Enhancing Crane Handling Safety: A Deep Deterministic Policy Gradient Approach to Collision-Free Path Planning
INDIN(2023)
摘要
Technological progress is allowing for a more efficient and safe crane operation, reducing the risks associated with heavy machinery use in construction and logistics industries. To enhance crane operations, this study aims to develop a collision-free path planning model for crane manipulation.To accomplish this, we have created a simulation environment that serves as a digital twin of the physical crane operating environment, employing reinforcement learning (RL) techniques, where the agent learns to improve its performance by interacting with the operating environment. We evaluated two different reward methods for our Deep Deterministic Policy Gradient (DDPG) algorithm: an adapted method and a proposed method. Our results indicate that the proposed reward method yielded superior training performance compared to the adapted method. These results demonstrate the potential benefits of implementing the proposed reward method in crane operations.
更多查看译文
关键词
Reinforcement learning,crane,path
AI 理解论文
溯源树
样例
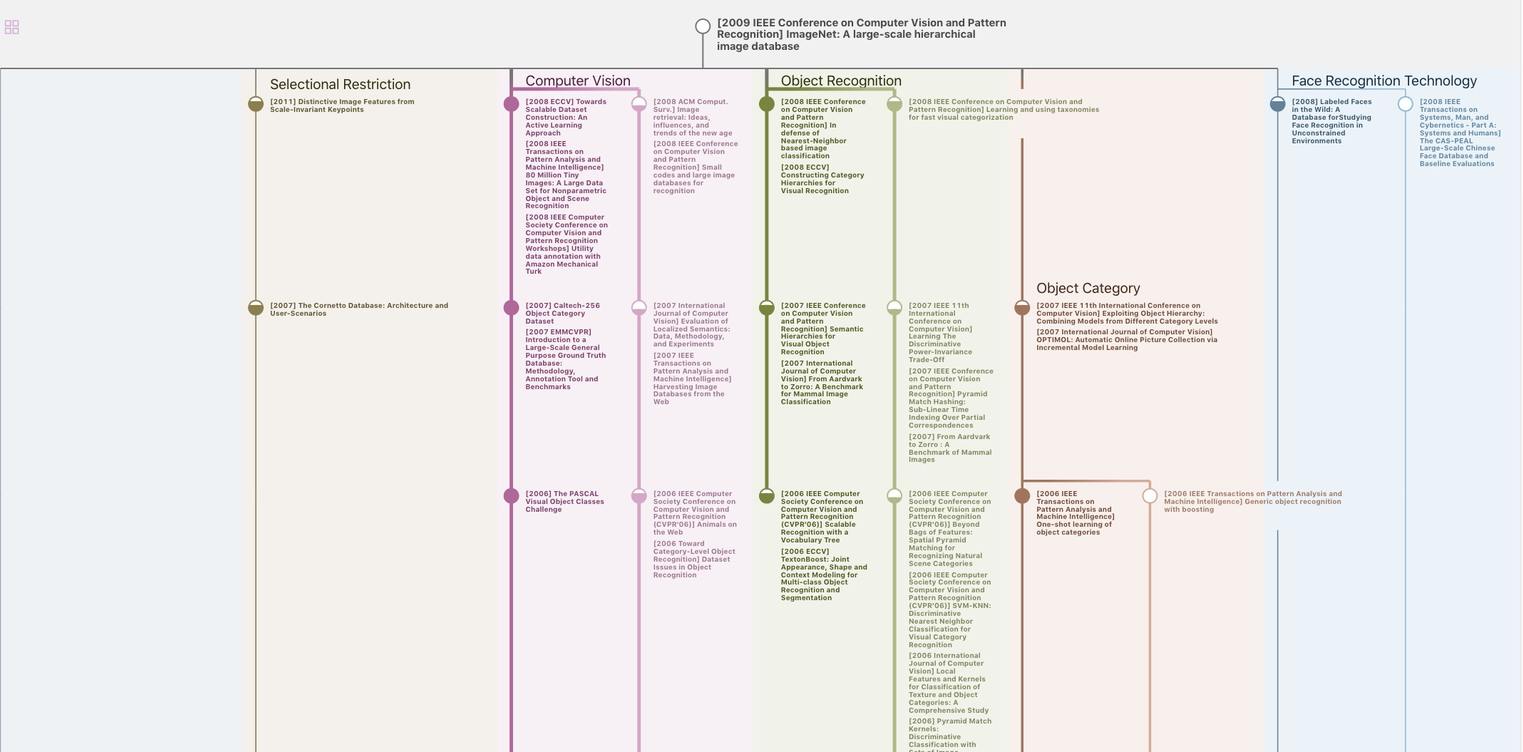
生成溯源树,研究论文发展脉络
Chat Paper
正在生成论文摘要