Accelerated Magnetic Resonance Parameter Mapping With Low-Rank Modeling and Deep Generative Priors.
SSP(2023)
摘要
Magnetic resonance (MR) parameter mapping aims to quantify MR tissue parameter maps that are valuable biomarkers for a range of biomedical applications. However, it involves solving a high-dimensional imaging problem and its practical utility has been limited by long acquisition times. This paper presents a new image reconstruction method for accelerated MR parameter mapping, integrating low-rank modeling with deep generative priors. Specifically, the proposed method employs a low-rank model to capture the strong spatiotemporal correlation of contrast-weighted images in an MR parameter mapping experiment, while representing the spatial subspace of the model using an untrained generative neural network. Here the untrained neural network serves as an effective regularizer for the low-rank and subspace reconstruction. We develop an algorithm based on variable splitting and the alternating direction method of multipliers to solve the resulting optimization problem. We demonstrate the effectiveness of the proposed method in an MR parameter mapping application example.
更多查看译文
关键词
Low-rank model, subspace model, generative model, deep learning, quantitative MRI
AI 理解论文
溯源树
样例
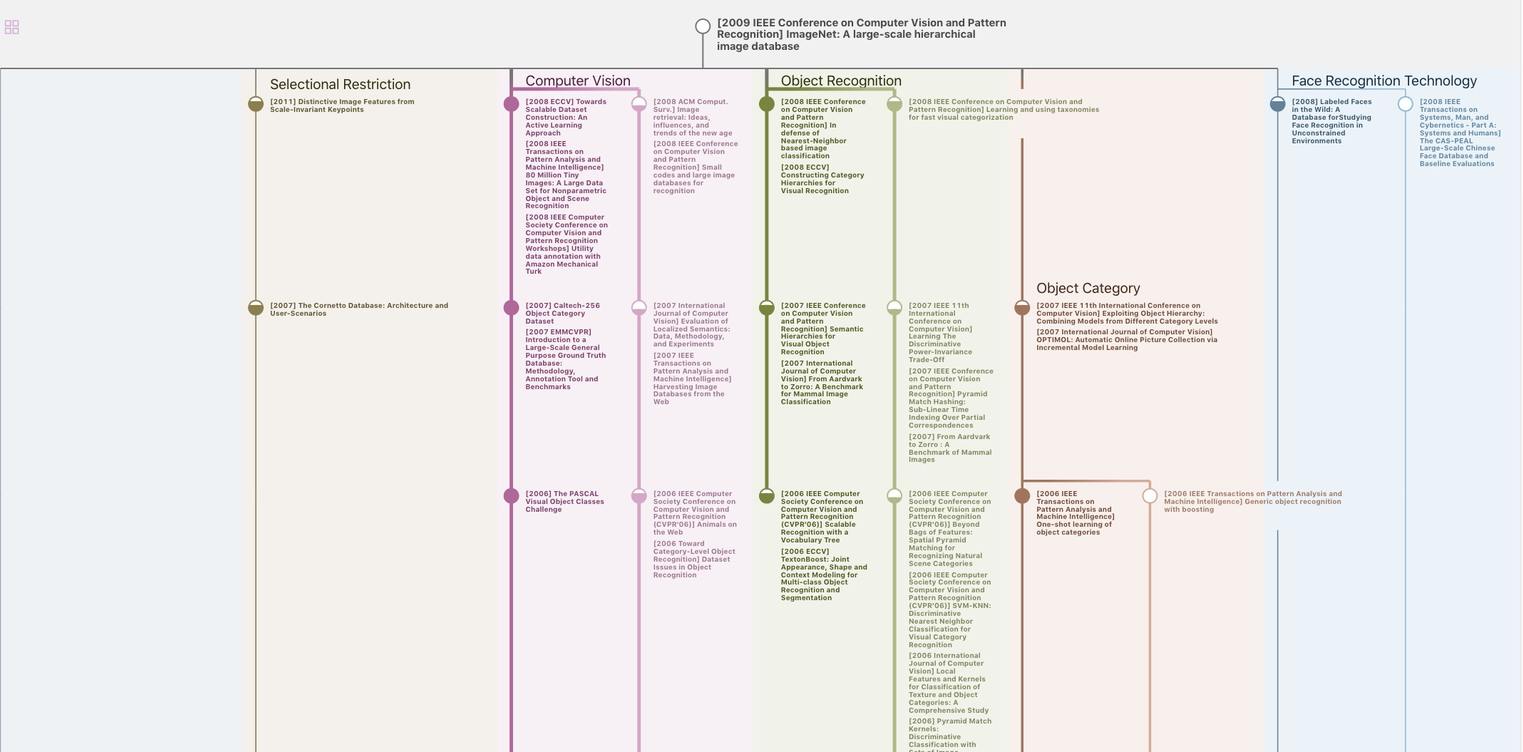
生成溯源树,研究论文发展脉络
Chat Paper
正在生成论文摘要