Abstract Visual Reasoning Enabled by Language.
CVPR Workshops(2023)
Abstract
While artificial intelligence (AI) models have achieved human or even superhuman performance in many well-defined applications, they still struggle to show signs of broad and flexible intelligence. The Abstraction and Reasoning Corpus (ARC), a visual intelligence benchmark introduced by François Chollet, aims to assess how close AI systems are to human-like cognitive abilities. Most current approaches rely on carefully handcrafted domain-specific program searches to brute-force solutions for the tasks present in ARC. In this work, we propose a general learning-based framework for solving ARC. It is centered on transforming tasks from the vision to the language domain. This composition of language and vision allows for pre-trained models to be leveraged at each stage, enabling a shift from handcrafted priors towards the learned priors of the models. While not yet beating state-of-the-art models on ARC, we demonstrate the potential of our approach, for instance, by solving some ARC tasks that have not been solved previously.
MoreTranslated text
Key words
abstract visual reasoning,AI systems,ARC tasks,artificial intelligence models,broad intelligence,brute-force solutions,cognitive abilities,flexible intelligence,general learning-based framework,handcrafted domain-specific program searches,language domain,vision domain,visual intelligence benchmark
AI Read Science
Must-Reading Tree
Example
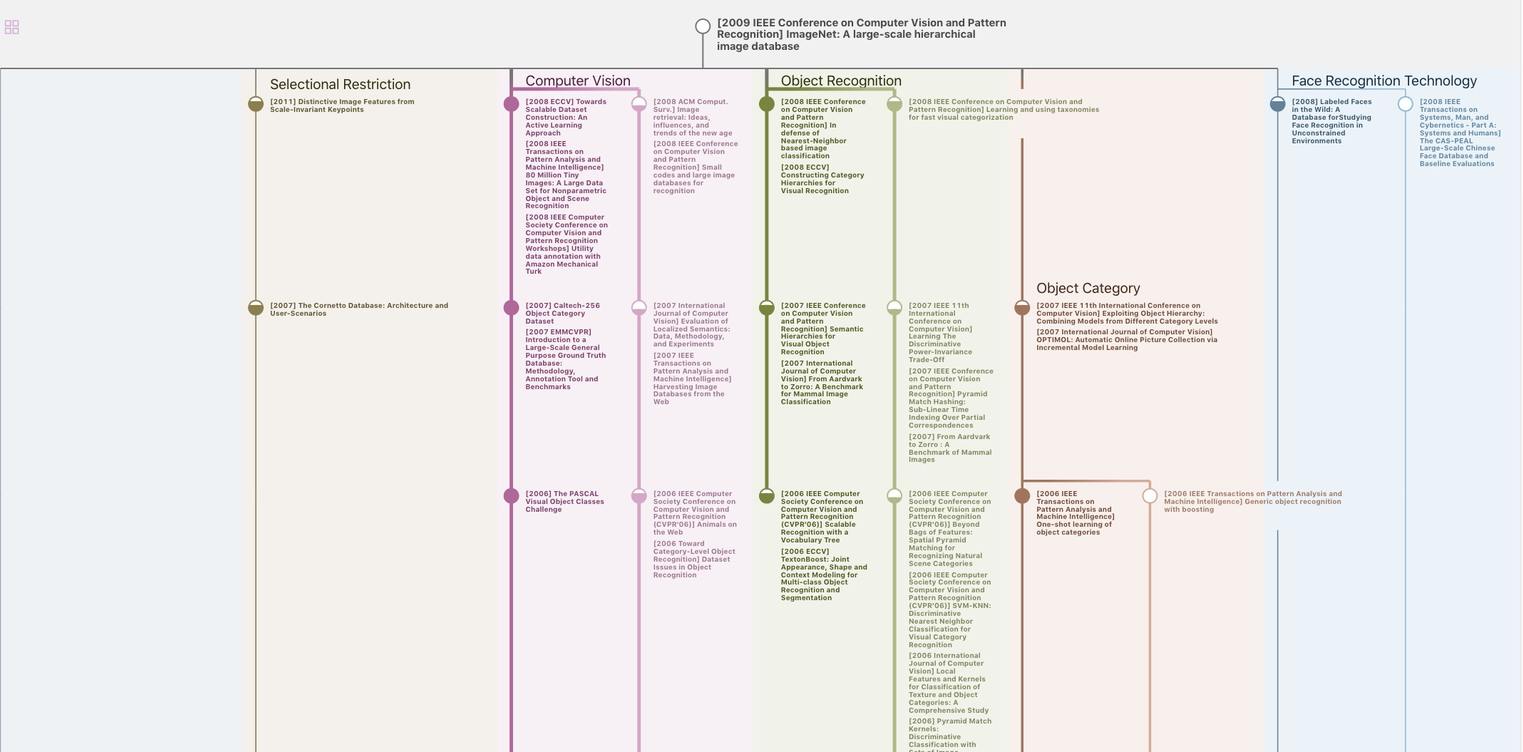
Generate MRT to find the research sequence of this paper
Chat Paper
Summary is being generated by the instructions you defined