A Case-Based Reasoning Approach to Company Sector Classification Using a Novel Time-Series Case Representation.
ICCBR(2023)
摘要
The financial domain has proven to be a fertile source of challenging machine learning problems across a variety of tasks including prediction, clustering, and classification. Researchers can access an abundance of time-series data and even modest performance improvements can be translated into significant additional value. In this work, we consider the use of case-based reasoning for an important task in this domain, by using historical stock returns time-series data for industry sector classification. We discuss why time-series data can present some significant representational challenges for conventional case-based reasoning approaches, and in response, we propose a novel representation based on the factorization of a similarity count matrix, which can be readily calculated from raw stock returns data. We argue that this representation is well suited to case-based reasoning and evaluate our approach using a large-scale public dataset for the industry sector classification task, demonstrating substantial performance improvements over several baselines using more conventional representations.
更多查看译文
关键词
company sector classification,reasoning approach,time-series time-series,case-based
AI 理解论文
溯源树
样例
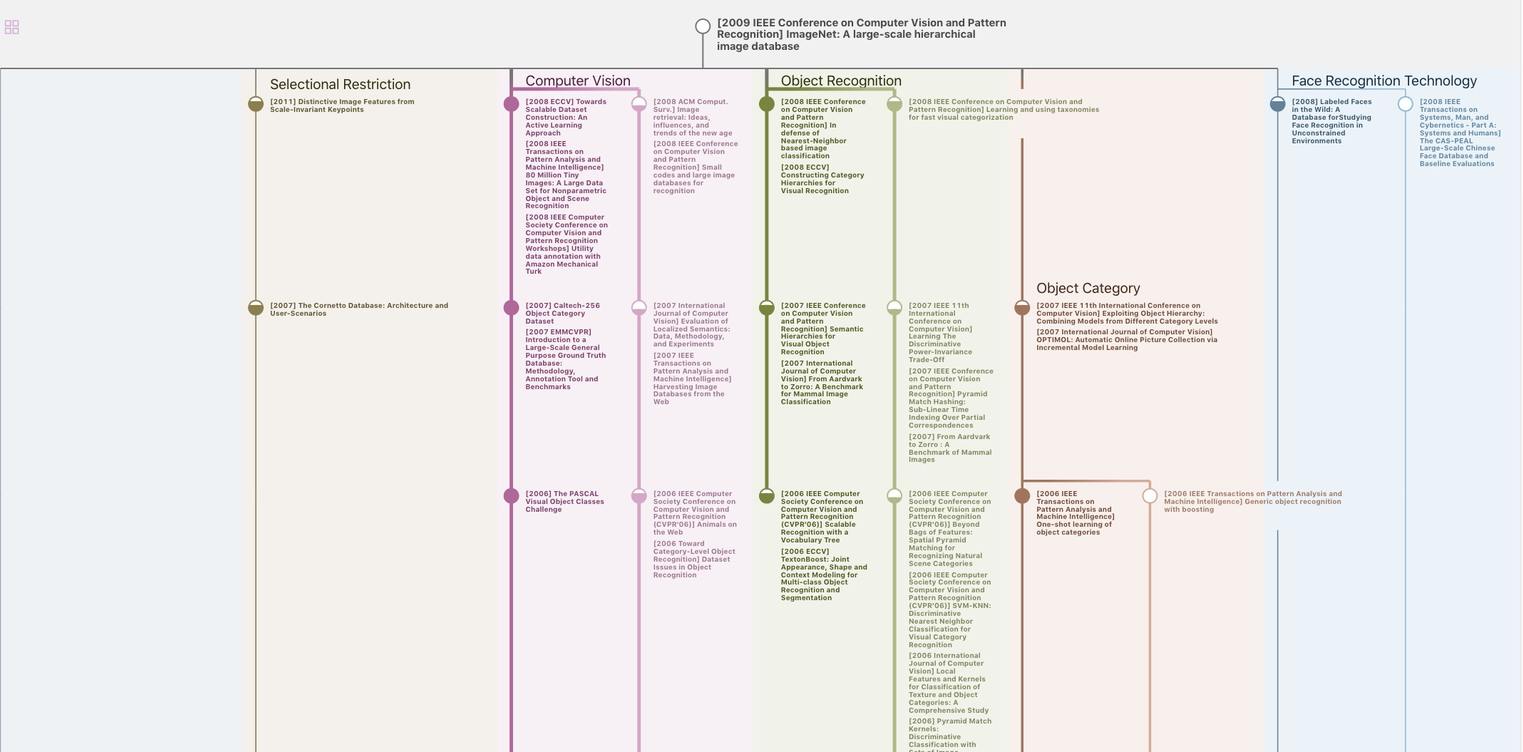
生成溯源树,研究论文发展脉络
Chat Paper
正在生成论文摘要