Resilient Navigation Based on Multimodal Measurements and Degradation Identification for High-Speed Autonomous Race Cars.
IV(2023)
摘要
This paper presents a localization system robust against unreliable measurements and a resilient navigation system recovering from localization failures for Indy autonomous challenge (IAC). The IAC is a competition with full-scale autonomous race cars that drive at speeds up to 300 kph. Owing to high-speed and heavy vibration in the car, a GPS/INS system is prone to degrade causing critical localization errors, which leads to catastrophic accidents. In order to address this issue, we propose a robust localization system that probabilistically evaluates the credibility of multimodal measurements. At a correction step of the Kalman filter, a degradation identification method with a novel hyper-parameter derived from Bayesian decision theory is introduced to choose the most credible measurement values in real-time. Since the racing condition is so harsh that even our robust localization method can fail for a short period of time, we present a resilient navigation system that enables the race car to continue to follow the race track in the event of a localization failure. Our system uses direct perception information in planning and execution until the completion of localization recovery. The proposed localization system is first validated in a simulation with real measurement data contaminated by large artificial noises. The experimental validation during an actual race is also presented. The last part of our paper shows the results from the real-world tests where our system recovers from failures and prevents accidents in real-time, which proves the resilience of the proposed navigation system.
更多查看译文
关键词
Navigation,Sensors,Multimodal Systems
AI 理解论文
溯源树
样例
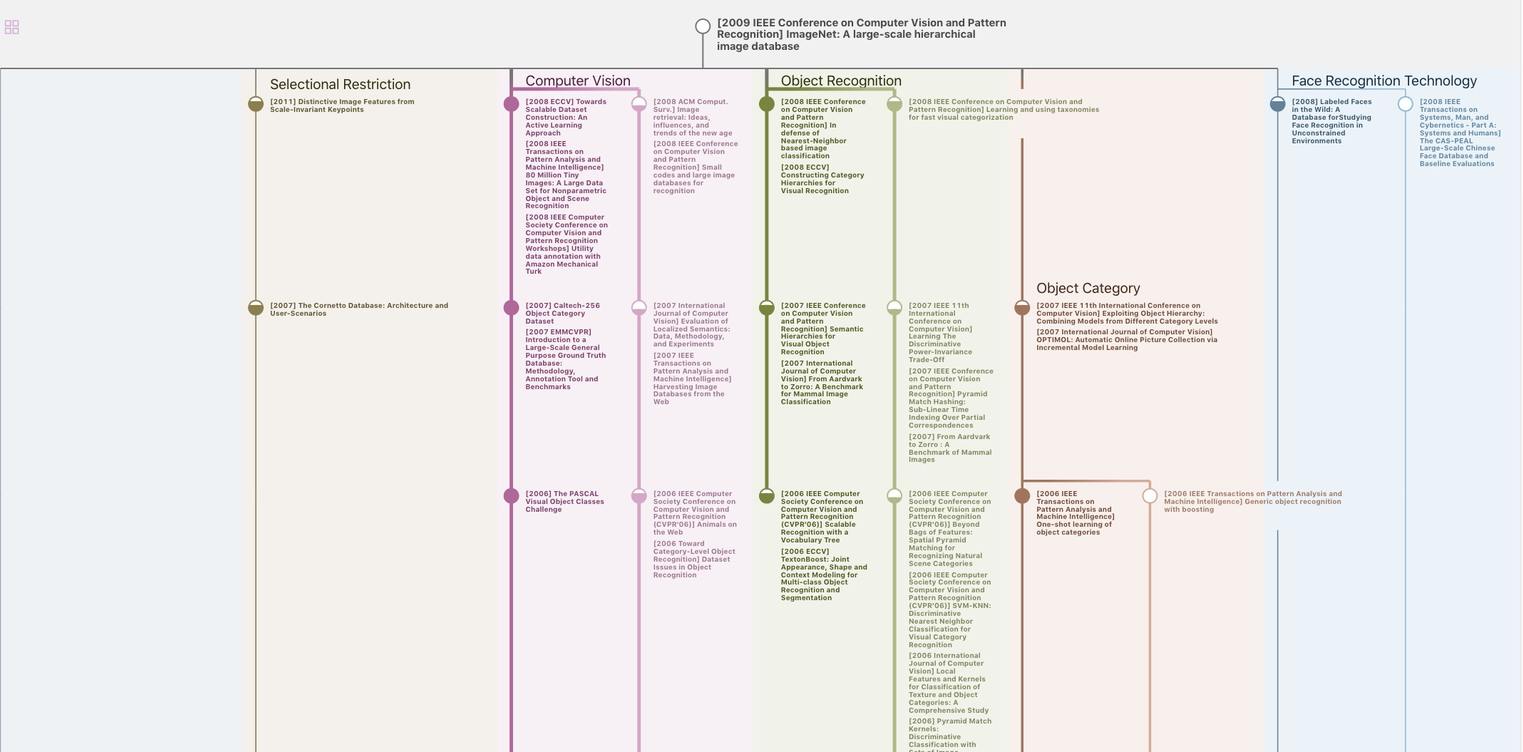
生成溯源树,研究论文发展脉络
Chat Paper
正在生成论文摘要