Experimental Digital Twin of a Tunnel Ring Building Erector.
EUROCON(2023)
摘要
Tunnel building is a hazardous working environment, where operators mainly deal with two processes: tunnel excavation and ring building. Ring building is a crucial task involving the assembly of concrete segments into a tunnel ring using an erector unit to reinforce the freshly bored tunnel surface. The erector unit is a human-controlled robot with six degrees of freedom movement used to grab and place the individual tunnel segments. The experience of the erector operator is hence decisive for an efficient ring building process, which can be optimized with the assistance of intelligent control systems. Reinforcement Learning (RL) is a learning approach that provided many control policies for robotic applications. Currently, no simulation environment is publicly available to train and test RL agents on erector units in tunnel ring building. This research aims to address this gap by designing an Experimental Digital Twin (EDT) for the erector unit in ring building. We created our EDT using the simulation tool MuJoCo and Proximal Policy Optimization as RL method. Our findings reveal that the RL agent learned to successfully build tunnel rings with a control policy that can be further optimized for fine adjustments in segment placing, especially the last ring segment. Our future work consists of investigating the RL agent robustness and studying transfer potential to real-world applications under safety constraints. Our EDT is a first step towards learning control policies that optimally execute segment lining, thus helping human operators build tunnel rings efficiently in a challenging work environment prone to accidents and decreasing interest from future talents.
更多查看译文
关键词
Experimental Digital Twins,Reinforcement Learning,Intelligent Control Systems,Tunnel Boring Machines
AI 理解论文
溯源树
样例
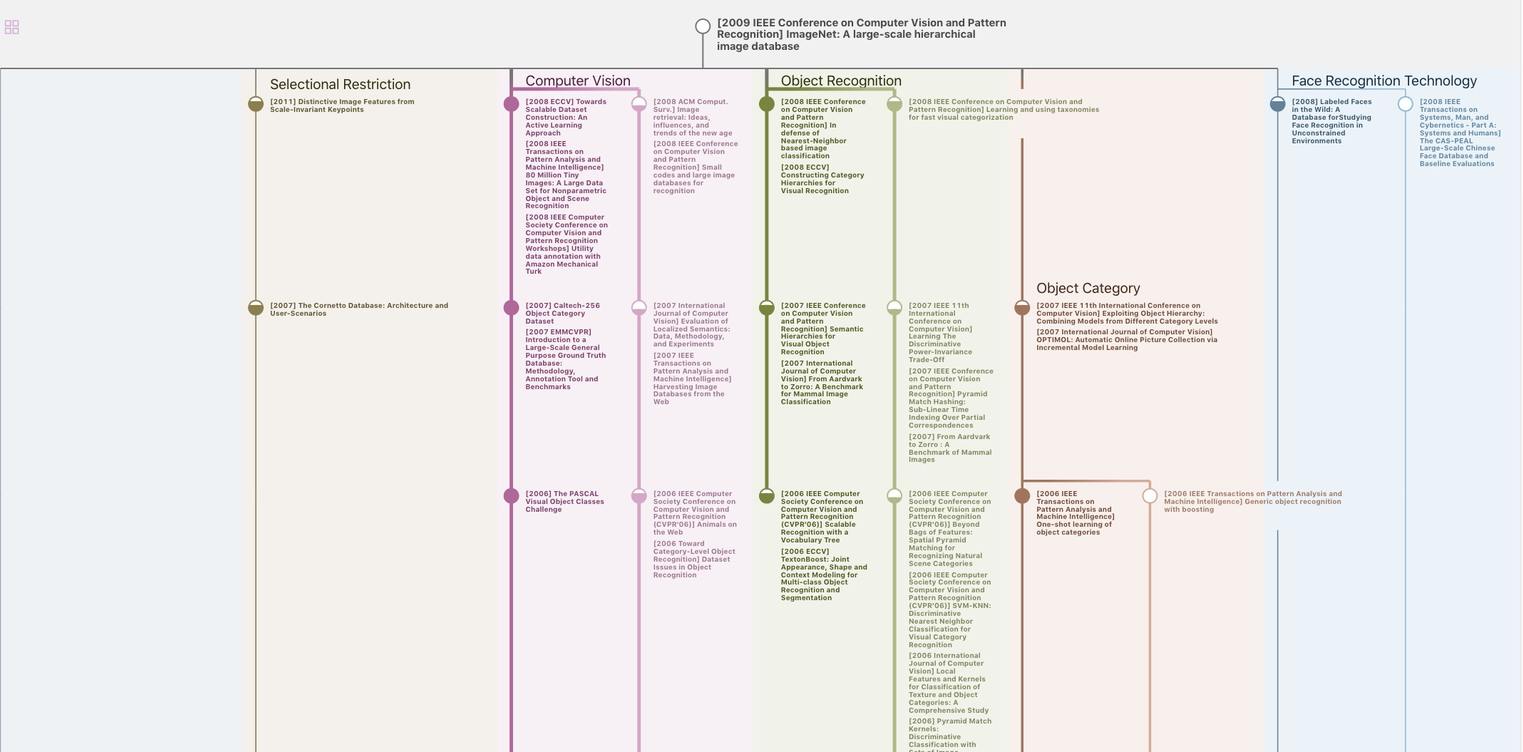
生成溯源树,研究论文发展脉络
Chat Paper
正在生成论文摘要