Stream-Based Active Distillation for Scalable Model Deployment.
CVPR Workshops(2023)
摘要
This paper proposes a scalable technique for developing lightweight yet powerful models for object detection in videos using self-training with knowledge distillation. This approach involves training a compact student model using pseudo-labels generated by a computationally complex but generic teacher model, which can help to reduce the need for massive amounts of data and computational power. However, model-based annotations in large-scale applications may propagate errors or biases. To address these issues, our paper introduces Stream-Based Active Distillation (SBAD) to endow pre-trained students with effective and efficient fine-tuning methods that are robust to teacher imperfections. The proposed pipeline: (i) adapts a pre-trained student model to a specific use case, based on a set of frames whose pseudo-labels are predicted by the teacher, and (ii) selects on-the-fly, along a streamed video, the images that should be considered to fine-tune the student model. Various selection strategies are compared, demonstrating: 1) the effectiveness of implementing distillation with pseudo-labels, and 2) the importance of selecting images for which the pre-trained student detects with a high confidence.
更多查看译文
关键词
compact student model,fine-tuning methods,generic teacher model,knowledge distillation,model-based annotations,object detection,pre-trained student model,pseudolabels,SBAD,scalable model deployment,stream-based active distillation,streamed video
AI 理解论文
溯源树
样例
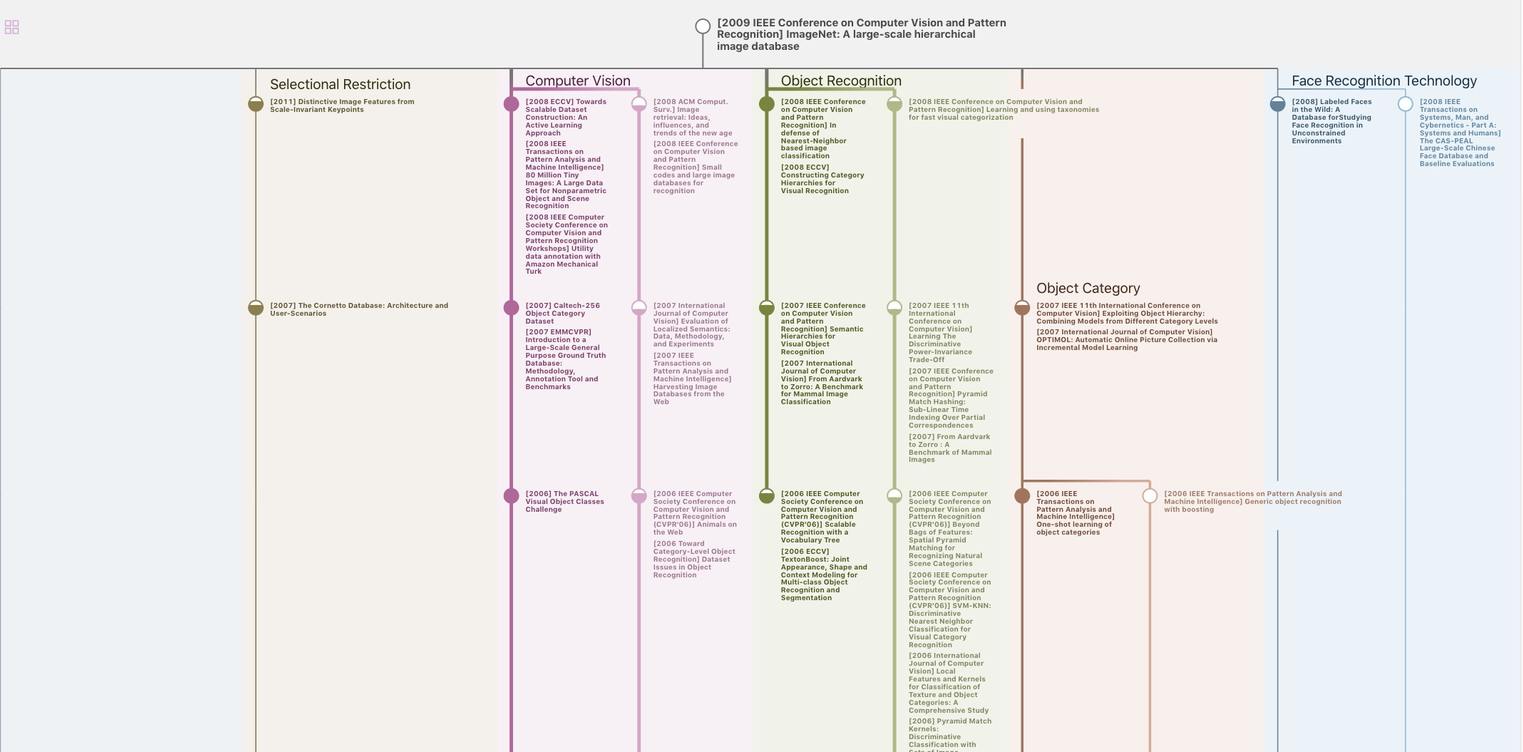
生成溯源树,研究论文发展脉络
Chat Paper
正在生成论文摘要