SiamDSA: Dual-Branch Self-Attention Siamese Network for Visual Object Tracking
SmartWorld/UIC/ScalCom/DigitalTwin/PriComp/Meta(2022)
摘要
In recent years, object tracking is studied and applied widely in human activity recognition. In object tracking scenarios, the object appearance changes significantly due to various factors, such as scale changes and deformation, which will drastically affect the robustness of the object tracking. To deal with the above issues, we propose a DSA module that integrates the siamese tracking network to implement the siamese network tracking methods based on the dual-branch self-attention (SiamDSA). It fuses object features of different scales to greatly enhance the target information and establish a better target appearance model for improving object tracking robustness. Specifically, a novel DSA module based on self-attention mechanism boosts the feature representation of objects from channel and spatial perspectives. And it is responsible for better focusing on the features of the target. We conduct experiments on GOT-IOK, OTB100 and VOT2018 datasets. The experimental results demonstrate that SiamDSA achieves superior performances and runs at 65 FPS, it further shows strong effectiveness and efficiency in object tracking.
更多查看译文
关键词
object tracking,computer vision,self-attention mechanism
AI 理解论文
溯源树
样例
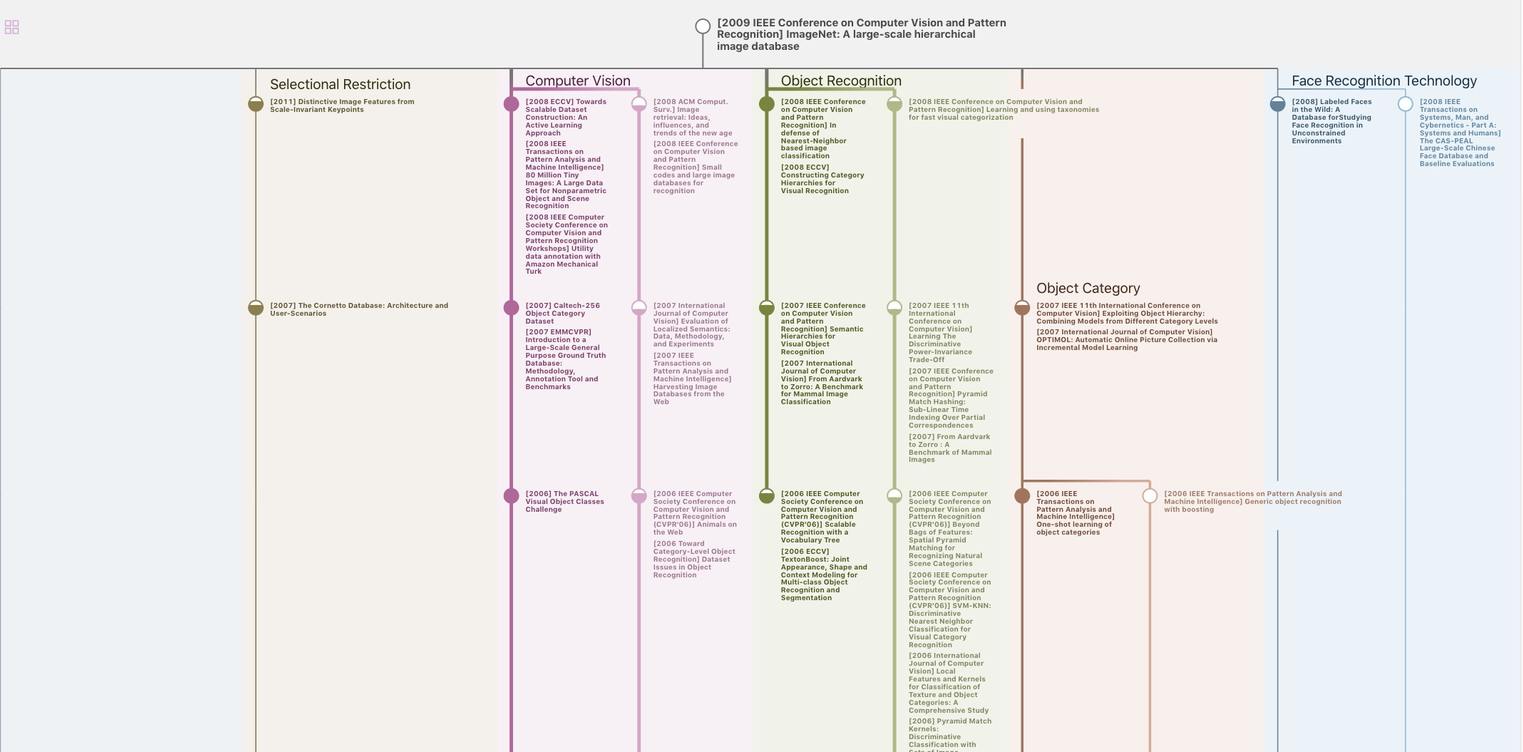
生成溯源树,研究论文发展脉络
Chat Paper
正在生成论文摘要