MMExit: Enabling Fast and Efficient Multi-modal DNN Inference with Adaptive Network Exits.
Euro-Par(2023)
摘要
Multi-modal DNNs have been demonstrated to outperform the best uni-modal DNNs by fusing information from different modalities. However, the performance improvement of multi-modal DNNs is always associated with an incredible increase in computational cost (e.g., network parameters, MAC operations, etc.) to handle more modalities, which ultimately makes them impractical for many real-world applications where computing capability is limited. In this paper, we propose MMExit, a multi-modal exit architecture that allows for computing appropriate modalities and layers to predict results for different data samples. To this end, we define a novel metric called utility of exit (UoE) to measure the correlations of performance and computational cost for different exits. We then use an equivalent modality serialization method to map the two-dimensional exit space into an equivalent linear space and rank the exits according to their UoE to achieve fast and adaptive inference. To train the MMExit network, we devise a joint loss function which synthesizes the features of different modalities and layers. Our results show that MMExit can slash up to 48.72% of MAC operations with the best performance compared to SOTA multi-modal architectures.
更多查看译文
关键词
adaptive network exits,mmexit,multi-modal
AI 理解论文
溯源树
样例
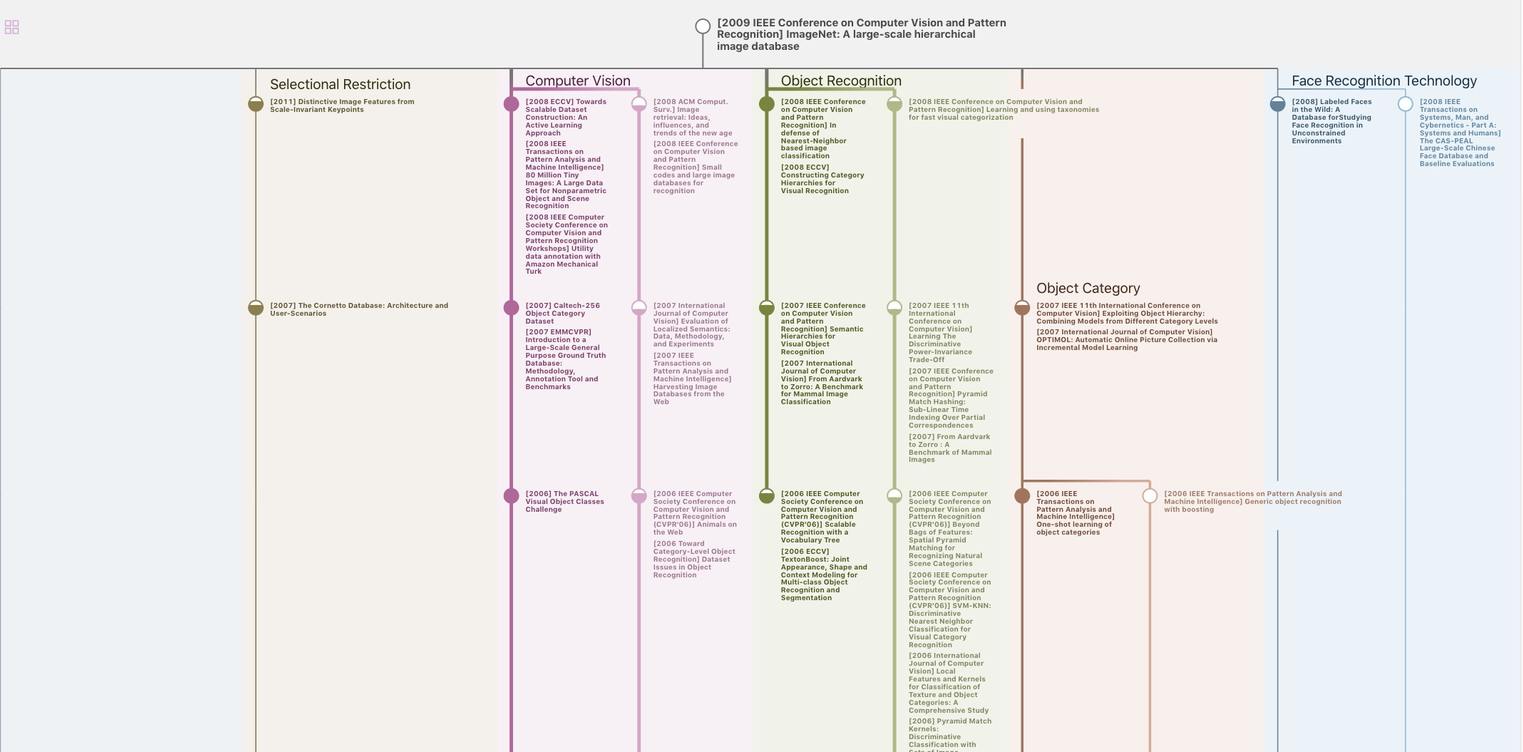
生成溯源树,研究论文发展脉络
Chat Paper
正在生成论文摘要