Human-in-the-Loop Integration with Domain-Knowledge Graphs for Explainable Federated Deep Learning.
CD-MAKE(2023)
摘要
We explore the integration of domain knowledge graphs into Deep Learning for improved interpretability and explainability using Graph Neural Networks (GNNs). Specifically, a protein-protein interaction (PPI) network is masked over a deep neural network for classification, with patient-specific multi-modal genomic features enriched into the PPI graph’s nodes. Subnetworks that are relevant to the classification (referred to as “disease subnetworks”) are detected using explainable AI. Federated learning is enabled by dividing the knowledge graph into relevant subnetworks, constructing an ensemble classifier, and allowing domain experts to analyze and manipulate detected subnetworks using a developed user interface. Furthermore, the human-in-the-loop principle can be applied with the incorporation of experts, interacting through a sophisticated User Interface (UI) driven by Explainable Artificial Intelligence (xAI) methods, changing the datasets to create counterfactual explanations. The adapted datasets could influence the local model’s characteristics and thereby create a federated version that distils their diverse knowledge in a centralized scenario. This work demonstrates the feasibility of the presented strategies, which were originally envisaged in 2021 and most of it has now been materialized into actionable items. In this paper, we report on some lessons learned during this project.
更多查看译文
关键词
deep learning,human-in-the-loop,domain-knowledge
AI 理解论文
溯源树
样例
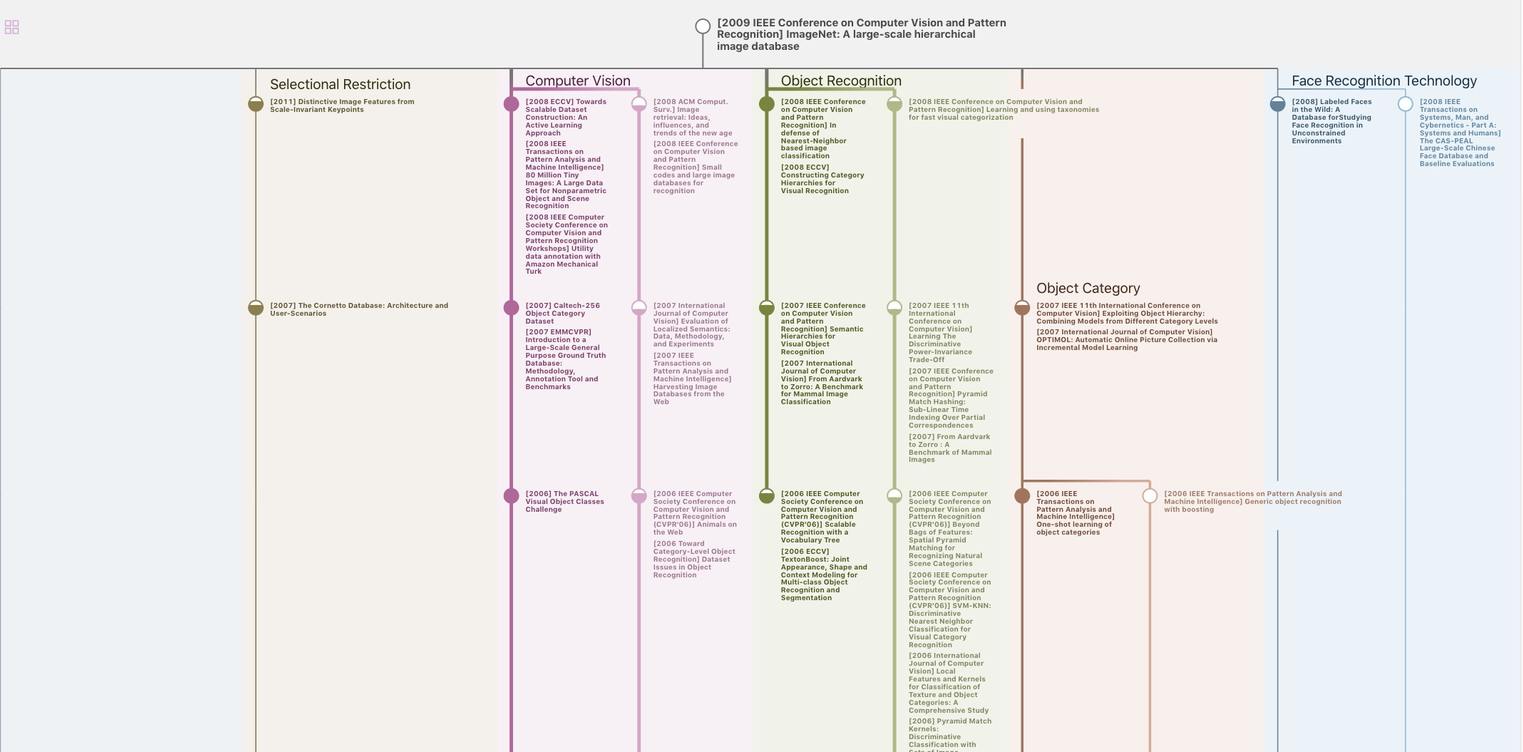
生成溯源树,研究论文发展脉络
Chat Paper
正在生成论文摘要