Bayesian Compressed Sensing-Based Hybrid Models for Stock Price Forecasting
SSP(2023)
摘要
Nowadays, conventional statistical approaches to stock price forecasting fail to provide accurate predictions because financial data are affected by noise from different sources. To deal with this issue, we propose to apply Bayesian compressed sensing (BCS) for noise removal before performing any prediction. This results in a hybrid forecasting model combining BCS, denoising, and a prediction technique. The BCS approach was chosen instead of the traditional compressed sensing (CS) due to its superiority in terms of signal recovery accuracy. In the prediction step, we consider three models namely, autoregressive integrated moving average (ARIMA), long short-term memory (LSTM), and forward neural networks (FNN). The Standard & Poor 500 index (SP500), the Hang Seng index (HSI), and the Euro Stock 50 index (EU50) series are used as sample data for validation. In terms of accuracy, numerical results show that the proposed BCS-based hybrid models provide better performance compared to their single counterparts.
更多查看译文
关键词
Bayesian compressed sensing,Stock price forecasting,Neural networks,LSTM,FNN,ARIMA,Denoising
AI 理解论文
溯源树
样例
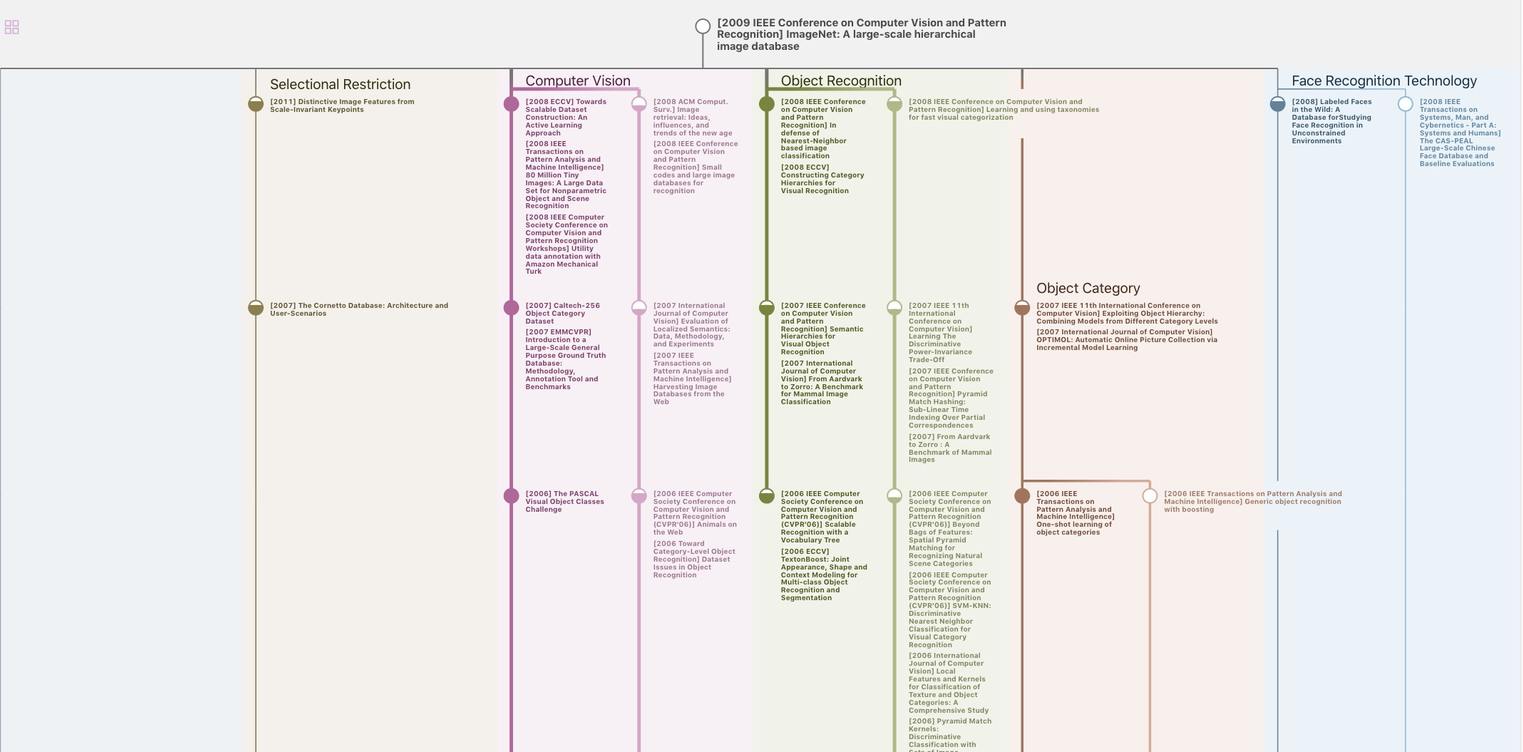
生成溯源树,研究论文发展脉络
Chat Paper
正在生成论文摘要