Anomaly Detection for Hydroelectric Power Plants: a Machine Learning-based Approach.
INDIN(2023)
摘要
Hydroelectric is currently the most prominent among the sources of green energy, but, differently from the other sources, it has very strict requirements in terms of security that are taken into account with extremely robust constraints both at design and operations control times. In this paper, we evaluated the effectiveness of anomaly detection and explainability algorithms to supplement Decision Support System insights in Predictive Maintenance and Root Cause Analysis for hydroelectric power plants. The objective is to reduce operational costs and increase reliability in the plant, making hydroelectric technology more appealing to investors and promoting the transition to renewable energy. Specifically, the performance of several anomaly detection models was compared on real-world data with respect to the needs of the expert of the domain, that is the final user of the DSS, to work as an additional feature to speed up predictive maintenance. Additionally, the impact of SHapley Additive exPlanations values on helping the user understand the anomaly causes was investigated. Our findings are that the most performing algorithm was Auto-Encoder since it was able to find all recorded anomalies and even propose additional ones later confirmed by domain experts. The application of SHAP values was found to effectively guide the user toward the features related to the anomaly, although its application on streaming data was slow.
更多查看译文
关键词
Anomaly Detection,Decision Support System,Hydropower Plants,Interpretability,Machine Learning,Maintenance Management,Root Cause Analysis
AI 理解论文
溯源树
样例
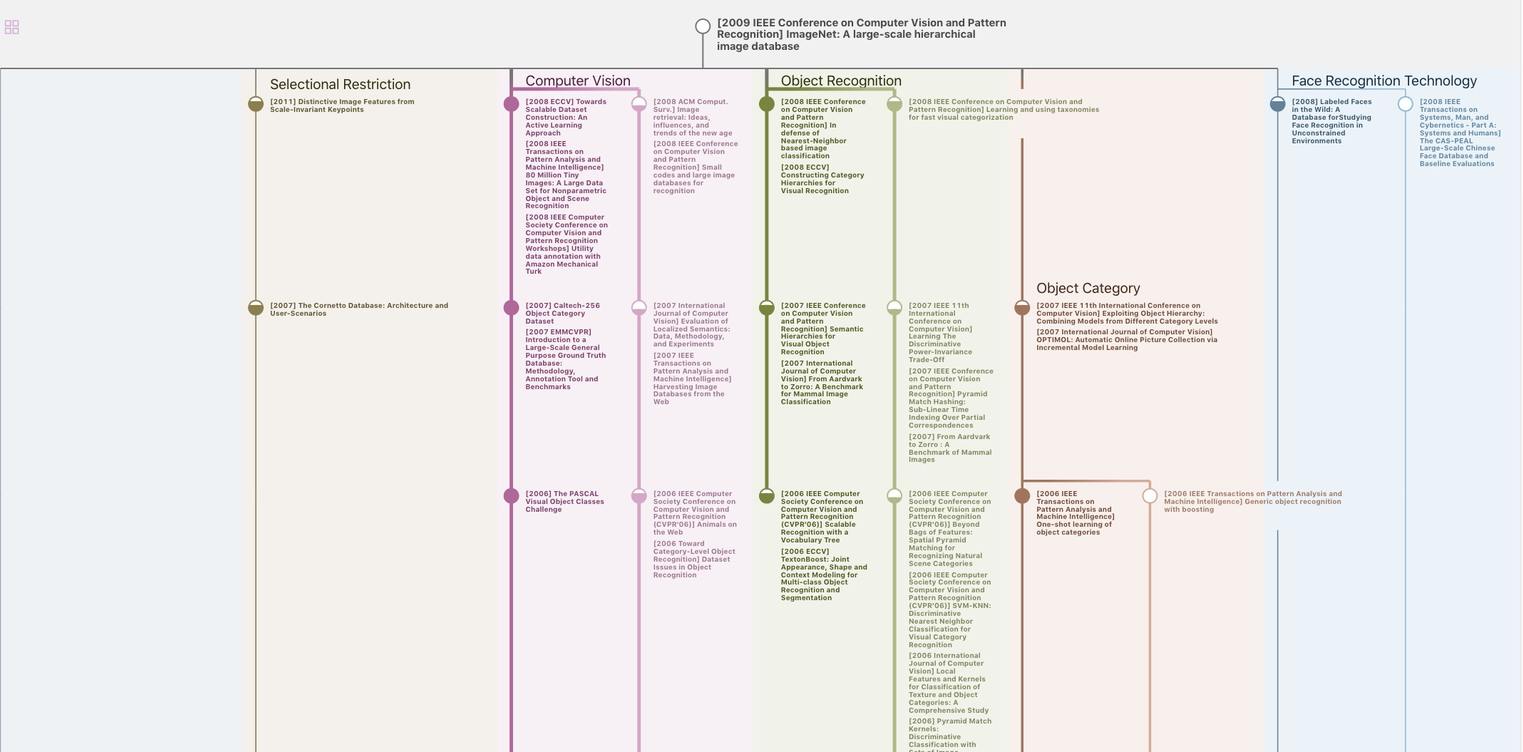
生成溯源树,研究论文发展脉络
Chat Paper
正在生成论文摘要