Integration of Reinforcement Learning into Fluid Control Systems.
INDIN(2023)
摘要
Reinforcement Learning (RL) is becoming increasingly important in closed-loop controller design. RL controllers adaptively adjust to time-variant and nonlinear system/ environmental characteristics and learn automatic controller parameterization without plant modeling. This paper investigates whether RL can be used as a control strategy for a pneumatic pressure control unit in time-pressure dosing (TPD) systems. These nonlinear systems are characterized by actuator hystereses and discrete-event driven changes in system dynamics depending on the system state. Further challenge in control arises from manipulating two actuators by one single control signal. We apply the Deep Deterministic Policy-Gradient (DDPG) agent and perform the training in loop with a first principles simulation model of the system dynamics in Simulink. We introduce a reward function to achieve the required steady-state accuracy and eliminate oscillating actuation. The trained RL controller is implemented on a 32-bit STM32F405 microcontroller by automatic code generation and is evaluated against an existing PI controller. The results show that the RL controller can control the pressure of TPD systems with the existing nonlinearities and discrete-event changes in system dynamics. Although the time constants of the real system differ from those of the simulation model, the RL controller still meets the requirements of the control loop. Compared to the PI controller, the RL controller improves the closed-loop dynamics by achieving lower time constants.
更多查看译文
关键词
Reinforcement learning control, DDPG, valve control, process control, MATLAB, Simulink
AI 理解论文
溯源树
样例
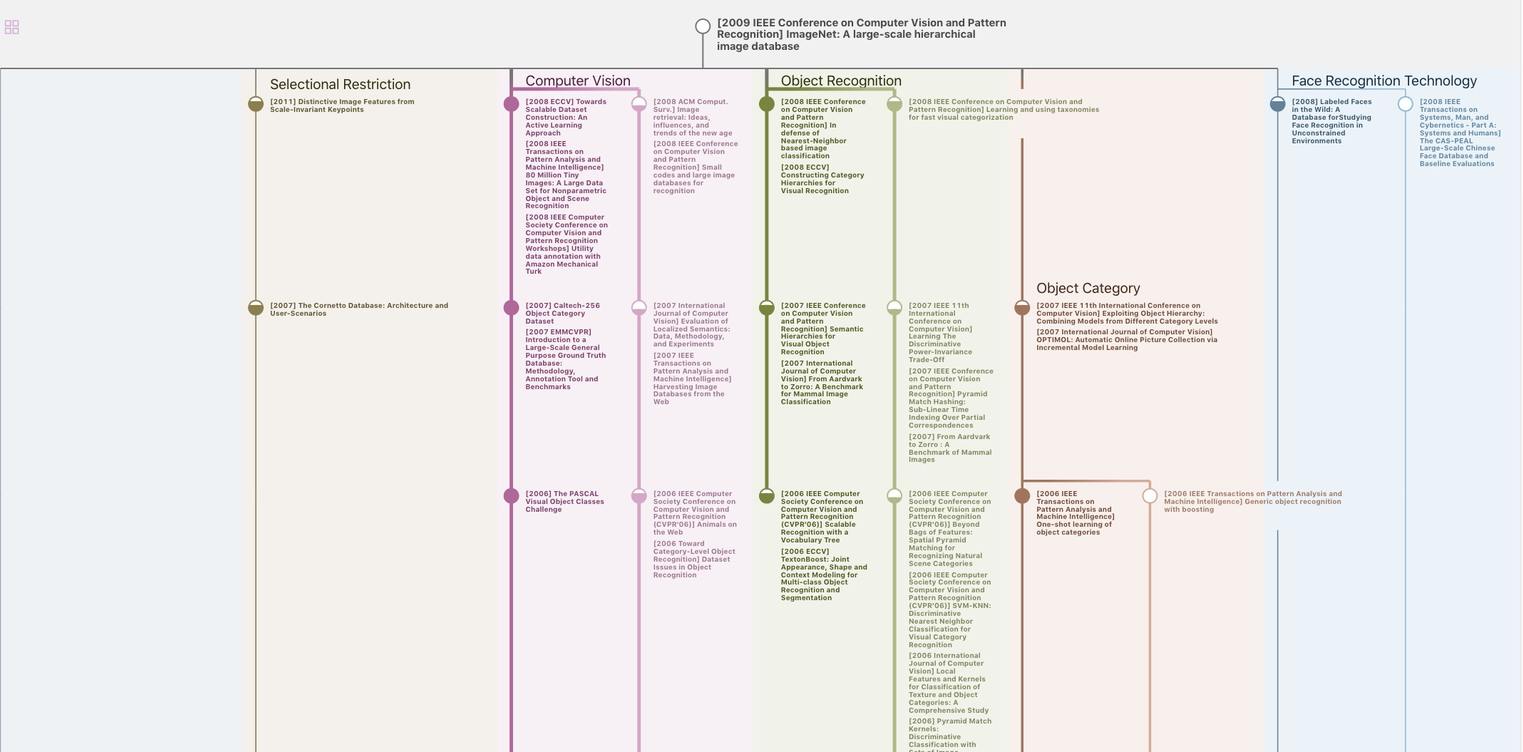
生成溯源树,研究论文发展脉络
Chat Paper
正在生成论文摘要