Towards a Lightweight 2D U-Net for Accurate Semantic Segmentation of Kidney Tumors in Abdominal CT Images.
EUROCON(2023)
Abstract
Accurate segmentation of the kidney anatomy is crucial in the diagnosis and treatment of various kidney diseases. However, 3D U-Net-based Neural Networks entail significant computational requirements, complex architectures, and a fully annotated volumetric dataset. To address these challenges, our study designs and implement a custom image preprocessing workflow that suppresses fat and uninformative structures and compares the performances of 2D U-Net-based Neural Networks for semantic segmentation of kidneys and tumors from abdominal CT images. We found the ResU-Net model to achieve an accuracy of 89.17% for kidney segmentation, outperforming other models, while the Vanilla U-Net during the renal tumor segmentation task, with up to 11.7% higher DSC scores. Moreover, all the investigated methods do not require 3D CNNs, thus reducing computational costs. This comparison could be potentially useful to make a step forward in identifying the most accurate and lightweight technology to aid physicians in diagnosing kidney diseases while improving patient outcomes.
MoreTranslated text
AI Read Science
Must-Reading Tree
Example
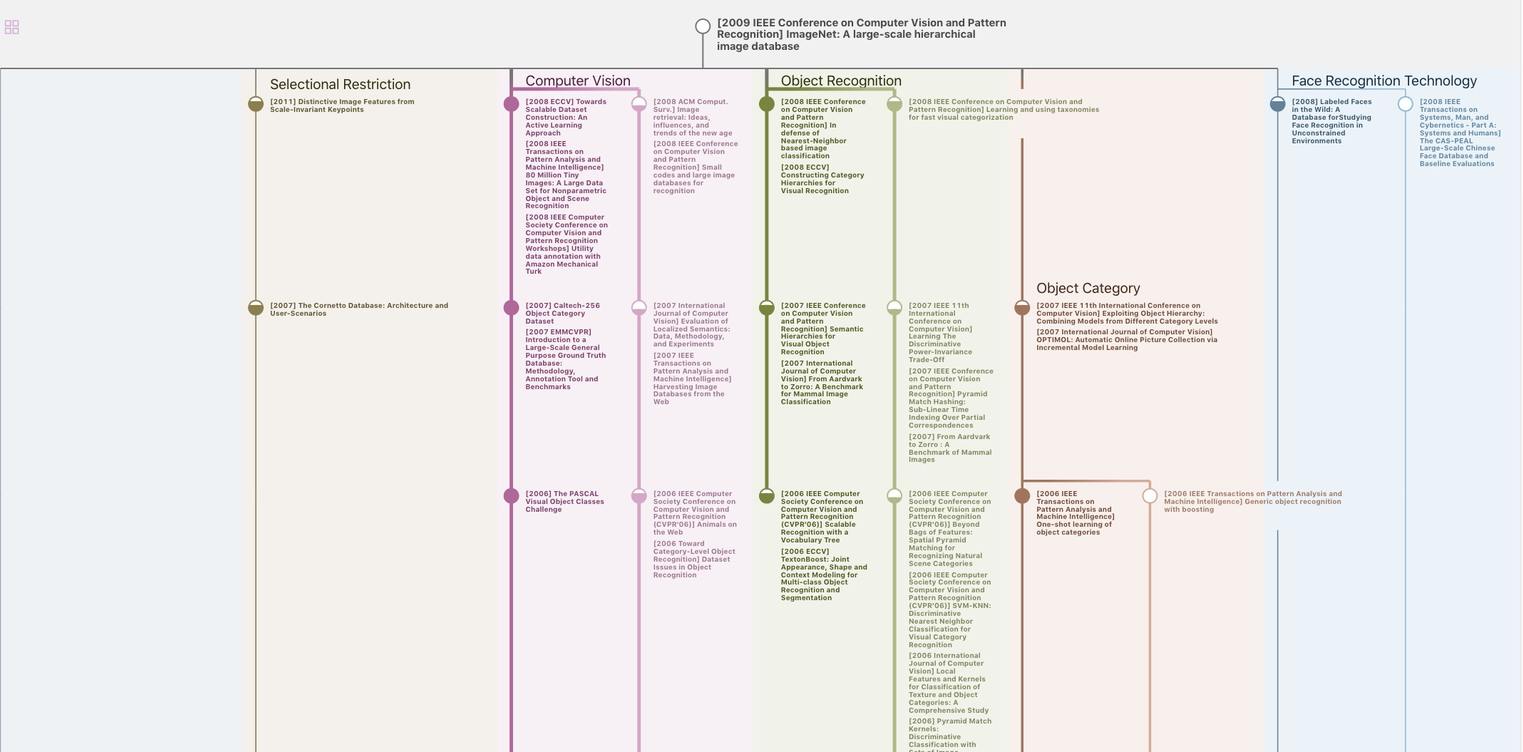
Generate MRT to find the research sequence of this paper
Chat Paper
Summary is being generated by the instructions you defined