Digital Twins-based Multi-agent Deep Reinforcement Learning for UAV-assisted Vehicle Edge Computing.
SmartWorld/UIC/ScalCom/DigitalTwin/PriComp/Meta(2022)
Abstract
UAV-assisted vehicle-edge-computing (VEC) has become a viable solution for a new generation of intelligent transportation systems (ITS) and has attracted widespread attention from academia and industry. Compared with fixed ground devices, UAV can provide line-of-sight (LoS) link and has good mobility, which better matches the needs of individual wireless connectivity and high mobility of vehicle. However, the mobility of UAVs leads to dynamic changes in the network topology environment and brings new challenges in the rational path planning of UAVs, which brings new problems for network autonomous decision-making to achieve network resource allocation and load balancing. Therefore, in order to solve above problems, we introduce digital twins-based multi-agent deep Q-network (DT-based MADQN). Digital twin (DT) collects network data and reconstructs the network environment and provides the basis for Deep reinforcement learning (DRL) model training. DRL model provides a network decision-making solution based on real-time network status and empirical data. The simulation results show the effectiveness of the proposed algorithm. Compared to the baseline algorithm, it reduces the average task delay by 16.4% and improves the task completion rate by 97.6%.
MoreTranslated text
Key words
UAV-assisted,Vehicle Edge Computing,Multi Agent Deep Q-network,Digital Twin
AI Read Science
Must-Reading Tree
Example
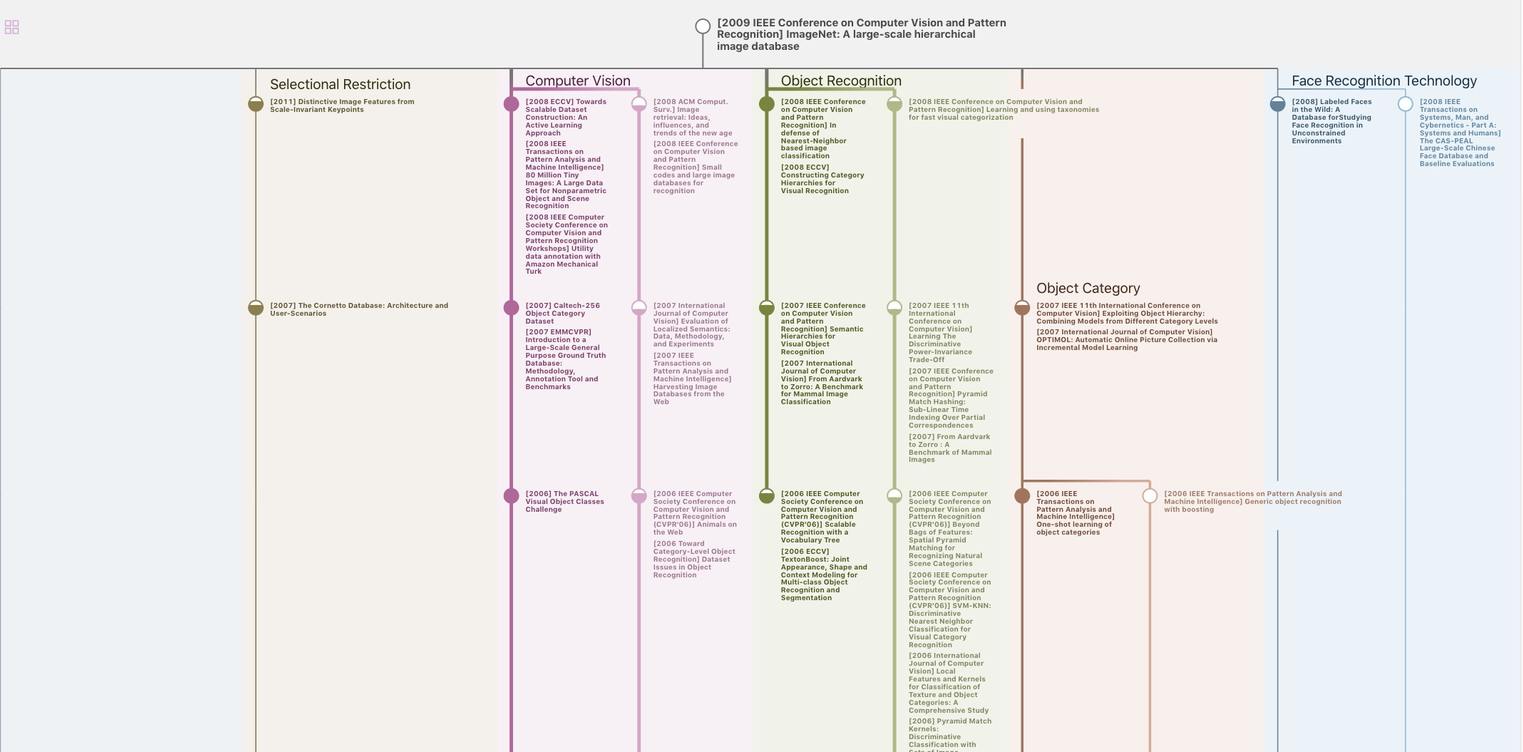
Generate MRT to find the research sequence of this paper
Chat Paper
Summary is being generated by the instructions you defined