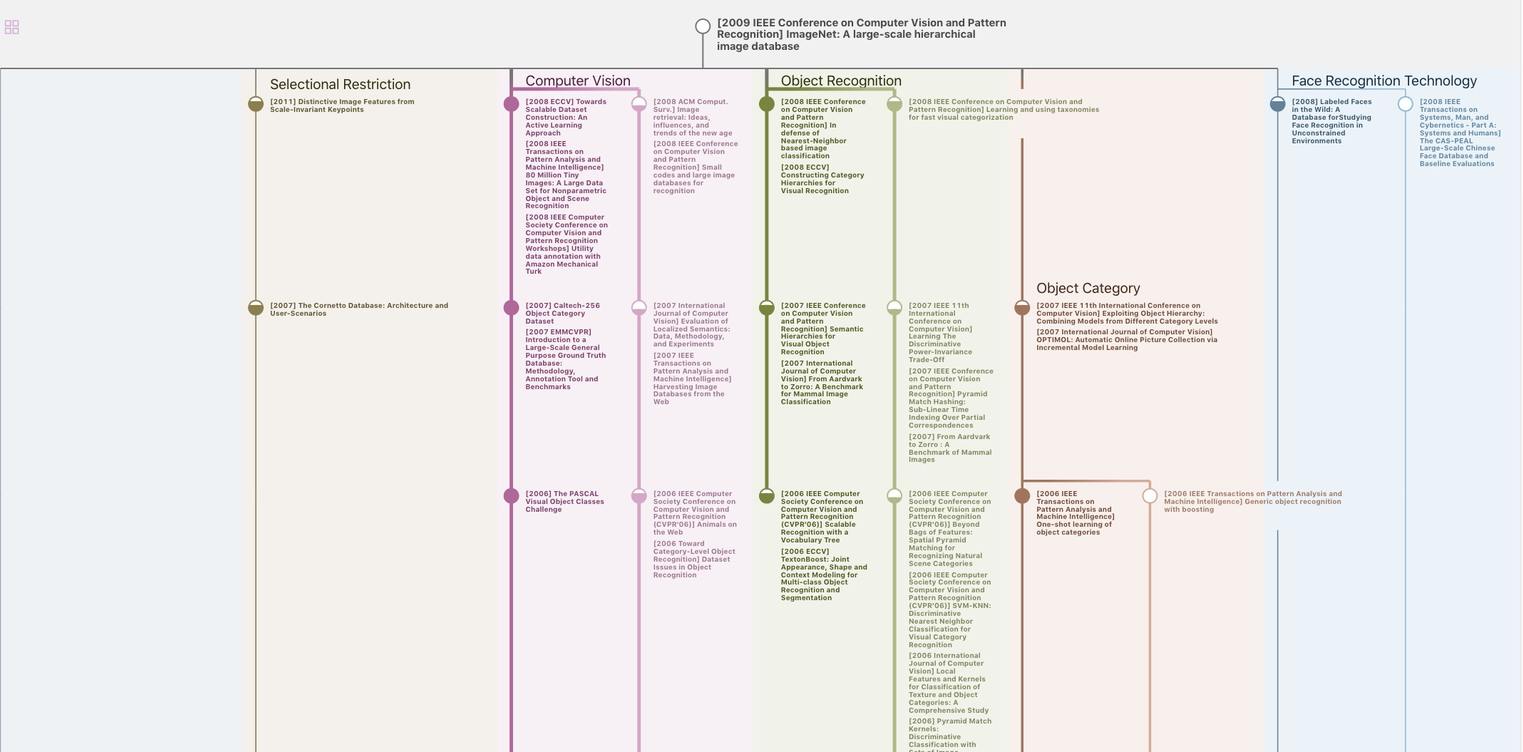
生成溯源树,研究论文发展脉络
Chat Paper
正在生成论文摘要