Automatic Generation of Visual Concept-based Explanations for Pest Recognition.
INDIN(2023)
摘要
Pest management is an important factor affecting agricultural and food industry products. A large number of insect species and the subtle differences bring a challenge to the accurate recognition of pests. Many studies tackle the challenge of pest recognition through deep neural networks (DNNs) and achieve significant success in terms of accuracy. However, the complex structure and a large number of parameters make DNNs difficult for end users to understand the reasons for the decision of models, which causes distrust in the classification of harmful insects and overuse of insecticides. To address the lack of explainability of DNNs, we propose an explanation generation workflow to generate concept-based explanations for pest recognition. Specifically, the concept extraction method uses a clustering algorithm to extract image segments with meaningful concepts from a portion of the training dataset. Then, concept models are trained to detect the presence of concepts in the image. Finally, the explanation generation method provides concept-based global and local explanations in the form of weighted directed graphs and concept importances, respectively. Through qualitative and quantitative analysis, the proposed workflow extracts meaningful concepts for pest recognition effectively and detects the presence of concepts in images.
更多查看译文
关键词
Explainable machine learning,Concept-based explanation,Deep neural networks,Pest recognition
AI 理解论文
溯源树
样例
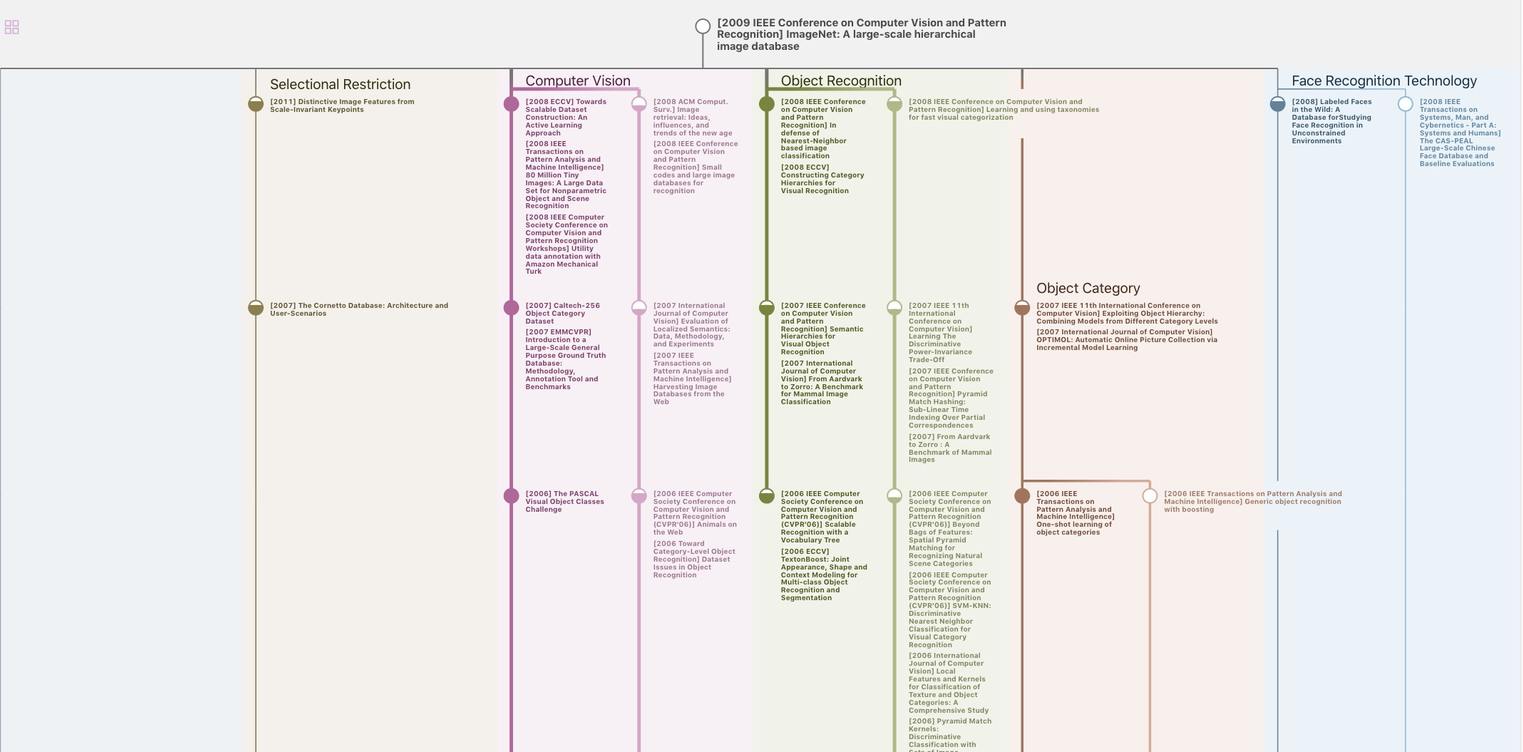
生成溯源树,研究论文发展脉络
Chat Paper
正在生成论文摘要