Fusion-SUNet: Spatial Layout Consistency for 3D Semantic Segmentation.
CVPR Workshops(2023)
摘要
The paper discusses the need for a reliable and efficient computer vision system to inspect utility networks with minimal human involvement, due to the aging infrastructure of these networks. We propose a deep learning technique, Fusion-Semantic Utility Network (Fusion-SUNet), to classify the dense and irregular point clouds obtained from the airborne laser terrain mapping (ALTM) system used for data collection. The proposed network combines two networks to achieve voxel-based semantic segmentation of the point clouds at multi-resolution with object categories in three dimensions and predict two-dimensional regional labels distinguishing corridor regions from non-corridors. The network imposes spatial layout consistency on the features of the voxel-based 3D network using regional segmentation features. The authors demonstrate the effectiveness of the proposed technique by testing it on 67km
2
of utility corridor data with average density of 5pp/m
2
, achieving significantly better performance compared to the state-of-the-art baseline network, with an F1 score of 93% for pylon class, 99% for ground class, 99% for vegetation class, and 98% for powerline class.
更多查看译文
关键词
3D Semantic segmentation,airborne laser terrain mapping system,corridor regions,deep learning technique,dense point clouds,efficient computer vision system,Fusion-Semantic Utility Network,Fusion-SUNet,irregular point clouds,minimal human involvement,regional segmentation features,reliable computer vision system,spatial layout consistency,state-of-the-art baseline network,utility corridor data,utility networks,voxel-based 3D,voxel-based semantic segmentation
AI 理解论文
溯源树
样例
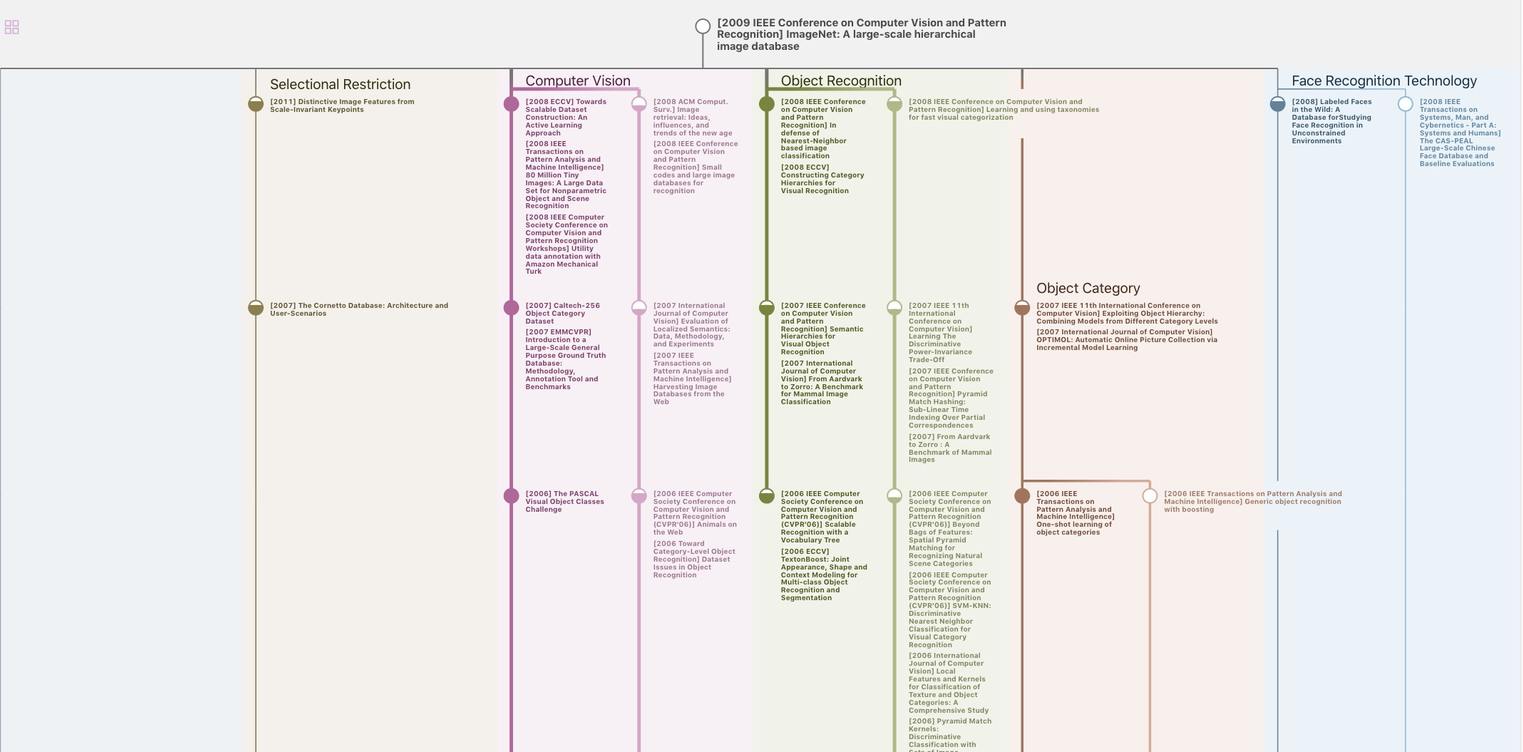
生成溯源树,研究论文发展脉络
Chat Paper
正在生成论文摘要