TraceArk: Towards Actionable Performance Anomaly Alerting for Online Service Systems.
ICSE-SEIP(2023)
摘要
Performance anomaly alerting based on trace data plays an important role in assuring the quality of online service systems. However, engineers find that many anomalies reported by existing techniques are not of interest for them to take further actions. For a large scale online service with hundreds of different microservices, current methods either fire lots of false alarms by applying simple thresholds to temporal metrics (i.e., latency), or run complex end-to-end deep learning model with limited interpretability. Engineers often feel difficult to understand why anomalies are reported, which hinders the followup actions. In this paper, we propose an actionable anomaly alerting approach TraceArk. More specifically, we design an anomaly evaluation model by extracting service impact related anomalous features. A small amount of engineer experience (i.e., feedback) is also incorporated to learn the actionable anomaly alerting model. Comprehensive experiments on a real dataset of Microsoft Exchange service and an anomaly injection dataset collected from an open-source project demonstrate that TraceArk significantly outperforms the existing state-of-the-art approaches. The improvement in F1 is 50.47% and 20.34% on the two datasets, respectively. Furthermore, TraceArk has been running stably for four months in a real production environment and showing a 2.3x improvement in Precision over the previous approach. TraceArk also provides intrepretable alerting details for engineers to take further actions.
更多查看译文
关键词
Performance Anomaly Alerting,Online Service Systems,Human Feedback
AI 理解论文
溯源树
样例
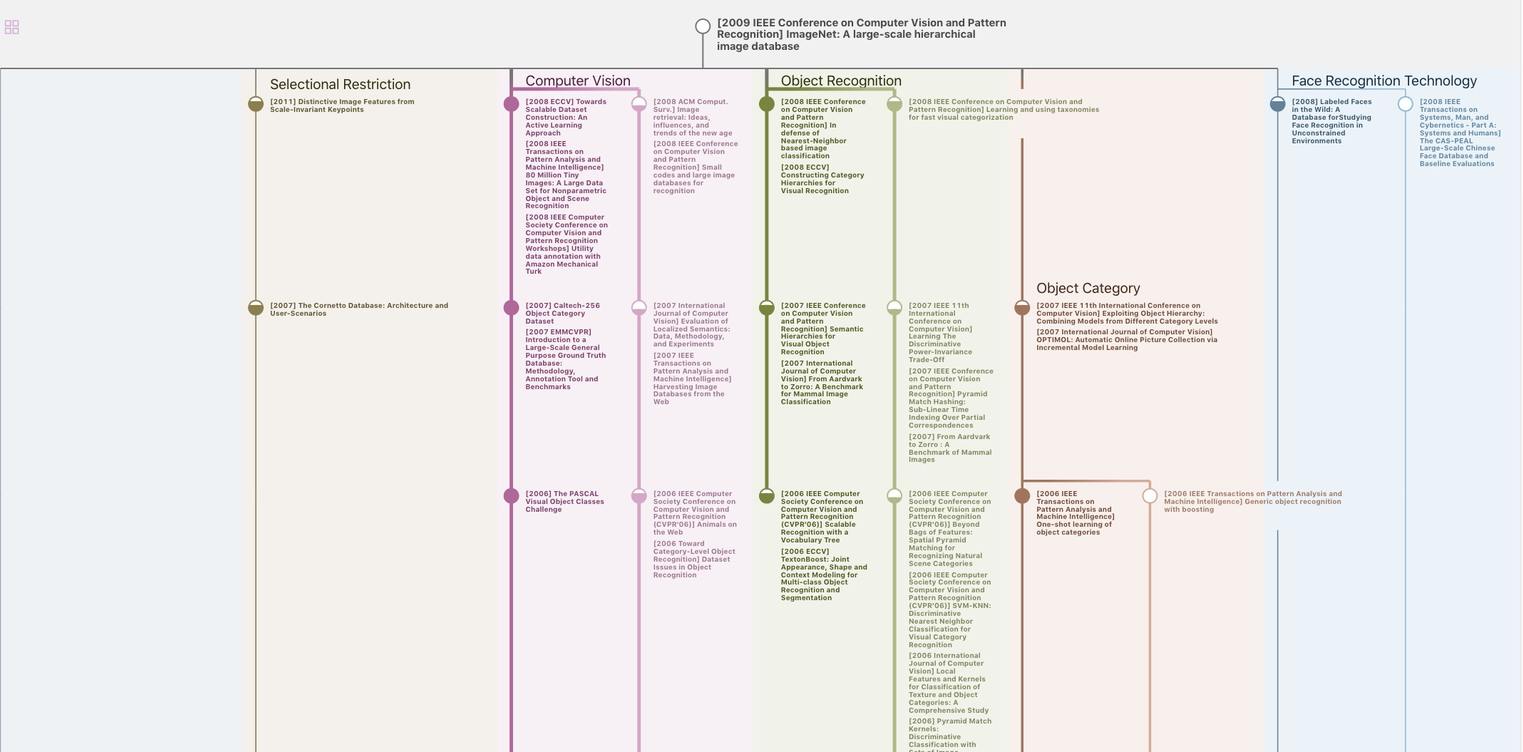
生成溯源树,研究论文发展脉络
Chat Paper
正在生成论文摘要