On the Implications of Heterogeneous Memory Tiering on Spark In-Memory Analytics
IPDPS Workshops(2023)
摘要
Today, the rise of big data has driven a growing demand for efficient and scalable computing solutions that can handle the massive amounts of data generated by modern applications. To address this challenge, application developers have embraced the use of novel distributed frameworks, such as Apache Spark, which enable efficient, in-memory processing for large amounts of data. Moreover, providers are seeking for new alternatives to cope with this increasing need for "infinite" memory resources, that can provide analogous performance efficiency, while also reducing operational costs. In this direction, novel multi-tier and disaggregated memory architectures emerge, which combine heterogeneous memory technologies that tradeoff between performance, cost and energy efficiency. This diptych of evolution imposes new challenges and open questions on how to optimally configure software and hardware as a whole, for maximizing resource efficiency. In this paper, we examine the implications of heterogeneous memory tiering on Spark in-memory analytics. Our study considers a multi-tier heterogeneous DRAM/NVM memory system with contrasting access latency, bandwidth and energy consumption capabilities. By using a set of 7 diverse applications from the HiBench benchmarking suite, we first explore the impact of these memory configuration setups on their performance and energy efficiency. Then, we perform a detailed analysis on how the system's low-level performance metrics correlate to higher level metrics of interest (i.e., performance/energy), which aims to provide deeper insights w.r.t. the relationship between software and hardware events. Driven by the obtained results, we identify a set of guidelines derived by deploying Spark in-memory analytics over heterogeneous memory tiered systems.
更多查看译文
关键词
Disaggregated computing,in-memory analytics,Multi-tier architecture,DRAM,Intel Optane DC
AI 理解论文
溯源树
样例
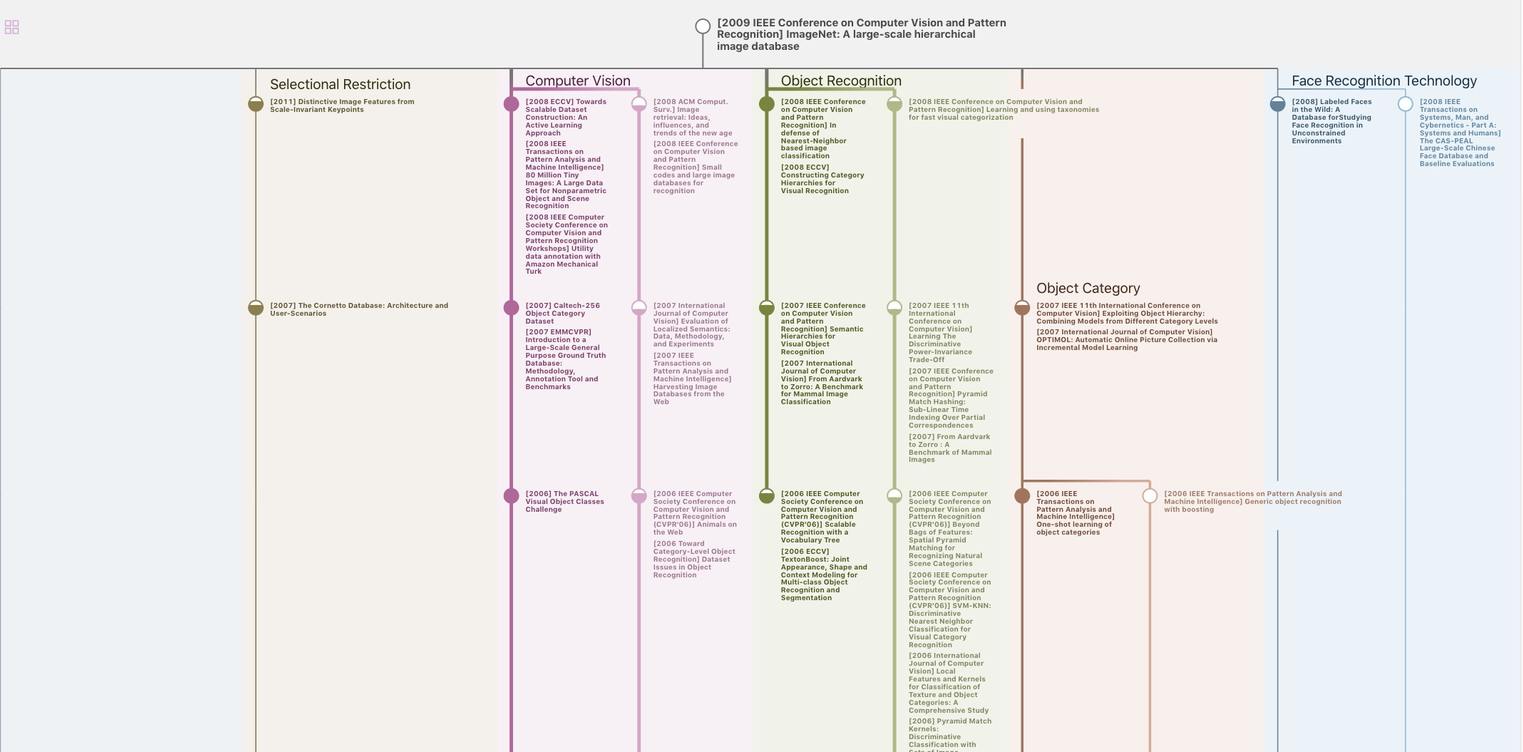
生成溯源树,研究论文发展脉络
Chat Paper
正在生成论文摘要